Progress VP: how to shine a light on dark data
There’s a lot of data out there and it comes in various different forms.
TechTarget defines dark data is digital information that is not being used.
Magical analyst Gartner Inc. describes dark data as, “Information assets that an organisation collects, processes and stores in the course of its regular business activity, but generally fails to use for other purposes.”
So… given that developers will typically build applications that ‘gorge’ on as much productive data as possible, should we care about the dark factor and try to shine a light into the shadows?
VP for product, cognitive first, at data platform company Progress is Ruban Phukan — and Phukan says yes, we need light.
He bemoans the fact that dark data continues to be a challenge for businesses, especially in spaces like Industrial IoT (IIoT).
Phukan lists three reasons (at both the machine and the organisational level) why this might be the case:
- Machines might be fit for data generation but the relevant sensors have not been set up to capture insights.
- The data generation capability of machines might be utilised but the data generated ends up discarded.
- On the organisational level, the business might not have the storage capability to redeem and process data.
We should also consider the situation where the organisation is able to collect and store data but, due to a lack of data analytics skills, it is simply unable to analyse it.
“These above scenarios mean that there are still data silos that remain untapped. To address these challenges, organisations need to ensure they have an end-to-end data generation, storing and analytics strategy in place that will allow them to reap the full potential of data,” said Phukan.
Steering us towards what he contents is a viable answer. Progress’ Phukan lists the four stages of end-to-end data management.
- Data generation: Identify all data generation points and ensure they are enabled. This requires good knowledge at the machine level
- Data collection: Put a data collection strategy in place. This will allow the regular and timely collection of the data generated
- Data storage: Data should then be stored in a data repository on the cloud or data centre so that it is not only safe but accessible.
- Data analysis: This is the stage where organisations need to decide how they will ‘productionise data science’ to leverage value from data and integrate into business processes’
Data layers
Progress explains that these ‘layers’ of data break out as follows: the front-end is the engagement layer with content, context and the interaction with the user; the back-end of our apps is made up of the infrastructural elements including application behaviour policies, business rules and business logic — and central layer is the data science zone where the analytics happens.
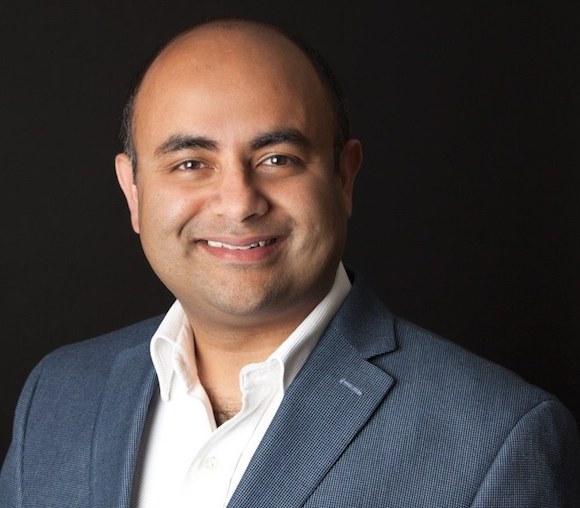
Ruban Phukan is the co-founder and chief product & analytics officer at DataRPM (acquired by Progress) where he leads product and the data science for the flagship Cognitive Predictive Maintenance product.