LLM series - Workday: How LLM ecosystems can prevent model collapse
This is a guest post for the Computer Weekly Developer Network written by Clare Hickie in her role as EMEA CTO at Workday.
Hickie says that ‘model collapse’ is a very real issue that must be addressed before any action becomes remedial.
Detailing the mechanics of this scenario, Hickie writes as follows in full….
What is model collapse?
It is what occurs when large language models (LLMs) and other AI systems aren’t trained on human-generated data, but instead on data generated by other AI models, intentionally or not. As the internet becomes flooded with AI-generated content, there’s a chance that new AI systems will increasingly become trained on that content. AI systems can malfunction when that happens, drastically reducing the quality of the model’s output, to the point where it becomes unusable.
The rate of AI development is a hockey-stick exponential curve. It is rapid. But we shouldn’t lose sight of what we’re really trying to achieve with AI – improve human lives. We won’t achieve this goal if we’re not intentional and responsible about how models are trained.
The dangers of model collapse
An AI model trained on the output of other such models collapses in three to four rounds on average. If a model is trained on data provided by a model that was trained on data provided by another model, the outcome is useless output.
It is predicted that by 2026, 95% of all content on the internet will be AI-generated. That in itself is an interesting phenomenon but if this future development isn’t factored into the ethical development of AI systems, new models won’t really be fit for purpose in solving the problems that matter.
Furthermore, there’s currently no real way machines can accurately distinguish between machine-generated output and the human equivalent.
Widespread model collapse is therefore a great concern to AI’s future development and the opportunity AI brings. However, it’s important to note that not all models will be affected equally. The worst affected will be generic LLMs that rely on the open Internet for training data. AI systems developed for a specific purpose, or disciplines by private vendors, will not be impacted as heavily. Generally, custom AI systems will be trained on internal, human-generated data resulting in good quality output as intended.
So how can we ensure that as many AI systems as possible do what they’re supposed to?
A unified, ethical approach
To mitigate potential disaster, enterprises need to use responsibly trained AI systems. Responsible and trustworthy AI systems can positively impact society and amplify human potential. These systems exhibit characteristics like validity and reliability, transparency and interpretability, fairness, privacy enhancement, and security and resilience.
Such models can only operate at this level if they’re trained by better yet human input at a community-wide level, not by machines. Ultimately, the enterprises that succeed going ahead will be the ones that produce relevant, human-informed data and use their data storehouses to train models and leverage the technology effectively.
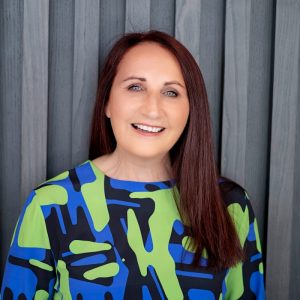
Clare Hickie, Workday, EMEA CTO.
As with most technologies that develop at pace, the only way to ensure that such technologies benefit humans is to develop them with collaboration at the center. As such, while there exists no real way to prevent model collapse at the moment, steps can be taken to mitigate the impact that would otherwise be felt.
Organisations involved in LLM creation should share information and data with one another, and make conscious efforts to determine the origins of both to train the models they build.
At the same time, enterprises should rely increasingly on bespoke AI models developed for a specific purpose as such tools can’t be corrupted by AI-generated data.
Ultimately, the entire ecosystem must be aware of the very real dangers presented by model collapse and follow best practices to mitigate them to make AI the life-changing tool it’s poised to become.
Only through responsible, human collaboration can we ensure AI does what it’s supposed to.