Oxford Immune Algorithmics founder: Defining the split between human brains & AI
In resonance, response and respect of the Computer Weekly Developer Network’s recent and ongoing coverage of Large Language Models (LLMs) and the related work associated with building generative Artificial Intelligence (AI) applications and indeed Artificial General Intelligence (AI)… we felt we needed to hear more.
So then, this is a guest post written in full by Dr. Hector Zenil, founder of Oxford Immune Algorithmics (OIA), a specialist organisation focused on data science and computational intelligence.
Zenil writes as follows…
It is amazing how far and fast AI innovation has evolved in the last five years, particularly prompted by the arrival of generative AI. However, rather than get carried away with the hype, it is crucial to understand what the different models can achieve and which ones are best suited to a particular task. Even two years ago, machine learning and then deep learning where seen as the breakthrough, but now all the talk is about generative AI and large language models (LLMs). Why is that so?
One way to think of written and oral natural language is as the operating system of the human mind. Everything we do, even most of our self-deliberating internal voices, are based on natural language. We speak to ourselves in whatever native language we were raised in or learned, from English, to Spanish, to Chinese and Hindi, we all generally interact with each other in some of these languages. It is almost like our machine code and interface.
LLMs are the AI approach to cracking the human’s operating system and it somehow feels like it has been broken. We feel exposed, like emperors with no clothes.
We suddenly find ourselves excited about technology while also fragile, violated and somehow surprised that cracking language was neither too difficult or very special. It is still early days as it does not yet feel like human intelligence has been completely broken into, but to some good extent, LLMs have taken significant steps forward in understanding language. This apparent disconnection between language and intelligence also feels uneasy, as we have always operated under the assumption that language is indicative of intelligence, from the way we praise political orators, influencers and communicators, more than say scientists in general, but cracking how natural language works is not the same as understanding natural intelligence.
Limitations of generalist LLMs
While the hype suggests AI will surpass human intelligence in no time, the reality is that it is not even entirely clear whether generalist LLMs, that is LLMs trained on non-specialised content only across all domains without any selection process, are more effective or can outperform LLMs that are trained on smaller and more specialised datasets even on questions of that particular domain. This is important when it comes to deciding which tasks may be undertaken by AI and how deep LLMs can go into human wisdom and human reasoning capabilities. Can LLMs really generate deep high-level content that requires sophisticated reasoning, or they are only regurgitating online content back to us?
A generalist LLM could be applied to a basic chatbot in an online consumer retail setting to respond to a variety of tasks and give the impression of a near-human dialogue; and if there are errors, they may cause frustration but not cause the system to fail. However, in the field of healthcare where Oxford Immune Algorithmics operates there is a huge variety of dependencies to consider when seeking to understand how the human body reacts to a particular treatment. This necessitates more specialised AI and is also dependent on how much data is included in the general training set. More importantly, though, we require LLMs to either think like human scientists or in ways that outperform their cognitive inference capabilities.
For example, a generalist LLM such as GPT 4 in specialised domains like maths and medicine has found it difficult to outperform smaller but specialised LLMs trained on domain-specific databases.
The most likely explanation for this phenomenon is that LLMs are still highly statistical objects that reply by averaging over all possible answers as a function of answer-space size. Specialised text is likely to accumulate in the extremes of long-tail distributions difficult to access. Paradoxically, if the distribution is large enough and the long tails are also fat, content in fat long tails is easier to access in very large generalist LLMs than in smaller specialised ones. This is why the phenomenon of model collapse also exists. An LLM is a giant averaging system, which if fed from averaged text, will end up with a very high mean that acts as a global attractor from which the LLM won’t be able to produce the variety needed to deliver any more original content.
In simpler terms this means there is a limit to the level of creativity and originality that generalist LLMs can produce and in specialised LLMs, those tails are less fat than in its generalist counterparts by definition.
A human mind’s higher-order mechanism
In contrast, human minds seem to be able to switch between generalist and specialised modes to speak one minute to children in an unsophisticated manner and the next one to a highly trained scientist colleague at a conference. While there is some prompting based on contextual framing and interlocutor queuing, the transition does not seem to be smooth but like a switch. The human mind seems to have a higher-order mechanism that allows it to plan, anticipate, simulate and move across different modes that LLMs currently do not. So, this has led to some consensus among scientists and technologists that current systems may require additional levels of abstraction with a higher-level hierarchy acting as a control mechanism.
There is still more to discuss so let’s move on to the rise of neuro-symbolic & hybrid AI.
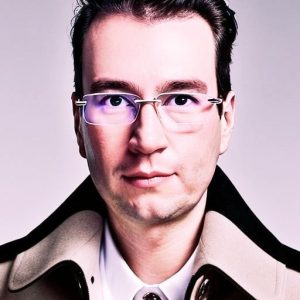
Dr. Hector Zenil, founder of Oxford Immune Algorithmics (OIA).
This behaviour or lack of behaviour not found across current LLMs will be a major discussion point when considering the future of gen AI and AI. New AI systems are likely to keep incorporating other components in the form of architecture choices, such as symbolic ones. A calculator, for example, is a quintessential example of a purely symbolic system that does not learn over time but knows how to deal with well-defined symbols. These systems are able to switch between modes or states displaying completely different behaviours determined by an internal rule directly or indirectly encoded in its rule table.
The advantage of symbolic systems is that they are able to answer with infinite accuracy to never-before-seen cases and why hallucinations are considered bugs in these systems. You do not need to train a calculator over an ever-larger arithmetic data set in order to deal with ever larger arithmetic calculations. By contrast, LLMs need to have seen each specific arithmetic example to get the symbolic computation right, hardly inferring any symbolic rule from its statistical patterns (or taking too long) and it is therefore an impossible task for current LLMs to ever learn arithmetic just as we pretend to do with our human minds.
This is why LLMs got things like arms and fingers wrong, because they never learned to count.
They would draw a finger and ask themselves how likely it was to have another finger next to it in that particular position. In more cases than it should it would then add another finger or even another arm, because it was not able to generalise or deduce that humans have five fingers and two arms no matter how many examples it would see.
Solutions to this kind of challenge have come by human intervention in the form of rule-system tweaks to force LLMs to draw hands and arms in a better way but that has been difficult to enforce – even when we assume that LLMs have cracked language they have never understood the concept of a finger or five. Human minds are somewhere in the middle of these two worlds. We do not learn as calculators do but we are good at abstracting, simulating (imagination and planning of future states) and mediocre at inferring while we can force our neural minds to learn rules from statistical patterns. The human mind can abstract multiple models in order to perform model selection. In addition, the human mind can simulate multiple likely models to compare them to the expected outcome. Let’s say we want to get a job promotion; we produce several mental models in which we see ourselves achieving the goal and we then act. This is what has been identified as ‘planning’ and the elements needed for planning seems to be lacking in current deep learning AI approaches, including foundation models, Gen AI and LLMs.
Therefore, the future will be a combination of these worlds at different levels, with architectural choices, training strategies and different levels of operation that will make AI more relevant in critical areas such as science, healthcare and medicine.
So far, AI has had limited impact in these fields as it is unable to rewrite physics or chemistry textbooks beyond successful classification applications even if often impressive such as those from AlphaFold that resolves large cases of protein folding. This approach has the potential to achieve Artificial General Intelligence, or AGI, as conceived as capable of performing like or beyond human capability in both generalist and specialised situations.