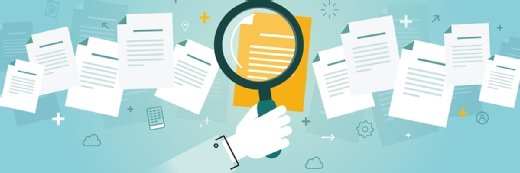
How JSR is using generative AI to drive insights
JSR Corporation has been using the Cohesity Gaia enterprise search assistant to surface insights from backups of employee mailboxes and OneDrive data
When Ryan Reed first joined JSR Corporation, a precision technology company with businesses in semiconductor material manufacturing and drug discovery, among others, he knew he had his work cut out for him.
The company’s data landscape was a patchwork of systems and repositories, spread across on-premise infrastructure and cloud environments. It was also dealing with large amounts of information from instrument readings to HR policy documents.
As the new IT leader, Reed’s top priority was to establish a robust, secure data management strategy that would not only protect JSR’s critical data, but also unlock its value for the business.
After evaluating several data protection and management solutions, Reed and his team landed on Cohesity. The platform’s approach to data security and access aligned with JSR’s needs, particularly its ability to provide immutable backups of instrument data.
“That was really critical because from a data integrity standpoint, as soon as we capture data from a lab instrument, we needed to have a backup and an immutable copy of the data,” Reed said. “One of the biggest advantages of Cohesity was the ability to start interacting with that data.”
But as Reed and his team dove deeper into Cohesity’s capabilities, they began to see even greater potential. The platform’s tight integration with cloud services like Microsoft Azure and Amazon Web Services (AWS), and its support for a wide range of data sources, meant JSR could start to unify its disparate data silos.
The game-changer came when Cohesity introduced its Gaia enterprise search assistant powered by the Azure OpenAI service. After an executive briefing on the new offering, Reed said JSR “jumped in pretty deep because as we try to digitise JSR, we want to put data at our people’s fingertips so they can make the best decisions”.
JSR became an early access customer for Gaia, working closely with the Cohesity team to test and refine the product. Reed’s team put Gaia through its paces, evaluating its performance on two use cases – e-discovery and surfacing insights from employee OneDrive accounts.
Reed said one of the things that he was impressed with was Gaia’s ability to understand context. “We had this test around ‘moonlighting’ – most companies address it in their HR policy handbook about doing a second job, but they don’t always call it moonlighting.
“We uploaded our employee handbook and Gaia came back with one of the best answers,” Reed said. “It understood the question and context, was able to return the answer in a very coherent way and cite where in the employee handbook the answer came from.”
Gaia’s ability to index and search Outlook mailboxes was another clincher for JSR, which often needs to retrieve information from employee mailboxes – even of those who had left the company – at the request of customers, particularly those in regulated industries.
Reed said compared to Microsoft Copilot, Gaia also does a better job of indexing an entire mailbox to surface content that may be older than a week, delivering a more comprehensive and contextual search experience.
Looking ahead, the company is planning to do the same for data hosted in other places, such as data lakes on AWS. “We want to be able to interact with that data using some of our models there, so we are going to remain multicloud and multi-LLM as we go forward,” Reed said.
As LLMs like those powering Gaia become more advanced, Reed is hoping to leverage those models to better interpret and extract insights from images that contain scientific data.
“We have enough images to do the training, but what we’d really like to do is to surface more of that data to different scientists in different areas without having to do a lot of training on multiple different images,” Reed said.
In addition, JSR is keen on leveraging Gaia to extract even more value from instrument data. “If you’re running an experiment with certain parameters, the LLM could help scientists accelerate the experiment by looking at potentially different variables,” Reed said.
Read more about AI in APAC
- Brunei’s Darussalam Assets is leveraging the AI capabilities in SAP SuccessFactors to generate job descriptions and optimise recruitment processes.
- Some 500 customer service officers at Singapore’s DBS Bank will soon be able to tap a GenAI-powered virtual assistant to improve workflows and better serve customers.
- Snowflake’s regional leader Sanjay Deshmukh outlines how the company is helping customers to tackle the security, skills and cost challenges of AI implementations.
- The Australian government is experimenting with AI use cases in a safe environment while it figures out ways to harness the technology to benefit citizens and businesses.