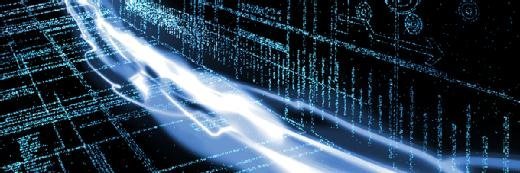
Kit Wai Chan - Fotolia
How Google is priming its infrastructure for the AI wave
The technology giant is integrating purpose-built hardware with an optimised software stack to meet the heightened computational demands of next-generation AI workloads
For over two decades, Google has been priming its infrastructure to support artificial intelligence (AI) workloads, which recently reached an inflection point with large language models (LLMs). These LLMs are expected to place immense strain on datacentres worldwide.
At the core of Google’s approach lies what it calls “workload-optimised infrastructure”, which centres on designing and optimising systems through closer integration between hardware and software.
“AI workloads demand purpose-built hardware, paired with an integrated and optimised software stack capable of meeting entirely new levels of computational demands,” said Mark Lohmeyer, Google Cloud’s vice-president and general manager for compute and machine learning infrastructure.
“With the size of LLMs increasing tenfold per year over the last five years, the only way to effectively train these models and deliver the required performance and scalability at the right cost is to adopt an integrated approach across the entire stack at the systems level,” he added.
Speaking to Computer Weekly during Google Cloud Next 2023, Lohmeyer elaborated on the company’s ongoing efforts to further optimise its infrastructure to support next-generation AI workloads.
These efforts include offering customers a choice of central processors (CPUs), graphics processors (GPUs) and storage options, including block, file and object storage. Additionally, Google is bringing these infrastructure components together into large-scale clusters through advanced networking technologies such as optical switching.
On the software side, Lohmeyer emphasised the importance of having an optimised software stack, leading Google Cloud to invest in open frameworks and compilers optimised for building software that seamlessly integrates with hardware.
He cited the example of Cloud TPU v5e, the latest iteration of the company’s custom-designed tensor processing unit (TPU) – an AI accelerator chip optimised for the training and inferencing of large AI models.
Cloud TPU v5e introduces a multi-slicing capability that aggregates multiple physical clusters of TPUs, allowing AI workloads to harness the compute power of a much larger TPU cluster.
Lohmeyer noted: “It’s extremely powerful because, as an AI researcher, you don’t have to concern yourself with the underlying hardware constructs. You can access this large pool of TPU capacity at a highly cost-effective point.”
During the event, Google Cloud also unveiled the A3 supercomputer, powered by Nvidia’s H100 GPUs and capable of supporting the most demanding generative AI workloads. These typically involve large-scale model training, tuning and serving at a massive scale.
Lohmeyer said that compared to its predecessor, the A2, the A3 boasts 10-times faster networking, enhancing connectivity between chips and delivering three times higher performance for very large models.
While model training traditionally occurs in public or private cloud datacentres, more models are now being deployed at the edge for inferencing. Although currently uncommon due to hardware constraints, future AI training may occur at the edge to safeguard sensitive data and expedite the training process.
To support increasingly distributed AI workloads, Lohmeyer highlighted the Google Distributed Cloud (GDC), offering a choice of AI infrastructure and locations for running AI workloads.
“It’s akin to a Google Cloud instance that you can deploy anywhere – in your datacentres or on the shop floor at retail locations. We are exploring ways to incorporate AI technologies into the Google Distributed Cloud footprint,” he said.
First introduced in 2021, GDC is a fully managed hardware and software service designed for AI and data. It offers a rich set of services, various extensible hardware form factors, and the flexibility to operate connected to Google or fully air-gapped.
Sachin Gupta, vice-president and general manager of infrastructure at Google Cloud, said: “If I’m in a store and need to process orders, but my connectivity is unreliable, I cannot depend on cloud connectivity. That’s where distributed cloud is fantastic. Or, if you’re in a regulated industry or providing services to the government, the air gap of a distributed cloud is also crucial.”
At this year’s Google Cloud Next event, the company announced new integrations between GDC and its Vertex AI platform, offering pre-trained models for speech, translation and optical character recognition, among other AI capabilities, for GDC Hosted customers. These services will be available for preview in the second quarter of 2024.
Gupta believes Google’s ability to provide a true air-gapped environment that can run on as few as three racks, support various AI models and hardware form factors, and its ecosystem of software partners will set GDC apart from competing distributed cloud offerings. “We will provide the best managed services and an open environment for you to build on an infrastructure that is truly modern, providing an experience similar to that of Google Cloud,” he said.
Read more about AI in APAC
- Australia’s Culture Amp is building a generative AI capability that summarises employee survey responses, automating a process that typically takes HR admins up to hundreds of hours to complete.
- Melbourne-based Cortical Labs’ lab grown neurons could speed up AI training in a more energy efficient way and its work has caught the eye of hyperscalers and Amazon’s CTO.
- India’s Cropin, one of the first movers in agriculture technology, has built an industry cloud platform with AI capabilities that is now used by the likes of PepsiCo to maximise crop yields.
- An AI engine developed by Singapore startup EntoVerse is helping cricket farmers improve yield by optimising environmental and other conditions.