SLM Series - Zencoder: Compact models maybe small, but they are mighty
SLM Series – Zencoder: Compact models maybe small, but they ARe mighty
This is a guest post for the Computer Weekly Developer Network written by Andrew Filev in his capacity as CEO of Zencoder.
Zencoder is an AI coding agent platform designed to accelerate software development by automating tasks, enhancing code quality and improving developer productivity. It offers features like code generation, unit test creation, docstring generation, code repair and chat-based assistance, all powered by AI agents that understand a codebase.
Filey writes in full as follows…
Sometimes, less is more. In the world of AI, that idea manifests as Small Language Models (SLMs). While Large Language Models (LLMs) are grabbing headlines for their incredible prowess – generating everything from code to legal briefs – the more diminutive SLMs can be surprisingly nimble and effective in a host of scenarios. Let’s walk through some practical considerations around where each model shines and how to strategise their use (sometimes even in tandem).
When time is of the essence
Picture being a developer coding in your favourite editor. When you type a new line, you get real-time suggestions from our AI. That’s a magical experience – if it’s instant. For an LLM with billions of parameters, just generating the first token often takes a second.
Take a look at this chart:
By the time the larger models have something to say, you’re already two lines further in your work. In practice, for code-completion to feel seamless, we need the entire round trip – collecting context, sending it to the server or an on-device model, generating a response and rendering suggestions – to happen in approximately 500 milliseconds. That’s where SLMs truly excel. They’re smaller, so they can typically start generating tokens more quickly. Even then, you’ll want some engineering ingenuity (caching strategies, “predictive decoding”, etc.) to shave off more precious milliseconds. But the point stands: fast user-facing tasks are often best served by smaller models.
When the going gets tough…
Now, let’s look at the opposite extreme. You ask our AI agent to resolve a bug in your product. It needs to figure out what caused the bug across your 1,000 code files, quickly orient itself in the nuances of your architecture and propose a good solution. Even for a seasoned engineer on your team, who worked on this project for the last year, that kind of debugging might take an hour. And if you take an engineer from the street without prior knowledge of your project, it will probably take them a couple of days. Waiting five minutes for an AI’s “deep reasoning” is suddenly acceptable if it saves you 95% of that time.
For this scenario, bigger is often better, or as they say, “more is more”. A large, general-purpose model that has seen millions of lines of code in training can bring broad insight and produce robust solutions – even if it’s less snappy. Yes, you could ask an SLM to do it, but it might not have the “mental bandwidth” to hold all the context at once, or it might produce a more brittle solution. For big, open-ended tasks, an LLM is often the right call.
The magic of inference-time compute
Now that we have covered the two extremes, we can call it a day, or you join me on my journey into the rabbit hole in between, where things get curiouser and curiouser. This is the land of “inference time compute”:
- Sometimes, you can let the model run a little longer and “think about the problem deeper”. Techniques like “chain-of-thought prompting” or self-critique can dramatically enhance even moderate-size models. They have recently been taken to the next level by the models like OpenAI’s o1 and DeepSeek’s R1.
- Sometimes, by running a model multiple times and selecting the best result, you can achieve a better solution than running a bigger model once. That “multi-sampling” strategy often gives the required jump in intelligence, like going from o1 to o1-pro.
- Sometimes, you can evaluate the results of AI and ask it to correct its errors. This was one of the first things we built into our product to fix some of the problems traditional AI code-gen tools had.
In each of these scenarios, the raw speed of models matters because you’re effectively compounding multiple inferences. A bigger model might cost 10 times more – and take 10 times longer. If you multiply that overhead by repeated tries, it adds up quickly. That’s why “fast is sometimes better than perfect”- especially if you can rectify imperfections programmatically.
Training trade-offs
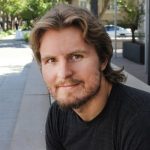
Filey: Big models are eye-wateringly expensive to train.
If you are still with me, let me take you further down the wonderland – into the area where fewer humans have stepped yet. Training. Big models are eye-wateringly expensive to train. For all but the largest labs (think Google, Meta, OpenAI, Anthropic), that’s a massive chunk of budget. And even those labs focus on cost optimisations, from innovations in model architecture to optimising data to specialised hardware.
Enter the SLM: Smaller models cost a fraction to train. That’s liberating because you can afford more experiments and more specialised tasks. Paying $5K for an experiment vs. $500K is game-changing for R&D cycles. This is partly why so many organisations now prefer to fine-tune a model with <100B parameters for domain-specific tasks – like analysing legal contracts or triaging medical images – rather than trying to wrangle a large, general-purpose model with endless hyperparameters.
However, training remains overrated for many businesses. The dizzying speed at which new base models appear means you might be better served by a well-crafted prompt engineering approach. Instead of spending months and millions on training, you might get most of the value from optimising instructions, examples and context that you give to LLMs. And if that doesn’t get you to the destination, the inference time compute and RAG techniques can sometimes be more advanced and powerful than training.
Balancing speed & power
Looking at the broader landscape, we can draw several important conclusions about the roles of different AI models. Small Language Models offer exceptional speed and cost-effectiveness, making them ideal for real-time applications and edge deployments where immediate response is crucial. On the other hand, Large Language Models, while slower and more resource-intensive, provide the broad knowledge and deep reasoning capabilities needed for complex analytical tasks. Between these two extremes, inference-time techniques are emerging as a powerful middle ground, particularly with mid-size models around 70B parameters, offering a balance between speed and capability.
In essence, both SLMs and LLMs are indispensable in modern AI. When you align the right tool (or combination of tools) with the right job, you’ll see vastly improved efficiency, accuracy and – most importantly – results your customers and teams will love.