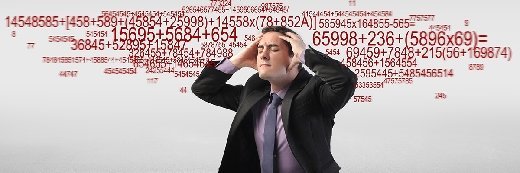
olly - Fotolia
Interview: Innovating with data science
Amadeus’s head of data science for innovation, Baptiste Chatrain, discusses his GitHub page and how the business innovates with data
Prior to meeting someone new, it is often a good idea to check their LinkedIn profile. Where have they previously worked? Which college did they attend? Who is in their network of contacts?
The power of the web provides an instant snapshot of someone’s CV. But Googling Baptiste Chatrain, the head of data science for innovation and AI research at Amadeus IT group, it is his Github page that perhaps offers the best introduction.
Chatrain has made 750 contributions to about 50 open source projects in the last year, and has repositories ranging from a self-flying quadcopter to a working prototype for capturing frames off a live MJPEG video stream.
The repositories illustrate Chatrain’s background as a programmer. “Code is important,” he says. “Even though I am in the data science group, I’m still coding every day.” For him, coding is a skill that helps data science, and the data science innovation and AI research group encourages collaboration with the developer community.
“It is our responsibility to show the value of innovation. Some of the things we learn need to be applied quickly,” says Chatrain. Coding provides the means by which the team can find the shortest path to deliver value.
The team comprises 15 data scientists mainly based in Nice, with two staff in Madrid. The group works across the business units and on its own internal projects.
“We are part of a factory,” he says. “We turn ideas into value. The inputs are the ideas.” The results are driven by the needs of the business to grow. As well as the internal aspect of the work and working with business units, the team also works with some external customers such as airlines and airports.
Wine is fine
In a demonstration at the Transforming Tomorrow’s Travel (T3CH) conference in Madrid, showcasing some of the data science innovation at Amadeus, Chatrain discussed how cognitive bias has an effect on people’s buying habits.
The demo illustrates what happens if someone is presented with the choice of purchasing either a €5 or a €15 bottle of wine. He says that if the wine is to accompany a pizza, then the customers would likely select the cheaper one.
However, if there’s a third wine at €30, more often than not, the customer would select the middle price – the €15 bottle. Improvising, by showing a €30 wine, even though it will not be bought, has been proved to encourage the customer to spend an extra €10 on a bottle of wine. It is such insights that make data science innovation a hot topic in many organisations.
Read more about Amadeus
- Amadeus is adding to the business intelligence capability it has been building since 2013. Its head of travel intelligence says industry at start of data analytics revival.
- Amadeus, the airline flight ticketing system, has used Google Compute Platform to scale its service to support internet flight searches.
Exploring data
Exploratory research conducted by the team is used to feed new ideas. Amadeus also uses a collaborative platform to collect ideas from across the business. These ideas may be based on looking at how other industries use data, or by looking at issues that are specific to the travel sector.
In addition to the internal work, he says Amadeus also runs a startup incubator programme. “Our Explore programme collaborates with startups to exchange ideas. APIs [application programming interface] with developers create an ecosystem of new ideas.”
Sometimes, rather than look at problem areas in the business, he says the team focuses on exploring pure technology. As an example, Chatrain says Generative Adversarial Networks (GANs) can benefit from algorithms that generate fake data, such as fake pictures of people who do not actually exist. “We dedicate part of our exploratory time to such techniques and technologies and then look for applications,” he says.
Looking at a practical example of how a fake data algorithm could be deployed, he says: “With GDPR and the need to feed test systems with high volumes of realistic data, we used [synthetic data algorithms] to create fake travellers with travel itineraries.”
Such synthetic data is indistinguishable from the data that represents the travel plans of real people, and this data can be used to test the robustness of systems at Amadeus. “Today, no one tests the systems if we have twice as much data,” says Chatrain.
But this is possible if data for a vast increase in passenger numbers is simply generated via a synthetic data algorithm. Beyond being used to test application software, he says synthetic data also enables Amadeus to anonymise the data it shares with third parties. “We are not allowed to share [personal] data, but we still need a business partnership.”
Priorities
Skills and the challenges in innovating with data science are often high up on the priority list for data scientists. Addressing the skills question, Chatrain says: “You need multiple skills in data science. We use pure applied mathematics and operational research. Part of the team has a data engineering role.”
The team can sometimes be regarded as disruptive. This is the change management aspect of data science.
“To prove value, you have to address change management,” says Chatrain. “You have to be a change agent, and work with the users in business to evangelise and show the true value.” For instance, the team may need to spend time showing stakeholders how a human performs a task, compared to a data model running on a machine.
“This can be challenging, but it is the only way to incrementally deliver products that add value,” he says.