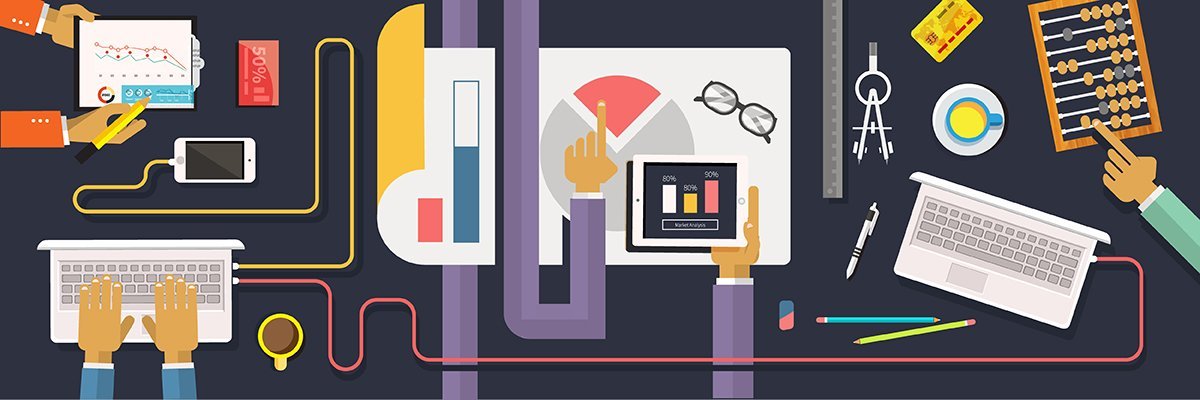
Data Matters
Debate and discussion around data management, analytics, BI and information governance.
Recent Posts
-
Knowledge Management 2.0: have we found a way to make KM work?
- Senior Analyst, Business Applications 23 Jan 2024 -
Unit4 predictions for 2024: pervasive ERP, conversational UX breakthroughs
- Senior Analyst, Business Applications 04 Jan 2024 -
Predicted tech trends driving the world of work in 2024
- Senior Analyst, Business Applications 20 Dec 2023
This is a blogpost by Jim Webber, Chief Scientist at Neo4j, on how tech has finally caught up with ideas. To a computer, classifying things in the real world can be a bit of a puzzle. We’ve taught ...
This is a guest blogpost by Claus Jepsen, Chief Product and Technology Officer, Unit4 2024 is going to be an important year for the enterprise technology sector as various factors influence ...
This is a guest blogpost by Paul O’Sullivan, UKI CTO and SVP Solution Engineering, Salesforce. The only constant in technology is change and the next revolution is upon us - Artificial ...
-
Embracing AI: understand the end user first
- Senior Analyst, Business Applications 07 Dec 2023 -
Teaching AI common sense an old problem ChatGPT foregrounds
- Senior Analyst, Business Applications 08 Nov 2023 -
We need to give AI more time (series)
- Senior Analyst, Business Applications 07 Nov 2023 -
Generative AI, one year later: moving beyond the hype
- Senior Analyst, Business Applications 16 Oct 2023 -
Transforming industries with an AI-enabled data supply chain
- Senior Analyst, Business Applications 04 Oct 2023 -
How should your SAP strategy evolve in line with SAP’s evolution?
- Senior Analyst, Business Applications 04 Oct 2023 -
GenAI: Boffins in clover
- Senior Analyst, Business Applications 27 Sep 2023
This is a guest blog post by Sara Portell, VP of User Experience and Rafael Artacho, Product Director, Unit4 How we interact with enterprise software, particularly enterprise resource planning ...
This is a guest blogpost by Jim Webber, Chief Scientist, Neo4j Is the best way to end the LLM hallucination problem to teach it some basic logic? Great as they are, we’re starting to have to deal ...
This is a guest blogpost by, Evan Kaplan, CEO, InfluxData. Two contrasting forces are driving the AI revolution: generative AI (GenAI), known for its creative prowess, and real-world applications ...
This is guest blog post by, James Fisher, Chief Strategy Officer, Qlik Almost one year ago, ChatGPT exploded on the scene and quickly took AI mainstream. Since then, an endless number of articles ...
This is a guest blogpost by Vaibhav Vohra, Chief Product and Technology Officer at ERP provider Epicor Essential industries are going through a period of radical transformation, as businesses that ...
This is a guest blogpost by Luiz Mariotto, group vice president, SAP Product Management, Rimini Street. In it he suggests three questions IT leaders should ask about their SAP roadmaps to help ...
When Larry Ellison gave his keynote speech at Oracle Cloud World in Las Vegas last week, it would not have been shocking if he had expressed some scepticism about the hype generated by Generative ...
-
The companies set to win using LLMs are the responsible ones
- Senior Analyst, Business Applications 01 Sep 2023 -
Is the data guardian hinting that Palantir should be ousted from NHS IT?
- Senior Analyst, Business Applications 29 Aug 2023 -
Resisting AI
- Senior Analyst, Business Applications 28 Jul 2023 -
How the high street gains from advanced data analytics
- Senior Analyst, Business Applications 26 Jul 2023 -
Navigating application fragmentation
- Senior Analyst, Business Applications 13 Jul 2023
This is a guest blogpost by Triveni Gandhi, Responsible AI Lead, Dataiku Large Language Models (LLMs) including ChatGPT have seemingly moved from hype, to hot seat, to hazard. In the midst of the ...
Nicola Byrne, the National Data Guardian, published a blogpost on the Gov.uk site on 24 August that seems to suggest Palantir should be excluded from the technology involved in the NHS Federated ...
Technology is not neutral. It is always embedded in social, economic, and political relations built on systematic, structural imbalances of power. Welcome to capitalism. Including the Xi Jinping ...
This is a guest blog post by Phillip Sewell, CEO at Predyktable. The high street is finally back in business, with customers flocking back to stores, restaurants and pubs. To fuel and sustain this ...
This is a guest blog post by Gert-Jan Wijman, VP of EMEA, Celigo. Computer applications have done wonders for bringing people together. From the early days of chatrooms to the rapid rise of social ...