Claranet cloud lead: Sharper developer tools needed for edge compute
We all know where the edge is, it’s that peripheral zone of data existence somewhere near the outer reaches of the western spiral arm of the galaxy in the Internet of Things (IoT).
Given that we are now empowering our IoT devices with an increasingly sophisticated set of data storage, compute, analytics, interconnectivity and integration prowess, does the hardware-software section of the software industry need to step up and provide greater toolset power.
Proponents of so-called Computational Storage Disks (CDS) and the functions it can deliver would argue yes. Indeed, the Computer Weekly Developer Network ran a series of analysis pieces on this arguably increasingly important sub-genre of technology earlier this 2021.
In a follow up to those discussions which can be found here, this is a guest post examining related issues written by Liam Bennett in his role as cloud practice director at Claranet — a company known for its work in modernising and running critical applications and infrastructure through end-to-end professional services, managed services and training.
Bennett reminds us that edge computing and the process of bringing computation and data storage closer to the location where it is needed, is transforming the power and sophistication of everyday technology.
He says that Computational Storage (CS) is taking this to the next level and notes that new modern solid-state drives (SSDs) are being designed with compute-capacity built-in, meaning processing is done on the spot, with minimal to no throttling or latency issues.
Bennett writes as follows…
Computational Storage holds the solution to some of the limitations of edge computing. Rather than information being sent to the cloud or another appliance for processing, all this work can be done at the storage device level, which brings a host of benefits.
CS allows technology to work at lightning speed, save on bandwidth and not compromise on any quality. It also helps to decrease the risk of cyber-security attacks as it enables tougher and more extensive encryption to take place within the device itself. As data has no cause to leave the device, it is better protected from information leaks.
It also enables tougher and more extensive encryption to take place within the device itself, known as ‘self-encrypting drives’ or SEDs. As data has no cause to leave the device, it is better protected from information leaks.
Green green grass of cloud
This is the way we can expect things to be going forward. Hyperscale cloud providers such as Amazon Web Services and Microsoft Azure have started to provide this level of secure compute within their cloud services. For example, AWS IoT Greengrass, an open source edge runtime and cloud service that helps users to build, deploy, and manage device software and Microsoft Azure’s IoT Edge, which allows cloud intelligence to be deployed locally on IoT edge devices.
By providing this integration and buying into the hardware themselves, the hyperscale cloud providers are helping to drive down the costs of this technology, making it more accessible for everyone within the enterprise.
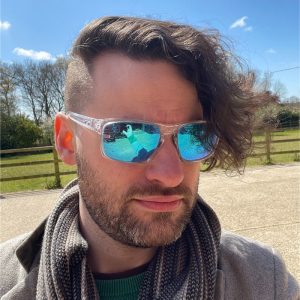
Claranet’s Bennett: The edge is edgy, but it needs (security & encryption) depth too.
For our customers, it allows us to provide more services and value. We are able to speak with our customers about edge-computing and IoT and have deeper and more meaningful conversations about security and encryption too. It helps to give us a much broader range of depth of services to offer and talk about.
A smarter ML edge
Additionally, CS is also helping to support a lot more analytics and machine learning happening at the edge. Being able to have that compute both within appliances and on the storage enables machine-learning models to be pulled down from the cloud – where they are trained and generated – to the source of the data. This opens up the door for edge analytics, helping users to make more instantaneous decisions based on data that is being streamed through.
The hyperscale cloud providers are using this within their database offerings to provide increased performance on analytical workloads.
Increasing demand for real-time data analytics will drive edge technology forward over the coming years. Most companies are now moving beyond these big data warehouses which are crunching numbers and making decisions hourly, daily and weekly, to streaming analytics where they are making these decisions dynamically.
To achieve this, machine learning needs to be able to work as close to the problem as possible – right on the edge.
I often use the example of predictive maintenance in manufacturing. You have companies investing millions into the production of equipment, from a digger used in construction to a fridge that sits in in a home. Manufacturers want to be able to analyse and detect the performance and failure of this equipment in order for them to be pro-active in their maintenance services but also drive better quality into their production systems.
As a result, equipment is now being produced that is able to track, store and send data back to the manufacturer about these devices. Computational Storage is the next extension of this, as it allows on-device processing of machine-learning models to react to the data produced on these devices rather than having to stream the data into the cloud.
In these manufacturing scenarios where safety is the biggest concern, having the compute directly embedded into the storage devices enables the greatest performance and therefore a much greater reaction time to failure events.