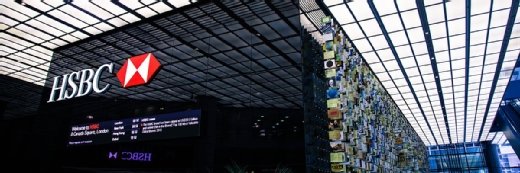
HSBC adopts cloud-first strategy to solving big data business problems
David Knott, chief architect at banking giant HSBC, tells Computer Weekly about how machine learning, big data analytics and cloud are changing the way it does business
HSBC has revealed how the success of five machine learning-related pilot projects is set to pave the way for deeper and faster adoption of cloud technologies across its business.
Speaking to Computer Weekly, David Knott, the international banking giant’s chief architect, says the organisation is in the “early days” of its overall journey to the cloud, but a growing desire to do more with its big data reserves looks set to accelerate things.
“Managing data is a core competency for us, and we found we have loads and loads of really important and really fascinating business opportunities that rest on the use of data,” he says.
The organisation has identified five areas where big data analytics could open up new lines of revenue.
For instance, by assisting the bank with its investigations into anti-money laundering, risk analytics, risk reporting, while also helping it cut down the time it takes to conduct valuation and finance liquidity assessments.
“We deliberately picked projects that were real business problems, because we didn’t want to do a meaningless proof of concept that was kind of interesting to us but didn’t really solve anything,” says Knott.
“We chose those five areas because they are important, but they’re not so big that we’re betting the bank on the success or failure of these things.”
Some of these use cases, such as the bank’s anti-money laundering activity, requires sifting through billions of transactions looking for suspicious activity, and the organisation wants to use machine learning models to cut down the time it takes to do this work and improve its accuracy.
Do it yourself vs buy it in
The company considered building out its in-house big data analytics capabilities to tackle these five areas, but the process turned out harder than expected. Particularly with regard to sourcing, integrating and scaling the technologies that could help it make sense of this data, says Knott.
“That marketplace is extremely richly served, developing extremely fast and has an awful lot of choices to make in it.”
“I was trying to put together a set of technologies, integrate them coherently, and scale them, but building all the capabilities internally to do that has proven difficult,” he says. “I don’t think that’s an uncommon experience to other large enterprises.”
There was also a danger that going down the “do-it-yourself” route would result in the “smart, busy people” Knott employees spending too much time building and managing a stack of heterogeneous big data technologies rather than gleaning business-benefiting insights from them.
On the back of these realisations, Knott and his team began investigating whether adopting a cloud-first approach to tackling its big data issue might be the way forward sometime last year.
“There was a huge appetite to do this, but we also needed to satisfy ourselves that cloud is safe and secure, our regulators are happy, and all the important people are comfortable with what we are doing,” he says.
“So, we said, we’ll take these five cases all the way through to production to prove our security posture and help us build capability internally – and clear a safe path for ourselves to the cloud.”
Read more about Google Cloud
- Fei-Fei Li, Google’s chief scientist of artificial intelligence and machine learning, talks up societal benefits of making artificial intelligence tools and technologies accessible to all.
- With Amazon Web Services’ growth rate continuing to decline over successive quarters, what does this mean for its cloud rivalry with Microsoft and Google?
Each use case has had a team of about half a dozen people assigned to working on it, says Knott, which is a considerably smaller number than HSBC has traditionally used to deliver projects in the past.
“We’re a large enterprise and have traditionally constructed large project teams to do anything big and complicated and one of the reasons we’ve done that is because those teams have had to build everything from the ground up,” he says.
“For this work, each of the team has consisted of a handful of people, backed by a slightly larger central team, worrying about security and the legal side of things, for example, but even that has been less than a 1,000 people.”
Evaluating the market
The company initially embarked on conversations with a number of cloud providers about how they could help HSBC make better use of its big data, before the company settled on using Google.
“Part of the reason we liked Google is that they invented a lot of this stuff. Hadoop, for example, is based on the MapReduce whitepaper Google wrote back in 2004, and they’ve carried on building and innovating for themselves as well as their business,” says Knott.
Google has a growing array of machine learning tools and technologies in its portfolio, such as the Google Cloud Machine Learning Engine, which is a managed service that helps organisations create machine learning models for any size or type of dataset.
The company also has a collection of callable application programming interfaces (APIs) to train their machine learning models, which Knott cited as particularly appealing to HSBC and its use cases.
“So if I can call an API, I can access a machine learning algorithm,” says Knott. “They’ve also created enablement capabilities, such as their Advanced Systems Lab, which is a four-week course where people learn how to do machine learning,” he says.
Proving its worth
The company embarked on a series of proof of concepts with Google, with the aim of answering three questions, says Knott.
“We needed to know, could we get the results we wanted? Was it economic to use cloud, and was it easier to do it this way than trying to do it ourselves?” he says. “The answer to the first two was a firm yes, and the answer to the last one was a very firm yes. It was enormously easier.”
The company is now on the verge of putting the original five pilots into production, before applying all it has learned to a new set of use cases, and accelerating the spread of cloud-based data analytics and machine learning tools through the rest of the business.
“We’ve largely been running these pilots with manufactured data and test data, so when we go live, we’ll be putting our real data in the cloud and collecting the outputs of those models and feeding that back into our process,” he says.
“Once we’re live, which we very nearly are, that opens up the path for us to take the next set of use case and then the next set of use cases after that, because there is this huge, pent-up demand for this across the business.”
To back this point, Knott claims a meeting with HSBC’s retail banking arm recently unearthed around 90 additional use cases for machine learning within that division alone.
Mixing old and new
The organisation is no stranger to using complex algorithms, data modelling and data analytics in its risk and financial crime detection units, for example, but the viability of using machine learning has only really come to the fore in the past year or so, says Knott.
“A year or two ago, we had lots of niche product suppliers trying to sell products into companies they would install and manage themselves, and in some cases it was quite difficult to understand how to deploy and manage them,” he says.
The Google engagement is also far from being its first foray into cloud, as the company already uses Amazon Web Services (AWS) to stand up to the test and development environments used to build and update its mobile applications.
Knott describes the company’s overall cloud strategy as “evolving”, before suggesting the organisation is open to considering the merits of any of the major cloud platform providers, depending on how their technology fits with the business problem that needs addressing.
“We’re still early in our cloud adoption journey. We know cloud will be an increasingly important part of our products
“We will continue to work with AWS, and it just so happens we saw the Google data capabilities as being a better fit,” he says.
“Our cloud strategy is emerging, and we certainly see AWS and Google have strong capabilities to offer. We’re not doing any exclusive deals with anyone, but we will continue educating ourselves on cloud and adopt more of its products as it hits the market.”