The low-no-code series - Databricks: Ethical coding, behind the science of bias
The Computer Weekly Developer Network gets high-brow on low-code and no-code (LC/NC) technologies in an analysis series designed to uncover some of the nuances and particularities of this approach to software application development.
Looking at the core mechanics of the applications, suites, platforms and services in this space, we seek to understand not just how apps are being built this way, but also… what shape, form, function and status these apps exist as… and what the implications are for enterprise software built this way, once it exists in live production environments.
This piece is written by Lexy Kassan is her role as data and AI strategist at Databricks – a company known for its data warehouses & data lakes ‘lakehouse architecture’ technologies and platform designed to allow users to collaborate on all data, analytics & AI workloads.
Kassan writes as follows…
To address the imbalance between the demand for software development and the shortage of skilled developers, organisations are increasingly turning to low-code/no-code platforms. In fact, [estimates suggest that somewhere] around 70% of new applications will be developed by companies using low-code/no-code technologies by 2025.
The value of this new technology is considerable. Now anyone, with just a very basic understanding of the capability of Machine Learning (ML), can train advanced models on their datasets.
These individuals are commonly referred to as ‘citizen data scientists’ and by empowering them to experiment with various features and models before reaching out to the data science team to help, business can unleash hidden talent, innovate and grow.
But with all this rapid growth and change, are businesses monitoring for ethics and compliance?
It would be a mistake, when asking this question, to lump compliance and ethics together. Staying compliant is fairly straightforward, with new data processes requiring compliance audits – essentially ensuring legality. But ethics is a very different story. The reason for this is that when discussing whether something is ethical or not, the outcome will largely depend on the context. Ethics should be thought of as the end result and legal as the baseline. With legal requirements so often trailing behind fast-paced technological advancements, this is not always so simple.
The big ethical dilemma
One of the major challenges of remaining ‘ethical’ is the lack of any universally accepted or agreed-upon code of ethics when it comes to data technologies. This is despite many calls and attempts for them to be created. The Royal Statistical Society (RSS), for instance, published a ‘Guide for Ethical Data Science’ in 2019.
As a testament to how quickly the data space moves, low-code/no-code was not mentioned. So herein lies the problem – even if a universally accepted code of ethics did exist, the world of technology is ever-changing. Regulation is always playing catch-up, always in need of updating.
Yet, this doesn’t mean companies and individuals alike shouldn’t strive for ethical practice. Companies must have their own code of ethics in place and must ensure that their employees are educated on why it’s there and how it’s used. Having this in place will not only protect an organisation’s customers, but also save an organisation from legal issues or reputational damage.
Lies, damn lies… and statistics
Importantly, data ethics is not just for those responsible for implementing code or developing an ML algorithm. It is something that needs to be widely adopted, especially with the rise of low-code/no-code tools.
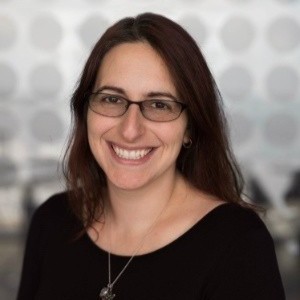
Databricks’ Kassan: The science of bias is real – the responsibility lies with all of us.
Understanding data ethics could help raise awareness around areas where data use has the potential to produce unethical results. For instance, the number of hours worked over a week might be used to measure productivity. However, this could possibly create bias against working parents, for example.
Importantly, companies will have to decide if a problem is one for the citizen data scientists using low code / no code, or whether the process is deemed too sensitive. Restrictions need to be in place to mitigate risky use of data or development of models that could produce these type of inaccurate or discriminatory results.
The big ethical automation question
Ethics is especially important when it comes to automating processes. Amid the widespread shortage of tech workers, automation tools are now seen as one long-term solution to address the lack of available tech talent in the market.
While there is a lot of value to be gained from this, automation is not without its challenges. For instance, when it comes to workforce allocation, automating scheduling removes the task of organising calendars from managers. However, an unintended outcome could be that shift workers are systematically removed from future schedules due to a lack of data or a perception that their shifts had been less productive. This means that instead of helpful interventions, like offering training or more support, employees could simply be removed from a team.
The onus is not on data scientists alone.
Organisations, as a whole, have a responsibility to analyse what processes could be biased to prevent complications and unintended consequences. Clear guidance and transparent communication from a corporate standpoint must go hand in hand with use of low-code/no-code platforms. With this approach, organisations can fully reap the benefits that low code / no code has to offer – enabling greater speed, flexibility, and innovation.