Computational storage series: Model9 - The cloud + computational storage sweet spot
Software runs on data and data is often regarded as the new oil. So it makes sense to put data as close to where it is being processed as possible, in order to reduce latency for performance-hungry processing tasks.
Some architectures call for big chunks of memory-like storage located near the compute function, while, conversely, in some cases, it makes more sense to move the compute nearer to the bulk storage.
In this series of articles we explore the architectural decisions driving modern data processing… and, specifically, we look at computational storage
The Storage Network Industry Association (SNIA) defines computational storage as follows:
“Computational storage is defined as architectures that provide Computational Storage Functions (CSF) coupled to storage, offloading host processing or reducing data movement. These architectures enable improvements in application performance and/or infrastructure efficiency through the integration of compute resources (outside of the traditional compute & memory architecture) either directly with storage or between the host and the storage. The goal of these architectures is to enable parallel computation and/or to alleviate constraints on existing compute, memory, storage and I/O.”
This post is written by Gil Peleg in his capacity as CEO & founder of Model9 — the company provides cloud data management for mainframe, specialising in delivering mainframe data directly to private or public cloud for backup, archive, disaster recovery and space management, as well as integration with BI and analytics tools.
Peleg writes as follows…
The SNIA’s concept of computational storage and Computational Storage Functions (CSF) represents an important acknowledgment of the growth of data and its increasing importance to the enterprise.
While the focus of CSF seems to be mostly on edge applications (reducing and preprocessing large volumes of data) and on strengthening on-premises storage handling, similar motivations are also impelling data movement and data sharing with the cloud.
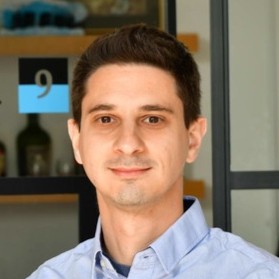
Model9’s Peleg: Computational Storage, Sometimes – Cloud, Always.
Particularly in the case of mainframe environments, bottlenecks and limitations abound in legacy infrastructure. Copying data to the cloud – or simply relocating it there – allows for cost-effective retention and analysis using, in many cases, parallel processing and achieving a revolution in value from data that is currently trapped and siloed. Cloud offers flexibility and unlimited processing capability when needed.
The cloud computational storage sweet spot
The use of computational storage to reduce latency for performance-hungry processing tasks is not necessarily a matter of adding specialised processors or radically transforming architectures. For many tasks and many organisations, particularly legacy mainframe with its chronic siloing of data in tape and VTL storage, the answer is making data available to the cloud and processing in the cloud… but if organisations can combine and dovetail an element of their new cloud estate with the intelligent implementation of computational storage, then things start to look sweet.
Few enterprises tap large data sets continuously. Rather, data scientists seek productive ways to analyse data and then repeat the process at useful intervals. This is something that can be done cost effectively with commercial off-the-shelf technology – namely cloud, yes I am advocating cloud again, but it needs to cloud + computational storage where the use case justifies and validates its implementation.
Moving beyond legacy mainframe
Legacy mainframe is no longer the best compute platform for big data analytics. Its storage is expensive and typically not performant enough for these use cases. Tape and virtual tape, in particular, are not up to modern demands. Computational storage can play an important role in the new architectural make-up that organisations could be looking to build in the smarter storage plus smarter cloud era, it’s a question of knowing which key data sets need to be exposed to which compute and storage resources when and where.
The bulk movement of data and conversion of data to standard formats used in the cloud is no longer prohibitive or especially challenging. This should further help businesses adopt computational storage in deployment scenarios where it makes sense.
This approach obviates the high-risk and potentially high-cost approach of engineering and implementing a new generation of ‘smart’ storage devices on their own – though such technology may be useful or necessary in applications such as edge/IoT.