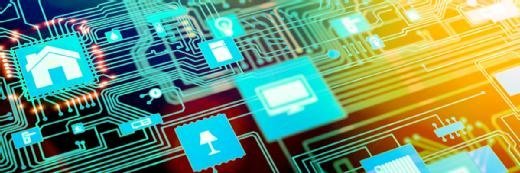
AA+W - Fotolia
Computational storage: What is it? Why now, what for, who from?
Computational storage is an emerging architecture category, in which compute is put near storage to address I/O bottlenecks resulting from large volumes of data
Handling data at the network edge is not a new idea, but it is becoming more important as organisations grapple with growing data volumes and the need to process information quickly.
Computational storage is, however, a relatively new way to tackle that challenge.
A key driver here is that conventional IT systems, with separate compute, networking and storage components, come with inherent bottlenecks.
One option is hyper-converged infrastructure (HCI), where processing, storage and networking are integrated into nodes that can be built into clusters.
Computational storage goes a step further, and puts processing onto the storage sub-system itself. This, its advocates say, offers far greater efficiency when data growth comes from the proliferation of sensors and the internet of things (IoT), or needs rapid processing for artificial intelligence (AI) and machine learning (ML) use cases.
However, the technology is still relatively immature, with only a handful of suppliers offering computational storage hardware, although a larger number are part of the Storage Network Industry Association’s (SNIA) working group.
What drives the need for computational storage?
According to the SNIA, “computational storage solutions typically target applications where the demand to process ever-growing storage workloads is outpacing traditional compute server architectures”. The industry body cites AI, big data, content delivery and ML as such workloads.
Other applications include encryption and decryption, data compression and deduplication, and storage management.
Data growth is certainly a driver. However, the push doesn’t just come from increasing data volumes, but the need to speed up processing and reduce overheads from repetitive tasks.
“We are seeing a gradual increase in capabilities – it’s not revolution, but evolutionary changes,” says Andrew Larssen, an IT transformation expert at PA Consulting. “As it becomes more mainstream, we’ll see the technology being used more widely, in pre-processing, compression, encryption and deduplication, or searching data, rather than having to load the data into a CPU.”
On paper, a system equipped with computational storage is less CPU- and energy-intensive than conventional architectures.
This helps at the network edge – where local processing can filter data before sending it on to a conventional server – but also in the datacentre, where computational storage modules take some workloads from the CPU.
In a conventional server-storage architecture, the CPU requests data, performs the task and sends data back to storage. In a computational storage model, the CPU sends a task, such as decryption, to the “intelligent” storage sub-system. Security is a further benefit, as the data need never leave the drive.
Technologies and deployment options
Computational storage is enabled by technologies that include high-performance solid-state media, low-cost programmable arrays or processing cores, and efficient interfaces.
The current generation of computational storage systems use flash memory, although, as processing and storage are separate, any sufficiently high-performance storage technology could work.
The system itself is typically a U.2 or M.2 NVMe drive or a PCIe card. Some suppliers also offer EDSFF. The exception is Samsung’s SmartSSD which, as the name suggests, is based on a standard 2.5in SSD mechanism. SNIA’s roadmap favours NVMe for CSDs (computational storage drives).
To provide the compute capability, suppliers either use field-programmable gate arrays (FPGAs) or ARM-based system-on-chips (SoCs).
FPGA technology is currently more widespread. IT departments can buy a “fixed” FPGA system, with specific functionality programmed in, typically common storage management functions. Custom or programmable systems allow users to add their own functions, via low-level FPGA languages, or a high-level programming language such as Xilinx Vitis.
FPGAs cannot be programmed on the fly, however. This has prompted some suppliers to use ARM core SoCs instead. These can run Linux, and have the potential to expand the capability of computational storage technology even further.
Read more on computational storage
- Putting container technology doesn’t feature in a hard drive? That’s being explored in one of the Storage Networking Industry Association technical working groups under the title of computational storage.
- How the computational storage drive is changing computing. Take a closer look at computational storage technology and products, and how localised computation is changing storage architecture and the compute-storage dynamic.
For example, the SNIA anticipates suppliers adding Ethernet or other networking to allow peer-to-peer communication between computational storage devices.
So far, though, only one supplier, NGD, is making ARM-based computational storage devices.
Because there are so many applications for computational storage, the market for the technology is not homogenous. Instead, suppliers are developing devices tailored by application, by interface, and by the way they are programmed and managed.
Some suppliers have focused on dedicated sub-systems, while others position computational storage as a potential upgrade to existing storage hardware.
The industry currently defines computational storage devices as: computational storage drives (CSDs), which are storage with computational services added; computational storage processors (CSPs), which is hardware with no storage of its own but which brings processing to a storage array; and computational storage arrays (CSAs), which provide multiple CSDs or ordinary drives with a CSP added for compute.
SNIA also defines computational storage services (CSS) as the services layer that handles discovery, operation and, potentially, programming of computational storage devices.
Use cases for computational storage
Computational storage lends itself to any application where moving data from storage to the CPU is the bottleneck. This suggests data-intensive, rather than compute-intensive, uses. The processing power available in an FPGA or SoC is limited.
And with few suppliers offering, for now at least, SoC-based systems that can run a full operating system (OS), fixed CSDs lend themselves to pre-determined, fixed workloads such as deduplication, storage management or encryption.
Programmable CSDs are finding a place in AI and business intelligence (BI) applications, as are SoC-based systems. They can also be used for database acceleration.
Only SoC-based systems can be loaded with code directly by a CPU, and even there, this means cross-compiling code from X86 to ARM. But this gives users the greatest flexibility, allowing applications to move processing onto the CSDs as needed.
If CSDs get their own native networking, they could also share tasks directly and take further loads off CPUs, or allow more complex work which might be too great for a single CSD to be done at the edge.
“Storage is cheap at the moment, and there are applications with a lot of repetition, especially when you move to ‘data lake’ quantities,” says PA Consulting’s Larssen. “So if you can do compression and deduplication [in the storage layer], you will see big gains from it.”
Larssen expects to see uptake of computational storage by streaming services, for video compression and content distribution networks (CDNs). He also predicts growth in database accelerators, for Postgres and MySQL.
“The technology is still niche,” he says. “Unless it is fundamental to your business, you’re probably not going to deploy it. It might be cheaper to double the amount of general compute you are using. When that is unachievable, that is when you need to think outside the box.”
Computational storage suppliers
Pliops markets its Storage Processor as a hardware accelerator for databases and other data-intensive workloads. It is focused on Postgres and MySQL. The supplier offers compute and storage node acceleration, using NVMe-based technology.
Samsung offers its Smart SSD, which uses a Xilinx FPGA chip. The technology is available via Samsung or Xilinx. Samsung is currently positioning the technology for BI, financial portfolio intelligence, and for the aviation industry.
NGD is currently the only supplier to use SoC that can run an OS to power its computational storage. It says its system is Nand flash agnostic and runs 64-bit Linux.
Eideticom offers its NoLoad computational storage processor. It is claimed to be the first NVMe CSP, and is targeted at datacentre infrastructure acceleration and scientific research, as well as general cost reduction.
Scaleflux offers AIC and U.2 drives. Its CSD 2000 Series supports up to 8TB (terabytes) of 3D Nand flash memory, with data path compression and decompression.