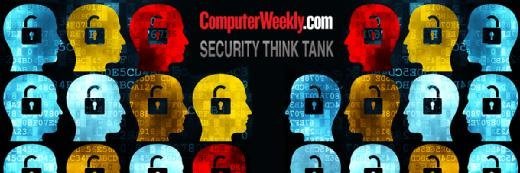
Maksim Kabakou - Fotolia
Security Think Tank: Get your house in order before deploying AI
AI and machine learning techniques are said to hold great promise in security, enabling organisations to operate a IT predictive security stance and automate reactive measures when needed. Is this perception accurate, or is the importance of automation being gravely overestimated?
If I start by saying that yes, I believe the perception that artificial intelligence (AI) and machine learning (ML) will offer great strides forward in security to be correct, then I need to also caveat it with the assertion that security will need to be done well already for these new approaches to be anywhere near as effective as everyone wants to believe them to be.
It is true to say that AI and ML offer great promise when it comes to organisational security measures. A predictive security stance may be some way off for many businesses and the belief that AI or ML will dissolve existing poor practice or protocols is as widespread as it is erroneous.
Before really talking about AI and ML, we must talk about bias and the impact it has on quality outcomes from either technology. Bias will simply double down on any practice or protocol in place and reinforce it, good or bad.
You don’t have to look very far to see an example of how it can go wrong if you have not considered the bias problem – Amazon was forced to scrap its experimental AI recruitment tool, as it eventually decided the best people for its roles were pretty much just men.
In simple terms, the data it was given about who was currently performing roles or had applied for those roles in the past 10 years became its bias and it applied that to applicants. The roles it was being tested on were technical roles and the vast majority of applicants for those roles were male.
As with any form of education, you get out what you put in and you cannot expect it to interpret your wishes. If you look at Microsoft’s experimental chat bot, Tay, you see how firmly we need to be in control of the inputs and how mindful they should be.
Designed to be reactive and develop based on its interactions with the good folk of the internet, Tay became a Nazi ideology spouting, sexist bully on Twitter, within a day. Even Microsoft was not prepared for this rapid descent. That says a lot. The intelligence wrote what it thought its creator wanted to read.
Apply this to security, then, and we can soon see some potential pitfalls if businesses imagine that AI or ML is going to be the next panacea for poor security – has any technology to date ever actually become the panacea despite the sales hype?
You can automate and speed up your poor security practices if you wish, but you won’t cure them. So many security incidents are caused by poor configuration or a lack of understanding of business processes, placing users in the precarious position of using insecure platforms, devices or software, but also being frequently blamed for the failings that inevitably occur.
We need our technology, our processes and our users to be fit for the environment it is actually in, not the one we wish it was. So, before we start turning to AI or ML for solutions, we need to make sure our house is in order first. Neither should impede the users’ ability to have the right data assets, in the right format at the point of need nor should any technology ever replace the need for effective risk management.
So whatever methodology we use needs to satisfy the business needs first, the technology second and the security should be an encompassing enabler for those two things, with or without AI.
Read more about AI in security
- Vendors and customers must be aware of potential gaps between expectations and reality in the sale or purchase of AI cyber security products, an AI security expert advises.
- Prudential, the UK’s largest listed insurer, is turning to artificial intelligence to protect its computer networks in the US, Asia and Africa from malware, hackers and internal threats.
- Experts offer four concerns for enterprises and vendors to discuss in order to deploy and run AI-based cyber security tools.