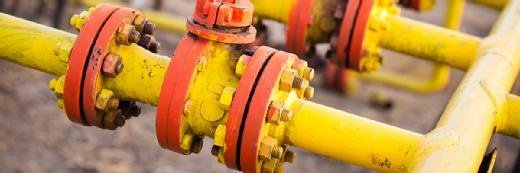
zorandim75 - Fotolia
How machine learning is applied in industrial IoT
GE Digital calls for the need to build industrial machine learning systems that are cognizant of the effects of a good and bad answer
Machine learning can have a greater impact on people’s lives when applied to the industrial internet of things (IoT) than in consumer applications, according to a machine learning expert at GE Digital.
Speaking at the Strata Data Conference in Singapore earlier in December, Joshua Bloom, vice-president of data and analytics at GE Digital, described the industrial IoT as the “internet of really important stuff, the objects and machines that power our lives”.
Bloom noted that when applied to the industrial IoT, machine learning can enable organisations to identify when an object should be replaced before it fails, or in the case of healthcare, help clinicians make sense of massive amounts of data in computer-assisted diagnosis.
Bloom said with 50 billion industrial IoT devices expected to be deployed by 2020, the volume of data generated through those devices will also balloon to 600 zettabytes per year.
“A single jet engine that GE creates produces about a terabyte of data in five hours,” he said. “That’s an unfathomable amount of data coming from just one engine and with 50,000 flights a day, you’d realise the scale of the data that we’re starting to deal with.”
In highlighting the impact of the successes and failures of industrial machine learning models, Bloom said a true positive could mean identifying a cancerous tumour before it was too late. “And if you have a false negative, it means you have a crack in a pipe that your system missed,” he said.
Bloom said organisations must also get comfortable with having more false positives. “If you can live in a world with lots of false positives, you’ll do better than if you had no machine learning at all,” he added, noting that this will ensure an “abundance of caution” built into machine learning models used in industrial applications.
Unlike data-driven models that may contain biases, industrial machine learning is grounded in the laws of physics. “We’ve been able to build physical models to capture our understanding of devices and simulate things that haven’t happened yet in the real world,” Bloom said.
However, Bloom conceded that there are some drawbacks in industrial machine learning. For one, physical models do not easily learn from new data, and not all physical laws have been built into large complex systems.
“That means we have an imperfect model, and we just hope that the imperfections are not fatal,” he said, adding that refinements to industrial machine learning models are not easy to make either, since experts with doctoral degrees are often needed in specialised fields such as metallurgy.
Read more about machine learning and IoT
- With its expanded Leonardo platform that includes machine learning capabilities, SAP hopes to go beyond its stronghold of supplying core ERP systems.
- Interest in implementing a cloud machine learning platform is up, but not every business is sold on the benefits of this emerging class of software.
- Artificial intelligence in various forms is not a panacea for every problem, but it can help enterprises fend off cyber attacks and get better at what they do.
- Businesses across the APAC region have started to dip their toes into AI and IoT to improve operations and stay competitive.
A data-driven model, however, does not require explicit understanding of the physical world, Bloom said. “You just throw in lots of data and optimise the model based on metrics that you’ve set up. It will naturally improve as you throw more data at it, and it’s extensible to more data sources.”
That said, Bloom noted that there is uncertainty over whether data-driven models can be generalised to other similar systems. “It’s also difficult to derive real physical intuition for those models,” he added.
At GE, work is underway to combine physical and data-driven machine learning models in its systems, and to ensure that the information generated from those models is trusted and understood by users. “Even if the models are correct, if people don’t trust and accept them, they are not going to wind up being used,” Bloom said.
“In the end, we need to create not just better algorithms, but also make machine learning suggestions understood to people with domain expertise. We also need to build systems that take in feedback, and are cognizant of the end user and the effects of a good and bad answer.”