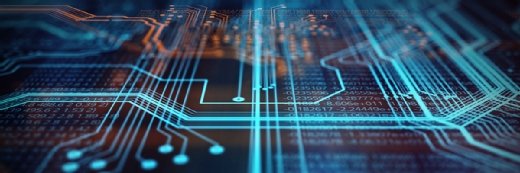
spainter_vfx - stock.adobe.com
Will computational storage meet data processing demands?
Computational storage promises performance for workloads that can benefit from dedicated oomph, but it’s not a cure-all. It may come at a cost, and with added application complexity
Data storage and data processing have always been separate functions, but what if they could be unified and achieve much better performance? That’s the promise of computational storage.
Although media have changed and capacity has grown, the core functionality of data storage has remained unchanged for decades. The magnetic disk drives and tapes of the 1980s often fulfil the same function as flash storage today, and in more or less the same architecture. However, computational storage is set to redefine how we approach storage and data processing. So, what are its potential benefits and challenges?
Data processing typically involves data movement in small batches across the input/output (I/O) bridge from storage device to processor, before writes back to storage. However, the I/O channel is typically slower than data transfer speeds that can be achieved closer to the storage. This slows down the rate at which data can be handled and results in bottlenecks.
This lag in data processing can inhibit real-time operations. By the time data has been processed, the crucial moment may have passed. When this occurs in time-sensitive environments, money can be lost and operational difficulties may arise.
Computational storage incorporates processing capability into the storage system. It’s akin to a mini server built directly into a hard drive. This means data no longer needs to move to processors dedicated to compute, because processing power has been attached to the storage system. It’s only been since the advent of solid-state drives (SSDs) that this has been possible.
This allows data to be processed far more quickly than ever before. Since data is processed in situ, computational storage is ideal for workloads that process massive amounts of data. The subsequent reduction of core processing power enables systems to operate more efficiently and reduce energy consumption for processors and associated cooling systems.
An additional potential benefit of computational storage is the reduction of network traffic, because some data can be processed on the storage device itself rather than being sent to the processor.
Types of computational storage
Currently, there are two different types of computational storage:
- Fixed computational storage services or fixed function devices have an onboard processor with a dedicated function (such as data compression) pre-programmed into them. Although they are easier to set up and install, they are function-specific and lack flexibility. One example of a fixed-function device is the SSD by Flexxon that monitors disk access for potential malware attacks.
- Programmable computational storage services or general-purpose devices are computational storage devices with an operating system that is typically Linux-based and needs to be programmed like a standard server. These have an on-board processor that can be programmed to perform specific data processing tasks. While general-purpose devices are far more suitable to meet specific business needs, they naturally take longer to set up.
Challenges remain
Despite the potential benefits of computational storage, there remain significant challenges to be addressed. The underlying technology of computational storage is in its infancy, and it’s currently only available from a limited number of manufacturers.
While SSDs are interchangeable and can easily interface with each other, this interoperability is lost with computational storage. Each supplier’s approach to computational storage is sufficiently different that interchangeability is not yet currently available.
As recently as late 2022, the Storage Networking Industry Association (SNIA) released new hardware and software architectural standards, as well as a preliminary standard for the application programming interface needed to access computational storage devices.
The potential security risks of computational storage are also not yet fully understood. The implications of potential threats and the security requirements of having a processor built into the storage device are yet to be fully considered.
To take full advantage of computational storage, existing applications and services may need to be re-factored to integrate with the new systems. This could make it difficult to use computational storage with existing applications, and development teams will need a thorough understanding of potential pitfalls. Modifying code always carries the risk of unintended consequences that some organisations may be unwilling to chance.
The question remains whether applications will be adapted to use computational storage in the future. If they are, then computational storage could reduce processing time. However, whether applications will be written to take advantage of on-board processing is dependent on how widespread computational storage becomes.
Use cases
Computational storage will not be a universal performance cure-all, as a single computational device will only offer performance gains in specific areas and could be costly. However, the onboard processors of computational storage make them ideal for specific data-intensive processing tasks. Some examples include real-time data analysis, machine learning and video compression for content distribution networks.
Given the limited processing power available to computational storage, tasks that are compute-intensive, such as modelling complex simulations, remain best performed by a dedicated processor.
Some of the companies that have already adopted computational storage include Tesla, Google, Facebook and Yahoo Mail. Some large-scale datacentres that offer processing resources, such as AWS and Alibaba, have also adopted computational storage.
Read more on computational storage
- Computational storage SSD options vary in benefit level. Computational storage SSDs come in two major formats: programmable and fixed-function. They have stark differences in feature set and market acceptance.
- Computational storage: What is it? Why now, what for, who from? Computational storage is an emerging architecture category, in which compute is put near storage to address I/O bottlenecks resulting from large volumes of data.
Computational storage devices are already commercially available, and any system equipped with this new technology will potentially be less CPU-intensive than conventional architectures.
With data-intensive tasks performed by the computational storage processor, the system processor can focus on compute-intensive segments of the workload. Managing a system in such a way increases overall performance, and allows tasks to be conducted faster and more efficiently. This would also enable time-critical workloads to become more viable.