Red Hat welcomes the end of the storage monolith
In an open source originated commentary from Red Hat’s Irshad Raihan, director of product marketing, Computer Weekly Open Source Insider welcomes Raihan’s comments on the subject of computational storage… a topic that the Computer Weekly Developer Network has featured a series of stories on this summer 2021.
Raihan suggests to us that, in layman’s terms, it’s useful to consider the evolution in storage architecture as analogous to developments in camera technology.
Before the existence of smartphones, both amateur and professional photography required dedicated cameras that people took with them everywhere. However, smartphones have meant that most people today no longer need to carry around separate cameras – now, they have a tool that can take high-quality photographs which can also store and distribute them.
Raihan writes as follows…
Just as with cameras, so too with storage. The majority of modern applications meet the definition of ‘data-intensive’, but with ongoing improvements in storage affordability and density, most don’t need to be housed in a specified data-dedicated form factor.
This explains the factors driving the evolution of computational storage.
A promise of parallelism
Historically, computational storage architecture promises to integrate computing resources directly with storage, enabling parallel computing and lifting many existing constraints on I/O, memory and computing.
While offering across-the-board benefits, traditionally computational storage is particularly valuable for data-intensive applications where workloads need to be performed close to where data collection occurs, such as machine learning and edge computing.
To achieve these efficiencies, however, computational storage requires more complex architecture to meld compute and storage resources effectively. One particular bugbear for organisations is the need to overhaul their Application Programming Interface (API) regimen, in order to allow computational storage to both work and interface with existing workloads and processes. This represents a step-change in complexity – leading some to wonder if it’s worth it.
Going (cloud) native
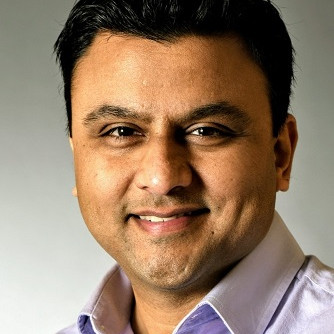
Red Hat’s Raihan” Computational storage is impacted by the [higher] ‘uber trend’ around infrastructure.
This shift has been driven by the need to make data accessible and portable from anywhere at low latency speeds – an effect of edge and hybrid cloud initiatives. This has seen those monoliths broken down into microservices that are enabled by containers – and underpinning this new development infrastructure sit APIs, which are responsible for communication and orchestration.
This shows, then, that the API complexity of computational storage has parallels in the industry, and that overcoming that hurdle is doable for many enterprises. Not only does this bring direct benefits through enabling computational storage, but rethinking how APIs interface with storage also brings a huge opportunity to break down the storage monolith.
For example, it presents the chance for organisations to implement a software layer of data services which can, for example, automatically store new data where it’s needed as it’s generated.
Reappraised relationship
By forcing us to reappraise how we treat the relationship storage has with compute, computational storage can compel us to reconsider how we treat storage in the first place. Due to how data was treated in the past, many developers regard storage as something necessarily monolithic that has to exist at scale, but this attitude doesn’t reflect either today’s technological reality or what may best suit organisations.
A more fine-grained approach is now possible and it promises to unlock huge benefits in tandem with the potential of computational storage. The ability for modern customer workloads to embrace data services, whether for data at rest, data in motion or data in action, has democratised access to more valuable data, in a more scalable, portable and accessible way.
Just as cameras and app developments have moved away from the restrictions of specialised equipment and infrastructures, so we can see an increased generalisation of enterprise infrastructure.
By the same token, infrastructure that is tied directly to specific workloads is becoming more specialised.
Computational storage is impacted by this uber trend around infrastructure. This shift, driven by the accessibility and portability needs of modern customer workloads, will likely encourage a revolution in how enterprises treat their data.