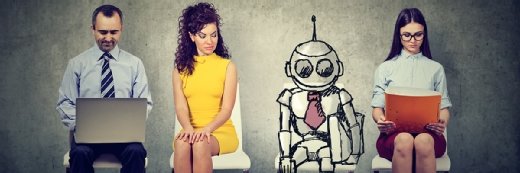
pathdoc - stock.adobe.com
How AI can remove bias from decision-making
Much is said about the risks of embedding human bias into artificial intelligence and algorithms, but one economist's theories suggest that AI could actually deliver the opposite
The UK government recently published a review of algorithmic bias – an important and even crucial subject as ever more decision-making progresses from wetware to silicon. However, it would have been useful if they’d understood what Gary Becker told us all about discrimination itself – work for which he won the Nobel prize for economics. Almost all the things they are worrying about solve themselves within his logical structure.
First though, a linguistic structure – let’s examine the difference between algorithms and artificial intelligence (AI). An algo doesn’t have to be encoded at all, it’s a set of rules by which to make a decision – usually, almost always, derived from the current methods by which we make such decisions, just formalised or even coded.
AI is often the opposite way around. Here’s the data, now what does that tell us? Often enough, in our modern world, we don’t know what the connections are – the machine just insists they’re there. It’s entirely common in financial markets that the AI trades connections that no one knows about, even those that own it.
The worry in the report is that increased use of algorithms could, or will, entrench the current unfairness we know is hardwired into our societal laws and decision-making. They are right. Although this is a point they don’t make, this has to be true for the algos to work.
We are, after all, trying to produce a decision-making system for our current society. So it has to work with the current rules of the world around us. Algos that don’t deal with reality don’t work. The solution to this requires a little more Gary Becker in the mix.
Taste vs rational discrimination
Becker pointed out that we can, and should, distinguish between taste discrimination and rational discrimination. One oft-repeated finding is that job applications with an apparently black name such as Jameel gain fewer calls to interview than something apparently white, such as James or Rupert. This is largely “taste” discrimination or, as we’d more normally put it, racism. Repeat the logic with whichever examples you prefer.
The point is that we wholly desire to eliminate taste discrimination precisely because we do – rightly – consider it unfair. And yet there’s a lot of rational discrimination out there that we have to keep for a system to work at all. Rupert’s – or Jameel’s – innumeracy is a good reason not to hire him as an actuary, after all.
Becker goes on to point out that taste discrimination – and his specific example was the gross racism of mid-20th century America – is costly to the person doing it. Yes, of course it’s costly to those discriminated against, but also to the person doing it. For they have, by doing so, rejected entirely useful skills and employees.
But the more society as a whole does this to a specific group, the cheaper such labour becomes to iconoclasts willing to breach the taboos – who then go on to outcompete the racists. Those “Jim Crow” laws in that time and place were an acknowledgement of this.
Only by the law insisting on the racism ending could the sidestepping of it in pursuit of profit be stopped. Free market forces, eventually at least, break such algorithms of injustice.
Human oddity
Which brings us to the AI side of our new world. Given the definition I am using, this is a matching of patterns that is entirely free of taste discrimination. No human designed the decision-making rules here – by definition, we’re allowing the inherent structure of the data to create those for us.
So those bits of the human character that lead to racism, misogyny, anti-trans bigotry and the rest aren’t there. But the parts that hire the literate to write books remain – we have a decision-making process that is free of the taste discrimination and packed with the rational.
Look at this Becker theory another way. Say, women are paid less. They are. Why? Something about women’s choices? Or something about the patriarchy? An algorithm could be designed to assume either.
An AI is going to work out from the data that women are paid less. And then – assuming it’s a recruitment AI – note that women are cheaper to employ, so it hires more women. Which then, over time, solves the problem. That is, if it’s patriarchy, human oddity, that causes women to be paid less, AI solves it. If it was women’s choices, then what needs to be solved?
There is some fun in the aside that we can’t go and examine this to ensure that it’s true. Because the entire point of the AI is to find the patterns we don’t know are there. If we are designing, then we are making algos, not AIs. Any such design brings in those human logical failures, of course.
Leaving the aside, well, aside, as it were, an AI will be working simply on what is, not on what we think is, nor even on how we think it should be. That is, we have now built a filter to allow only Becker’s rational discrimination because the rules by which decisions are made can only be those that are actually there, rather than imposed by the oddities of homo sapiens’ thinking processes.
Missed opportunity
This last point is precisely why some people are so against the use of AI in this definition. For if new decision-making rules are being written, there is an insistence that they must incorporate society’s current rules on what is to be considered fair.
This is something the report itself is very keen on – we must take this opportunity to encode today’s standards on racism, misogyny, anti-trans and the rest into the decision-making process for the future. Which is to rather miss the opportunity in front of us.
What we actually want to do – at least, liberals like me hope – is to eliminate taste discrimination, both pro and con each and every grouping, from the societal decision-making system. And be left only with that rational distinction between those who are the round pegs for the circular holes and those who are not.
AI can be a cure for the discrimination worries about algorithms. For they are the bias-free rules abstracted from reality, rather than the imposition of extant prejudices. It would be a bit of a pity to miss this chance, wouldn’t it?
Read more about economics and IT
- What if we find we rather like this working from home thing?
- Our data is making us “richer” – even if it’s the tech companies getting rich.
- The Google antitrust case is more about politics than economics.