Google Cloud VP: Beyond monolithic AI into heterogeneous harmony
This is a guest post for the Computer Weekly Developer Network written by Peter Bailis in his position as VP of engineering at Google Cloud.
Bailis’ full original title for this piece is — Beyond Monolithic AI: Heterogeneous Model Architectures for Enterprise Applications.
Reminding us that while Large Language Models (LLMs) have captured headlines, Bailis says that the practical application of AI within the enterprise often necessitates a more nuanced approach.
Small Language Models (SLMs), far from being mere downscaled versions of their larger counterparts, represent a distinct and valuable category of models optimised for specific enterprise needs, so what does the Google Cloud VP think are the main drivers for this space?
Bailis writes in full as follows…
SLMs are frequently designed with distinct architectures, trained on specialised datasets and optimised for objectives beyond pure capability – such as latency, memory footprint and/or power efficiency.
This targeted design often yields substantial advantages in efficiency, speed and cost-effectiveness, making SLMs a pragmatic choice for many tasks, either independently or as part of a larger system.
Engineering, fundamentally
The selection of an appropriate model size is fundamentally an engineering trade-off. For complex tasks requiring sophisticated reasoning, integration with multiple tools, or processing diverse data types, a larger parameter foundation might be necessary – think of multi-step processes that demand nuanced understanding.
Conversely, for “simpler”, more defined tasks like sentiment analysis within a batch offline analytics pipeline, a much smaller, highly optimised SLM can deliver excellent results with significantly lower resource consumption.
The key lies in matching model capability to task complexity, desired accuracy and budget constraints.
Hybrid homogeneity
Hybrid systems combining LLMs and smaller models can offer effective solutions. LLMs can provide broad knowledge and handle complex, open-ended queries, while specialised smaller models excel at specific, high-volume, or latency-sensitive tasks. Imagine a system where an SLM handles the bulk of routine customer service inquiries quickly and cheaply, escalating only the truly complex or novel cases to a more powerful LLM.
This “cascade” approach leverages the best of both worlds.
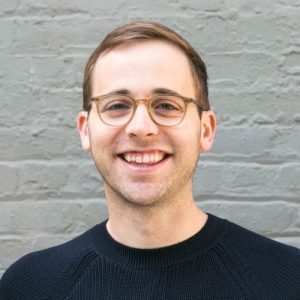
Bailis: The key (to model choice) lies in matching model capability to task complexity, desired accuracy and budget constraints.
Implementing such multi-model systems necessitates intelligent routing mechanisms. Think of a model router as an AI traffic controller. It analyses incoming queries and dynamically directs them to the most appropriate model – whether it’s a specialised SLM or a generalist LLM. This ensures optimal resource utilisation, minimises latency and maximises the accuracy of the response.
Routing logic can range from simple rule-based systems to learned models.
Current techniques include:
- Query Classification: A lightweight model predicts the type of task or required capability, directing the query accordingly (e.g., sentiment analysis vs. summarisation vs. code generation).
- Decomposition: A coordinating model (potentially an LLM itself) breaks a complex request into sub-tasks, dispatching each to appropriate specialised models and potentially synthesising the results.
- Capability-Based Routing: Routing based on explicit model capabilities (e.g., routing requests involving images to a multimodal model, time-series data to a forecasting model).
For AI data engineering, SLMs offer compelling advantages stemming from their efficiency.
Their smaller size enables faster training and fine-tuning, allowing data engineers to build, tests and adapt models much more quickly and with less computational burden, streamlining development workflows. Furthermore, the operational benefits are significant for data pipelines: lower inference costs make it feasible to integrate AI models into large-scale batch processing tasks, while reduced latency is critical for optimising real-time data streams and enabling timely actions based on model outputs.
In conclusion, while LLMs continue to advance the frontiers of general AI capabilities, the development of practical, efficient and cost-effective enterprise AI systems increasingly relies on the strategic use of SLMs.
Where AI models go next
We can say that the advantages of SLMs lie in training speed, inference efficiency and adaptability… all of which make them valuable components, often used in concert with larger models within intelligently routed, multi-model architectures.
This reflects a move towards heterogeneous systems tailored to specific operational constraints and task requirements, rather than relying solely on single, monolithic models.