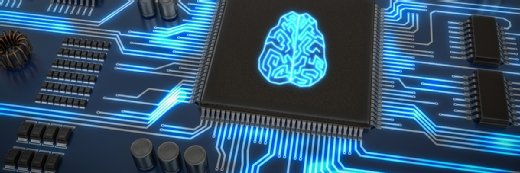
vchalup - stock.adobe.com
Algorithmic transparency obligations needed in public sector
Public sector’s use of algorithms with social impacts needs to be more transparent to foster trust and hold organisations responsible for the negative outcomes their systems may produce, says report
The government should force public sector bodies to be more transparent about their use of algorithms that make “life-affecting” decisions about individuals, says a review into algorithmic bias.
Published by the Centre for Data Ethics and Innovation (CDEI), the UK government’s advisory body on the responsible use of artificial intelligence (AI) and other data-driven technologies, the 151-page review proposes a number of measures that government, regulators and industry can put in place to mitigate the risks associated with bias in algorithmic decision-making.
“It is vital that we work hard now to get this right as adoption of algorithmic decision-making increases,” said CDEI board member Adrian Weller. “Government, regulators and industry need to work together with interdisciplinary experts, stakeholders and the public to ensure that algorithms are used to promote fairness, not undermine it.
“The Centre for Data Ethics and Innovation has today set out a range of measures to help the UK achieve this, with a focus on enhancing transparency and accountability in decision-making processes that have a significant impact on individuals. Not only does the report propose a roadmap to tackle the risks, but it highlights the opportunity that good use of data presents to address historical unfairness and avoid new biases in key areas of life.”
The review notes that bias can enter algorithmic decision-making systems in a number of ways. These include historical bias, in which data reflecting previously biased human decision-making or historical social inequalities is used to build the model; data selection bias, in which the data collection methods used mean it is not representative; and algorithmic design bias, in which the design of the algorithm itself leads to an introduction of bias.
Bias can also enter the algorithmic decision-making process because of human error as, depending on how humans interpret or use the outputs of an algorithm, there is a risk of bias re-entering the process as they apply their own conscious or unconscious biases to the final decision.
“There is also risk that bias can be amplified over time by feedback loops, as models are incrementally retrained on new data generated, either fully or partly, via use of earlier versions of the model in decision-making,” says the review. “For example, if a model predicting crime rates based on historical arrest data is used to prioritise police resources, then arrests in high-risk areas could increase further, reinforcing the imbalance.”
The CDEI also noted that “making decisions about individuals is a core responsibility of many parts of the public sector”, and, as such, the government should place a mandatory transparency obligation on all public sector organisations using algorithms to fulfil this responsibility, which would help to “build and maintain public trust”, as well as introduce higher levels of accountability.
“Government should conduct a project to scope this obligation more precisely, and to pilot an approach to implement it, but it should require the proactive publication of information on how the decision to use an algorithm was made, the type of algorithm, how it is used in the overall decision-making process, and steps taken to ensure fair treatment of individuals,” it said.
“The Cabinet Office and the Crown Commercial Service should update model contracts and framework agreements for public sector procurement to incorporate a set of minimum standards around ethical use of AI, with particular focus on expected levels transparency and explainability, and ongoing testing for fairness.”
Read more about algorithms
- Home secretary has pledged to stop using an algorithmic decision-making system to assess visa applications after migrant welfare and technology justice groups challenged the tool for helping to create a hostile environment for migrants.
- Strict ethical and professional standards should be applied to the development of algorithms with social impacts to recover public trust in the technology, according to a report by BCS, the Chartered Institute for IT.
- A new set of nationally approved guidelines is needed to ensure police algorithms are deployed in lawful and ethical ways, claims a report by the Royal United Services Institute security think tank.
But the CDEI said that if this information is not intelligible, it could fail to inform the public and foster even greater concern. Any transparency-related publications should therefore be easy to find, understand and use, and should absolutely avoid being used to serve narrow communications objectives or to purposefully manipulate an audience, it said.
“We should be able to mitigate these risks if we consider transparency within the context of decisions being made by the public sector and if it is not seen as an end in itself, but alongside other principles of good governance including accountability,” said the CDEI.
It called for the government to issue guidance that clarifies the application of the Equality Act to algorithmic decision-making, which should include information on the collection of data to measure bias, as well as the lawfulness of various bias mitigation techniques that could lead to positive discrimination.
Organisations in both the public and private sectors should also be using data to actively identify and mitigate bias, meaning they must understand the capabilities and limitations of their algorithmic tools, and carefully consider how they will ensure fair treatment of individuals, it said.
But the CDEI warned: “Bias mitigation cannot be treated as a purely technical issue; it requires careful consideration of the wider policy, operational and legal context.”
The review recommends that government and regulators provide clear guidance on how organisations can actively use data to tackle current and historic bias, which should address the misconception that data protection law prevents the collection or usage of data for monitoring and mitigating discrimination.
Gemma Galdon Clavell, director of Barcelona-based algorithmic auditing consultancy Eticas, has previously told Computer Weekly how AI-powered algorithms have been used as a “bias diagnosis” tool, showing how the same technology can be re-purposed to re-enforce positive social outcomes if the motivation is there.
“There was this AI company [Ravel Law] in France that used the open data from the French government on judicial sentencing, and they found some judges had a clear tendency to give harsher sentences to people of migrant origin, so people were getting different sentences for the same offence because of the bias of judges,” said Galdon Clavell.
“This is an example where AI can help us identify where human bias has been failing specific groups of people in the past, so it’s a great diagnosis tool when used in the right way.”
However, she noted that the French government’s response to this was to not to address the problem of judicial bias, but to forbid the use of AI to analyse the professional practices of magistrates and other members of the judiciary.