SAS study tour 2024, North Carolina - day 2
The Computer Weekly Developer Network plunged into day 2 of the SAS study tour at the company’s North Carolina headquarters to learn about the company from the inside.
Day two sessions started with a group briefing featuring Jared Peterson, senior vice president for platform engineering; Susan Haller, senior director for advanced analytics; Gordon Robinson, senior director data management; and SAS principal software engineer Joseph Henry.
Peterson kicked off by explaining where much of the drive behind the SAS Viya AI platform has come from.
“Software development in the past was often driven by C-suite initiatives designed to push ideas towards the R&D department in an effort to produce products and services that would enable an organisation to outpace its competitors,” said Peterson. “Given that reality, I think we all felt like it was time to create a development culture that stemmed from a more grassroots level.”
Peterson noted that the company’s software teams wanted to put the SAS language in the hands of a lot more developers and so democratise access to its toolsets via new channels. Specifically, the company thought that SAS should be accessible to more than just SAS language developers and also made easier for Python programmers and other software engineers as the roadmap continues to develop.
It’s YES code
“If we take you through some of the core mechanics of SAS Viya, I would say that this is not low-code or no-code… this is yes code [i.e. it’s coding and data analytics tools that a developer wants to get their hands on and start working with],” enthused software engineering team leader Joseph Henry.
Henry was realistic about how organisations might have to approach the installation of a platform at this level; even with the benefit of installation wizards to accelerate the process, this might take a whole day at best. As a result, SAS has been working hard to make the compute and storage available on SAS Viya Workbench a much more fluid process.
Why make SAS available to more programmers? Because it is essentially an analytics language and can perform analytics functions in far fewer lines of code than a general purpose programming language and the company even has what it calls its ‘Speed Doctors’ – a team focused on acceleration and performance of the platform.
“Integration is always cheaper than invention,” suggested Henry, with a view to just how far SAS Viya might be able to help programmers outside of the SAS universe to grasp new analytics functions.
SAS data management
The only Scot in the village this week, Gordon Robinson, SAS senior director of data management noted that the SAS data management division has doubled in the last few years from around 150 employees to somewhere close to 300. Its core product focus is SAS Studio with its low-code, drag-and-drop functionality and its dedicated Integrated Development Environment (IDE). This part of SAS also hosts the Data Quality division which looks after data concerns such as standardisation, compliance and Personally Identifiable Information (PII) issues. Also here we find teams working on data access so that customers can get the information they need quickly.
Looking at how broadly SAS tooling can be applied across the data universe, the team says that ultimately, the company would like to be database-agnostic and capable of working in every data estate on the planet. Although some custom coding is needed at the SAS end in many cases, this is the company’s bread and butter and it knows the ropes.
Gen-AI, but from a data angle
Susan Haller, senior director for advanced analytics at SAS explained that the company has ‘extended its definition of generative AI’ because it wants to ‘approach it from a data angle’ and from a programming perspective.
“It all starts with data [these days] of course… and in some highly regulated industries we know that there are restrictions on how and when we can work with some data sources (healthcare and finance are obviously good examples), so in these instances, it often makes sense to use synthetic data that has the same ‘distributions’ (in the statistical sense) of the original data so that we can still provide the same analytics services that will ultimately serve AI use cases,” said Haller.
SAS Data Maker has a rich suite of synthetic data algorithms that can produce synthetic data for what are tabular data loads. This approach to data science could also be useful for data analytics focused on extremely rarely occurring events (a patient with a highly uncommon tumour for example), or in parts of the world where data can not be shared across international boundaries.
AI ethics
Into the ethical side of data analytics, we heard from Kristi Boyd, senior trustworthy AI specialist & Vrushali Sawant, data scientist in the SAS data ethics practice.
“Just because we can do something with data, it doesn’t always mean that we should do something with data,” said Boyd. She further noted that we need to be careful about how we apply analytics in any and every given scenario i.e. what works well in one industry does not always translate and transmogrify to another. It’s all about taking responsibility for the strategic oversight of AI within defined business rules and priorities and keeping a close eye on compliance, operations and culture.
SAS AI ethics principles break down as:
- Human-centricity
- Inclusion
- Accountability
- Transparency
- Robustness
- Privacy + Security
The robustness factor is important because an AI model needs to be able to work over a complete use case lifecycle and we need to be cognizant of model drift throughout that same lifecycle period. Given that data changes over time, people and their information changes over time and the fact the world changes over time, a model today may drift out of accuracy in the future and that all forms part of the AI ethics management responsibility.
Rounding out this section of the SAS study tour, Vrushali Sawant explained how the company uses ‘model cards’ to denote the veracity and worth of any given AI model in use.
“It’s rather like an ingredient label on a food packaging,” said Sawant. “The ingredient list in this case would be the data and variables used. The serving size would be the model accuracy. The potential allergens would relate to ‘out of scope’ use cases where and when they happen. Humans should know what they are consuming and AI deployments should also know what they are consuming.”
Remember data mining?
Final sessions in the SAS study tour were presented by Udo Sglavo, vice president for applied AI and modeling R&D and Brett Wujek in his position as principal product manager for AI and Machine Learning at SAS.
Talking about the evolution of SAS and the organisation’s journey to now really operate as an enterprise AI analytics company, Sglavo reminds us that we need to remember our history.
“Let’s not forget, AI used to [just] be called data mining and data discovery,” said Sglavo. “As we now move forward with our modern notion of AI – and also take time to remember that SAS has been building neural network intelligence systems for over 25 years – an algorithm on its own is not much use… it needs to be operationalised (and often productised) into the business so that it can become part of the data-model-discovery lifecycle.”
As this journey unfolds, Sglavo is upbeat about the use of industry-specific solutions that stem from data resources aligned to specific domains, but he says, we should also recognise the fact that solutions aligned for one industry will not always be suitable for another. Some cross pollination can exist, but there is rarely perfect symmetry between domains, practices, industries or individual workflows. Sglavo’s team works to turn models into products by working with consultants in the customer field and dovetailing their work with the engineering teams inside SAS HQ.
SAS Viya Co-pilot
Taking on the last session of the SAS study tour for 2024, Wujek provided an additional overview of SAS Viya Co-pilot and explained how it exists as a central tool to enable individuals to use SAS better.
Wujek explained how SAS Viya Co-pilot features functions for software developers and data scientists to be able to use natural language to describe the features they want in an application or service.
“It can also find ‘problems’ in data such as missing values or skewed distributions that are not representative of the business case being analysed – meaning that there is clearly a need for more data before an analytics procedure would produce really useful insights. As an additional example, this technology also has specialised co-pilots designed to be used in specific use case – the law enforcement module helps police analyse witness statement reports to look for intent and helps to cross-correlate reports and more.”
SAS gave us a deep dive across its platform and tools, it opened up on where it needs to do more, it shared some aspirations for the future and, perhaps most of all, it underlined why so many of its employees stay with the firm for 10, 20, 30 or even 40 years, which certainly bucks the industry norm.
As we leave, we’ll have SAS and Carolina on our mind.
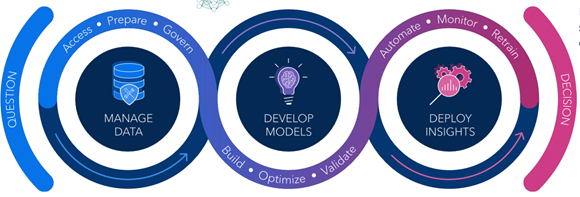
The SAS AI & data developer lifecycle.