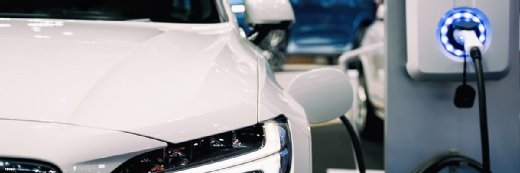
ake1150 - stock.adobe.com
CW Innovation Awards: Finding revenue gains from parked cars
Singapore real estate investment firm CapitaLand Investment taps machine learning models to optimise space utilisation across 23 commercial buildings and business parks
When CapitaLand Investment embarked on an initiative to develop a carpark prediction system, it set out with the goal to generate new revenue while minimising its environmental impact.
The Singapore-headquartered real estate investment firm tasked its data and artificial intelligence (AI) team with the project, which was developed in-house and primarily on Python. Completed over three months, the system was rolled out across the carparks of 23 CapitaLand business parks and commercial buildings.
Founded in 2000, CapitaLand Investment has 2,878 employees in Singapore, of whom 12 are in IT. The company aimed to enrich user satisfaction and deliver a seamless parking experience, by being able to dynamically release season parking lots to non-season carpark users when demand for the former was low.
It was keen to drive revenue with the ability to more accurately forecast usage patterns and optimise land space. It also hoped to reduce the need for additional infrastructure by optimising existing resources, hence minimising its environmental impact.
The project could further set new industry benchmarks should the prediction system prove to be scalable and adaptable. It could also inspire new innovation in urban planning.
The carpark system leverages several machine learning algorithms, including logistic regression, lasso regression, random forest, CatBoost and light gradient boosting machine (LightGBM).
These algorithms, which were used to train various machine learning models, were picked for their ability to handle large datasets and labelled data as well as predict specific outcomes, such as carpark occupancy rates and availability.
To ensure adjustments can be made dynamically based on recent data patterns, all machine learning models are run weekly to generate predictions for the following week. The best performing model is then selected each week and used to allocate parking spaces for the upcoming week.
Improving user experience
CapitaLand believes this approach will allow the accuracy and efficiency of the system to be continuously improved.
The prediction system also incorporates seasonal trends and events-based fluctuations, which enables the release of season parking lots to non-season users to ease demand during peak periods. This capability ensures space utilisation is optimised while user experience is enhanced.
Efforts also were made to engage stakeholders during the implementation, with regular discussions carried out to address concerns and provide updates on the project’s progress. Pilot trials were conducted at selected car parks, so potential issues could be resolved and operations were assured to run smoothly, before the system was deployed at full scale. In addition, CapitaLand gathered feedback from users so the necessary finetuning could be made to the system based on their experience.
With its ability to forecast usage patterns and optimise space, the carpark prediction system has generated a 15% increase in overall revenue for CapitaLand. It has also shown potential for future expansion into more commercial buildings, with the possibility of integrating more advanced AI techniques and additional data sources.
Through the project, CapitaLand has learnt that deploying scalable systems and AI models is key to success and the ability to handle large datasets crucial. The underlying infrastructure must also be able to expand to support multiple locations without any compromise to performance.
In addition, the prediction system must adapt to real-time data and evolving usage patterns. Here, CapitaLand believes its use of diverse machine learning models has proven to be critical, with each model offering unique strengths and abilities to carry out different prediction tasks effectively.
Its lodging business unit, Ascott, also leverages various real-time data to power a chatbot and help users plan their travels, including Bing search, Azure Map’s “nearby” and weather application programming interfaces (APIs), as well as Ascott’s DiscoverASR.com. Running on generative AI, the chatbot can provide various travel insights, including destination highlights, accommodation recommendations, attractions and “Instagram-worthy” spots.
Read more about CW Innovation Awards 2025
- Facing rising cyber threats and a shortage of experts, Citic Telecom International CPC developed an AI-powered penetration testing tool to automate security audits and reduce costs.
- M1 is running all its backend systems on the cloud, enabling it to speed up decision making and enhance customer service.
- A company-wide SAP revamp has transformed Bosch Global Software Technologies’ financial and HR processes, delivering granular insights and streamlined operations.
- DBS Bank’s generative AI tool is streamlining customer service, reducing manual work and speeding up response times, improving both employee and customer experience.