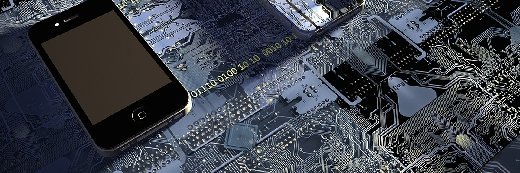
juanjo tugores - Fotolia
Intelligence on the edge will see workloads migrate from clouds
IoT devices’ ability to make their own decisions will see computing shift back from the cloud to the edge, ABI Research has predicted
The need to support artificial intelligence (AI) on the internet of things (IoT) will see processing migrate from the cloud back onto devices, according to a report from ABI Research.
The market for edge AI inference will grow from just 6% in 2017 to 43% in 2023, ABI predicted.
Jack Vernon, industry analyst at ABI Research, said: “The shift to the edge for AI processing will be driven by cheaper edge hardware, mission-critical applications, a lack of reliable and cost-effective connectivity options, and a desire to avoid expensive cloud implementation.”
Scaling hardware to a point where it becomes cost-effective will enable more verticals to begin moving processing out of the cloud and on to the edge, said Vernon.
ABI has identified 11 verticals that are ripe for AI adoption – automotive, mobile devices, wearables, smart home, robotics, small unmanned aerial vehicles, smart manufacturing, smart retail, smart video, smart building, and the oil and gas sectors – and split across a further 58 use cases. By 2023, it said, the market will see 1.2 billion shipments of devices capable of on-device AI inference – up from 79 million in 2017.
The analyst company said it expects cloud providers to remain pivotal, particularly when it comes to AI training. Of the three billion AI device shipments forecast to take place in 2023, more than 2.2 billion are expected to rely on cloud service providers for AI training.
However, this is a real-term decline in the cloud providers’ market share for AI training, which currently stands at around 99% but will fall to 76% by 2023, it said.
According to ABI Research, power-efficient chipsets will be the main driver behind edge AI. For instance, Huawei has introduced on-device AI training for battery power management in its P20 pro handset, in partnership with Cambricon Technologies. Meanwhile, Nvidia, Intel and Qualcomm are also looking at on-device AI training to support their efforts in autonomous driving.
Read more about AI at the edge
- When implementing AI, it is important to focus on the quality of training data and model transparency to avoid potentially damaging bias in models.
- Sometimes it is better to manufacture training data for machine learning models than it is to collect it.
“The massive growth in devices using AI is positive for all players in the ecosystem concerned, but, critically, those players enabling AI at the edge are going to see an increase in demand that the industry, to date, has overlooked,” said Vernon. “Vendors can no longer go on ignoring the potential of AI at the edge.
“As the market momentum continues to swing toward ultra-low latency and more robust analytics, end-users must start to incorporate edge AI in their roadmap. They need to start thinking about new business models, such as end-to-end integration or chipset as a service.”