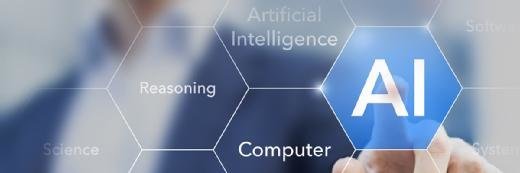
NicoElNino - Fotolia
Five steps to build an artificial intelligence strategy
Artificial intelligence is the next frontier for IT in businesses, helping to increase competitive advantage. A truly successful AI strategy needs careful planning
The competitive business landscape is ripe for the use of artificial intelligence (AI)-driven to set organisations apart. Yet, many are still treating AI like a sidekick: underworked and underestimated.
An AI strategy, operating model and solid execution framework all play a vital part in ensuring that the technology works in the interests of the organisation.
Aligned to business objectives and singular in its commitment to learning powerful automated processes to get the job done, AI has the potential to be a team’s ultimate superhero.
1. Ensure your goals guide your corporate strategy
During AI transformation projects, companies often make the mistake of separating the vision from the execution, resulting in disjointed and complicated AI programs that can take years to consolidate. This can be easily avoided by choosing AI solutions based on concrete business objectives that have been established at the project’s outset.
It’s important to align your corporate strategy with measurable goals and objectives to guide your AI deployment. Once complete, the strategy can be easily escalated down into divisional- or even product-level strategies.
2. Assemble a multi-skilled team
Form a multidisciplinary team to assess how the AI strategy can best serve their individual needs. Having members from different departments in your AI team – for example, web design, research and development (R&D), and engineering – will ensure your strategy will meet objectives for key internal stakeholders.
You may not deploy the right strategy in the first instance, so iteration is crucial. By fostering a culture of experimentation, your team will locate the right AI assets to form your unique competitive edge.
3. Pick the right battles to fight
This might seem like common sense, but the problems you’re looking to overcome have a large impact on your success. Some problems are not AI problems at all, and for the ones that are, the business should advocate the delivery through small ‘lighthouse’ projects that act as a beacon for their capabilities.
By identifying ‘lighthouse’ projects, your business will need to assess the overall goal and importance of the project, its size, likely duration and data quality. Aim to get the project done in eight weeks for maximum value and immediate impact.
From here, its success will increase AI’s profile across the business, empowering teams to deploy it, and allowing the AI to grow in autonomy and understanding.
4. Hit your KPIs
Customer-centricity has become one of the most popular topics among today’s business leaders. No longer are products built and then customers found. Your AI strategy should therefore measure success in relation to customer-centric key performance indicators (KPIs).
For instance, call centres often cause frustration due to poor data handling, leading to a drop in brand reputation. Improving customer experience can be achieved by increasing call-handling speed – a key KPI, by deploying AI to automate and so speed-up the business process.
AI can speed up call-handling with natural language processing (NLP). A slick AI-driven chatbot can ask key questions to determine whether the customer needs to speak to a live agent – and, if not, it can arrange a booking by itself. If the customer does need to chat to the call centre agent, the system can recall data from previous logs in a matter of seconds, sending them to the right agent.
By bypassing manual work, AI speeds up the entire process, hitting the KPI. As such, customer problems can be resolved far quicker and experience improves.
5. Repeat, repeat and repeat again to build best practices
The journey to business-wide AI adoption will be iterative and continuous. On successful completion of a product, the team should evolve into what’s known as an ‘AI community of practice’, which will foster AI innovation and upskill future AI teams.
Using AI for one-off experiments isn’t getting the best value from it. Data science is about repeatable experimentation and measured results – take frequently asked questions (FAQs), for example. If your customers are expressing confusion at your product on Twitter, AI can spot this trend. Further than merely alerting the team, it takes matters into its own hands, coordinating a response before the brand's reputation drops.
AI can automate the response by scanning for frequently occurring queries across social media, mobile searches and page hits, before consolidating into a trend alert, and funnelling this directly to the content writing team. From here, they can continually write and publish answers on an FAQ page before the confusion spreads further.
With this repeatable process, you’ll never be more than one step away from reassuring the customer. Contrastingly, if AI processes are non-repeatable and everybody is changing production by hand, then it is no longer data science but a data hobby.
As with any successful project, the formula for enterprise-wide AI adoption is to nurture the idea, plan, prove, improve and then scale.
Summing up, ‘lighthouse’ projects will need to be proven to work. Teams will need to be upskilled, processes will need to be streamlined, and there will be mistakes made and lessons learnt. And all of this is okay.
While your AI strategy will evolve, the focus should continue to be on a culture of learning and continuous improvement and it should always be driven by your business objectives, not the other way around.
Michael Chalmers is the managing director for Europe, Middle East and Africa at Contino.
Read more about artificial intelligence
- Artificial intelligence and machine learning storage is not one-size-fits-all technology. Analytics work differs, and has varied storage requirements for capacity, latency, throughput and IOPS. We look at key decision points.
- 5G and AI can be combined to improve the network speed, responsiveness and efficiencies of organisations in the enterprise, but the former needs more time to mature.
- A document has been published outlining the challenges for public sector buyers as well as best practices on procuring AI technology.