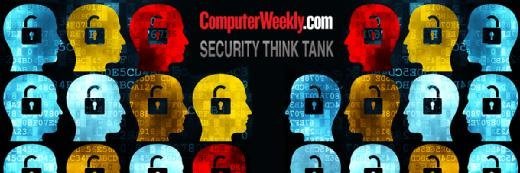
Maksim Kabakou - Fotolia
Security Think Tank: The past and future of security automation
Artificial intelligence and machine learning techniques are said to hold great promise in security, enabling organisations to operate an IT predictive security stance and automate reactive measures when needed. Is this perception accurate, or is the importance of automation being gravely overestimated?
There was a time when security analysts trawled through packet capture and log files trying to identify and diagnose potential intrusions. Looking for a cyber attack within these log files was often likened to trying to find a needle in a haystack.
However, it would be more accurate to say that hunting for an unknown cyber intrusion is more like looking for an unknown needle-sized object that had been broken into pieces and scattered in a large haystack.
Today’s systems may have 8,000 to 10,000 events per second – or approaching one billion per day – for an analyst to look through, so the use of automation to detect and analyse these events to identify potential attacks is essential. Visualisation of the results so the analyst can see the alerts and drill down to see what’s going on is also necessary so that the alert can be understood and a response developed.
Most security operation centres (SOCs) will use a variety of detection and analytic tools, ranging from signature-based antivirus and intrusion detection systems (IDSs), through to artificial intelligence (AI)-based tools performing anomaly detection and looking for low-level indications of an attack based on host and network monitoring.
We have also had decision-making support for some time, which can recommend potential courses of action to the user based on a play book and knowledge of the system – for example, the network architecture, servers providing critical services, or storing critical data and placement of security functions such as firewalls, proxies and IDSs that can be used to block traffic to specific domains.
Some platforms are starting to automate these actions, either following a human decision, or taking the human out of the loop altogether. Full automation will save the analyst time, but there is a risk of automated responses becoming predictable.
This predictability could be used by an attacker to find out if they have been detected, to cause a diversion from the real intent, or even to deny service by faking an attack. I have no evidence of this happening so far, but it is definitely possible and I believe very probable.
While we still use signature-based tools such as antivirus and IDS, it is almost compulsory now for new tools to be AI-enabled. There are different types of AI, the most common of which is machine learning (ML), other examples of technologies labelled as AI are neural networks and machine reasoning, but generally I would put all tools into two categories – deterministic and probabilistic. Signatures, analytic use cases and machine reasoning being deterministic, because the decision is traceable and the result consistent. ML is probabilistic, because it is based on statistics and probability.
Read more from this Security Think Tank series
- Artificial intelligence will be no silver bullet for security.
- AI cyber attacks will be a step-change for criminals.
- Get your house in order before deploying AI.
- SIEM and AI – a match made in heaven?
- Balancing human oversight with AI autonomy step-change for criminals.
- Ignore AI overheads at your peril step-change for criminals.
Machine learning is based on learning patterns from a large amount of data, with data sets typically representing “good data” and others representing “bad data”. The more data and the more representative the data, the more accurate the results. The algorithm derived from the learning is then used to process real-world data to see if it looks more like “good data” or “bad data”.
The result is usually based on at least a dozen parameters each with its own weighting, or threshold. This works well for well-defined problems such as face recognition or analysing cancer scans, but less well for poorly defined problems, particularly where context is important.
Take facial recognition, for example. You know what you are looking for (a specific face) and a face can be defined by only 80 nodal points. Even so, some systems can have a high false-positive rate. Cyber attacks are more complex and also require the context of the individual system.
That is not to say that we should not be using AI, just that we need to understand the capabilities of the individual tools, what they provide and how they fit into the overall tool chain so as to pick the right tool for the job.
Also, it is only possible to judge how effective a ML-based tool will be once it is deployed; false-positive rates will vary depending on the match between the training data and the characteristics of a specific network, so if a false positive rate is quoted, then it is important to understand what data was used to derive that figure.
Also, when an alert is triggered, it is essential that the events causing the alert are available to an analyst to investigate. ML systems that continue to learn from your data can help them adapt to specific systems, but will need an extended learning period to bed in.
This is also the case with anomaly detection systems based on other AI technologies, because anomalies are very specific to system context, the types of user and nature of the business.
We are however becoming more dependent on AI-based systems for detection, as well as in other areas such as decision-making and response.
While it is important that we respond to an attack as soon as possible, and AI systems have and are progressing at an accelerated pace, I believe their place is as a support tool for the analyst identifying potential attacks and providing decision support.
Decisions made in response to an attack require not just technical input, but business context. No chief information security officer (CISO) would come out well if a false positive led to the automatic shutdown of a conferencing system while the CEO was in the middle of a critical business negotiation.
Another area where AI and automation is proposed is the concept of predictive security. These systems are just emerging and detailed information on how they work is not sufficiently available to make any real judgement.
At the beginning of July 2001, I was preparing for a presentation at a conference in London when I saw some information about a potential threat to Microsoft’s IIS web server based on what appeared to be someone testing a new exploit. I included this in my presentation with a prediction that we would see a significant attack on MS IIS servers in the next two weeks.
By 19 July, more than 350,000 IIS servers had been hit by the Code Red worm. I have never been so lucky with a prediction and the timing since. It is this type of early activity that I believe these predictive systems are looking to find and exploit.
The concept is a little like automated vulnerability management, but using AI to search through huge amounts of data from hundreds of thousands of endpoints across the globe to identify the signs of vulnerability exploitation and identify endpoints that are vulnerable to the same attack before there is wide spread exploitation of the vulnerability. This would then allow patches to be applied, or mitigations to be made.
This could be a successful strategy, but could realistically only be provided as a service by some of the larger cyber security players because of the large amounts of data required. This would need to be collected from many sites around the world with as much business and political diversity as possible.
Also, it does bear some similarities to predictive security used in law enforcement (particularly in the US) to predict crimes and their perpetrator.
As with those systems, the issues with predictive cyber security are likely to be ethical, concerning the General Data Protection Regulation (GDPR) and protection of the personal information that could be gleaned from the data collected from individual hosts and analysed in the cloud. This will certainly need consideration from a GDPR standpoint. The risk of false positives and automation also remain.
The use of machine learning in cyber security has come a long way over the past few years and is proving itself as part of the tool chain. There are, however, a large number of solutions out there, some of which are making claims that are difficult to test and which they may not live up to. It is therefore important to be careful to use the appropriate technology and see how it performs before committing.
Decision-making support is critically important, but I believe more confidence is needed before taking the analyst out of decision-making. Predictive security will come and will have similar issues, but also privacy issues. Such systems could, however, prove extremely powerful. The only thing I will predict is that their predictions will be more successful than mine.
Read more about AI in security
- Vendors and customers must be aware of potential gaps between expectations and reality in the sale or purchase of AI cyber security products, an AI security expert advises.
- Prudential, the UK’s largest listed insurer, is turning to artificial intelligence to protect its computer networks in the US, Asia and Africa from malware, hackers and internal threats.
- Experts offer four concerns for enterprises and vendors to discuss in order to deploy and run AI-based cyber security tools.