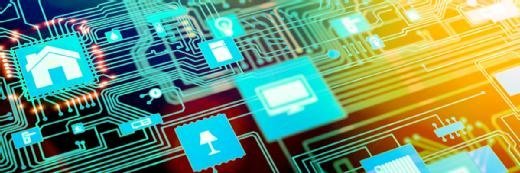
AA+W - Fotolia
Internet of things challenges in storage and data
The internet of things (IoT) brings challenges, with a host of new edge devices and data. We look at edge and core processing, compliance and information lifecycle management in IoT
The internet of things (IoT) has been touted as the next challenge for enterprises to adopt and exploit. But what exactly is IoT and how does it affect storage and the way we manage our data?
IoT refers to a broad network of physical devices that include sensors, vehicles, mobile devices and even home appliances that create and share data.
For enterprises this can mean cameras that monitor footfall, servers that run plant machinery, data collected from remote/branch offices or any location in which the business operates.
The breadth of options for IoT means that almost any device outside the datacentre that generates useful information could be part of an IoT solution.
Typically, IoT devices are seen as individual, remotely managed and embedded appliances such as cameras, but this isn’t always the case. Many businesses have distributed environments that run one or more servers at branch locations to monitor building access, environmental controls or other tasks that relate directly to the business itself.
As a result, IoT is a mesh of devices that could create, store and process content across many physical locations.
Distributed data and IoT
Probably the most obvious statement here is that the information created is outside the datacentre.
We are increasingly seeing the term “edge” used to describe computing and data management tasks performed outside core datacentres. Although edge computing has existed for many years, the current evolution in IoT and edge computing is notable for the sheer volume of data created in non-core datacentre locations.
This brings unique challenges to IT departments that must ensure this data is adequately secured, collated and processed.
Most IT organisations are used to knowing exactly where their data resides. With IoT, the challenge of putting arms around all of the content owned by a business is much greater, with obvious implications on user privacy and regulations, such as the General Data Protection Regulation (GDPR).
Distributed processing
With the possibility of so much information being created at the edge, it’s impossible to move the data into the datacentre for processing in a timely fashion.
First, with a wide variety of devices deployed it may be simply impossible for a business to move the data into the datacentre without investing heavily in external networking.
Second, in many instances the value of the data may not be best served by storing the entire content. For example, a camera that counts cars passing a traffic intersection doesn’t need to store the entire video, just report back the number of cars counted over specific time periods. The video data could be moved back at some time in the future or simply discarded.
A third point to consider is the timely processing of data. IoT devices may need to make local processing decisions quickly and not tolerate the latency of reading and writing the data into a core datacentre for processing to occur.
This distributed data and processing requirement means that businesses need to add the capability to push compute and applications to the edge and, in many cases, pre-process data before it is uploaded to the core datacentre for long-term processing.
The IoT information lifecycle
This brings us neatly to the subject of information lifecycle management (ILM).
ILM has been a broad aspiration of IT organisations for more than 30 years. Initially, this meant having the ability to move data between tiers of storage as the content aged and became less valuable. Eventually, data would end up in an archive or on tape.
In the modern enterprise, ILM is much more nuanced than it used to be.
As we’ve discussed, data is created at the edge and potentially pre-processed by in-situ edge computing devices. Over time, the data can be consolidated into core locations for further processing.
Businesses are increasingly starting to focus on getting additional value from all the data in the organisation by using artificial intelligence (AI) and machine learning (ML) techniques. AI/ML systems require huge quantities of data to train models and develop algorithms that in turn can be pushed back out to the edge as part of the pre-processing of data.
In this sense, ILM doesn’t look to directly optimise the cost of storing data, but instead to ensure it can be placed in the right location for the processing needed at the time. We’re starting to see the flow of information from the edge into core locations that continue to derive value long after the data was initially created.
IoT and public cloud
IoT data is mostly unstructured and so can easily be stored in public cloud infrastructure.
All the major cloud providers offer low-cost scalable storage systems based on object storage technology. With high-speed networks and no charge for data ingress, public cloud is a great location to store the volumes of IoT data being generated by businesses.
But, public cloud has more to give. Cloud service providers have extended their product offerings to include big data analysis tools that ingest and process large volumes of unstructured content. This allows businesses to create highly scalable ML/AI applications to process data potentially more efficiently than could be achieved in a private datacentre.
Supplier solutions in IoT
Looking at what suppliers are developing, we see a range of products and solutions. Here are some examples of how the requirements of IoT and storage are being addressed.
Some startup companies are developing in-situ processing storage devices that allow data to be analysed at the edge.
NGD Systems, for example, offers a range of “computational storage” products that look like traditional NVMe SSDs, but that also allow application code to run within the drive.
Meanwhile, ScaleFlux offers similar technology that can offload common tasks (such as erasure coding, database acceleration) to the storage device.
Amazon Web Services (AWS) provides the capability to import edge data into AWS S3 using Snowball. A Snowball appliance is effectively a ruggedised server with storage that can be used to physically transport data from an offsite location. AWS has further extended the capability of Snowball with Snowball Edge, which allows local data processing either with EC2 instances or Lambda functions.
Pure Storage, NetApp and DDN have all developed converged infrastructure or hardware reference architectures to use storage to support on-premise ML/AI systems. In these instances, the storage hardware provides the ability to process large volumes of data in parallel at extremely low latency.
Microsoft is working on project Brainwave, custom hardware to process data in real time as it is ingested from external sources. This is driving a move towards real-time AI processing.
Google already offers services in Google Cloud Platform (GCP) to process large data sets and is now looking at addressing the technology towards industry verticals. Still in early access development, Google is working on custom ASIC hardware that can be deployed at the edge to do initial ML/AI data processing.
Storage software startups such as WekaIO, E8 Storage and Excelero have developed products that provide scalable file and block storage for low latency analytics requirements. In the case of WekaIO, the software can also be installed on the public cloud (AWS) to create a highly scalable storage platform that uses NVMe storage.
StorMagic, a UK-based company, provides the capability to deploy scalable and resilient storage at the edge using SvSAN. The company has thousands of deployments of SvSAN running on standard hypervisors at edge locations such as wind farms and retail outlets.
HCP from Hitachi Vantara can be used as a centralised object store and archive for IoT data. Tools such as Hitachi’s Pentaho platform then visualise this data, making it easier to build data pipelines for use by the business to create value from disparate content stores.
IoT challenges
What becomes obvious when looking at the range of storage solutions on offer is the lack of standardisation in the market today.
There are no clear best practices or industry standards to ensure distributed data is securely accessed and transported into core datacentres. Data is typically moved in an asynchronous fashion, which risks it becoming inconsistent or out of step with copies in the datacentre.
As we move forward, the challenge for data storage and data management companies is to develop standards and tools that treat data outside the datacentre with the same level of security and consistency as within our public and private clouds.
Read more about the internet of things
- We hear from IT leaders who are early adopters of internet of things technologies about how to deliver on the potential of IoT.
- IoT brings huge opportunities, but how can organisations take full advantage? The latest CW500 Club looked at how to create the right environment for success.