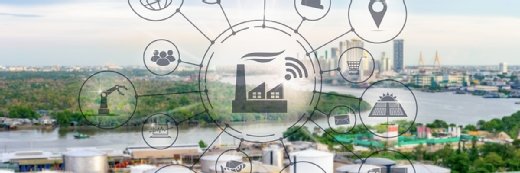
nirutft - stock.adobe.com
Edge computing implementations still in their infancy
It’s still early days for enterprise implementations of edge computing, with one analyst likening it to the early days of cloud
Even as edge computing continues to gain momentum among organisations that are looking to innovate outside the datacentre, enterprise implementations of the technology remain in their infancy.
That is according to Bob Gill, research vice-president in Gartner’s infrastructure strategies group, who compared edge computing today to the early days of cloud, when different groups within a business created their own cloud applications that might not adhere to corporate governance standards or have the ability to share data between them.
“We have the same problems today where people have been doing proofs of concepts in the edge world that are not based on any standard architecture, and so we have all these incompatible silos,” he said at Gartner’s 2022 IT Infrastructure, Operations & Cloud Strategies Conference in Asia-Pacific.
While Garter predicts that by 2023, 80% of enterprises will deploy multiple incompatible technology stacks due to the lack of architecture standards for edge computing, it is not tenable to reinvent the wheel for every emerging technology.
To that, Gill said organisations could adopt frameworks like the Linux Foundation’s LF Edge and EdgeX Foundry, or the Open Infrastructure Foundation’s StarlingX, which provide elements that they can use to build their edge technology stack.
IBM, for example, has taken an approach that combines elements of EdgeX Foundry and capabilities in IBM Cloud such as Cloud Pak for Data to develop a framework for its edge computing offerings, Gill said.
“Standards and tools will drive adoption at scale,” Gill added, noting that low-code development tools, for example, will enable business users and non-programmers to be more involved in building edge computing applications.
Edge security is another area that needs to be addressed over the next four to five years due to the larger attack surface brought about by a growing number of edge devices being deployed across the globe.
That is where emerging technologies and practices such as zero-trust security and SASE (secure access service edge) can come in to manage access to edge devices and ensure security all the way back to the cloud or datacentre, Gill said.
To address the demand for edge computing, public cloud providers have been offering hybrid cloud solutions such as Amazon Web Services (AWS) Greengrass and AWS Outposts which extend their cloud services to on-premise locations at the edge.
However, Gill said these offerings were restrictive “because what we’re really talking about is adhering to the line of a specific cloud provider for everything we do”.
“One of the things we’ve seen since the 1970s or 80s is trying not to be locked into a monolithic stack from top to bottom,” he said. “We want best-of-breed capabilities, and mixing, matching and optimising for our own specific situational context.”
One of the things that Gill has been working on is the idea of “edge-in” where organisations build edge applications that are optimised for low-latency and low-bandwidth autonomous connections, while tapping the cloud for machine learning and analytics.
“What we’re talking about with edge-in is not to eliminate the power of the cloud but having the ability to augment the cloud and getting the best of both the edge world and the cloud,” Gill said.
“Just as people are moving towards multicloud, one of the things we’re seeing with edge implementations is they want to be multicloud as well and use Google Cloud and AWS or Microsoft Azure on the backend – and a cloud independent platform at the edge itself,” he added.
Meanwhile, intelligent capabilities at the edge will improve, with machine learning inferencing expected to account for over 60% of edge use cases while machine learning training at the edge will be in 20% of use cases by 2027, according to Gartner.
“The disparity is due to the fact that training takes a great deal of computational horsepower, but we’re advancing so rapidly in machine learning training models that some of this will be enabled at the edge,” Gill said.
Read more about edge computing in APAC
- The move to the edge expands an organisation’s attack surface. Here are some measures that APAC organisations can take to minimise their edge security risks.
- Singtel’s new software platform is touted to make it easier for enterprises to deploy edge computing applications on its multi-access edge computing platform.
- Pilot projects are under way in the Asia-Pacific to deploy telco edge infrastructure to support high-bandwidth, low-latency applications.
- VMware is eyeing growing opportunities in India around cloud-native application development and edge computing, says VMware president Sumit Dhawan.