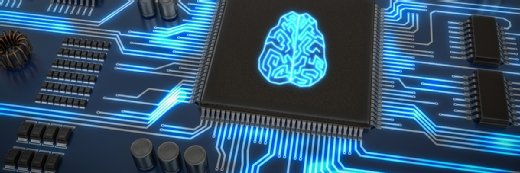
vchalup - stock.adobe.com
Grilling the answers: How businesses need to show how AI decides
As artificial intelligence becomes more widespread, so the need to render it explainable increases. How can companies navigate the technical and ethical challenges?
Show your working: generations of mathematics students have grown up with this mantra. Getting the right answer is not enough. To get top marks, students must demonstrate how they got there. Now, machines need to do the same.
As artificial intelligence (AI) is used to make decisions affecting employment, finance or justice, as opposed to which film a consumer might want to watch next, the public will insist it explains its working.
Sheffield University professor of AI and robotics Noel Sharkey drove home the point when he told The Guardian that decisions based on machine learning could not be trusted because they were so “infected with biases”.
Sharkey called for an end to the application of machine learning to life-changing decisions until they could be proven safe in the same way that drugs are introduced into healthcare.
And the IT industry is waking up to the threat to the next big wave of spending.
Although he does not use the same language as Sharkey, Patrick Hall, senior director for data science products at machine learning tools company H2O.ai, says decisions that cannot be explained will feel very “icky” to consumers.
“Companies are starting to be aware that they need to create explainable AI to satisfy human curiosity,” he says. “We are trying to get business adoption of this cool, new, very powerful technology and are trying to prevent this icky ‘computer says no’ feeling.”
In a study based on interviews with 4,400 consumers, Capgemini found that their views on ethics and AI threaten both company reputation and the bottom line – 41% said they would complain in case an AI interaction resulted in ethical issues, 36% would demand an explanation, and 34% would stop interacting with the company.
The results show that although machine learning ethics and explainability are separate issues, they are linked, says Hall.
“The way to test for bias in data and machine learning models is a fairly well-known process called disparate impact analysis, which is different, technically, from explainable AI,” he says. “They certainly do go together, but I would never use explainable AI as my front-line, fairness testing tool.”
To help organisations explain their machine learning decision-making, H20.ai has created a set of tools that provides companies with dashboards to explain the results of both their own driverless AI models and models built through other processes (see box below).
Explainable AI: a limited glossary of terms
Popular machine learning development environment H2O Driverless AI employs a number of techniques to help explain the workings of machine learning. These include:
- LIME (local interpretable model-agnostic explanations): The technique attempts to understand the model by altering the input of data samples and understanding how the predictions change.
- Shapley values use game theory to assign importance to machine learning features indicating which is likely to lead to a decision.
- Partial dependence describes the marginal impact of a feature on model prediction, holding other features in the model constant.
In August 2019, IBM launched a set of tools designed for a similar purpose. AI Explainability 360, the company says, is a “comprehensive open source toolkit of state-of-the-art algorithms that support the interpretability and explainability of machine learning models”. IBM is inviting the open source community to contribute to expanding it.
Saska Mojsilovic, an IBM fellow focused on AI, says businesses will have to adopt explainable AI because they need to get consumers to trust the machine learning model they are adopting with increasing frequency.
“It became very obvious that if you are going to be using these machine learning algorithms to inform, or guide some really important decisions in our lives, then you really need to have this confidence or trust,” she says.
But explaining machine learning decision-making to a data scientist is one thing; explaining it to consumers or the public will require a great deal more creative thinking, says Mojsilovic.
“Fairness may be a complex ethical issue, but in a way, explainability is even more difficult,” she says. “Think about how humans explain things, how we navigate the world around us and how we communicate. We do it in so many different ways. We look for examples and counterexamples and summarise things, and so on. We thought about how to take that expressiveness of human interaction and create the methods to communicate [the way AI reaches conclusions].
“There are these ways to get to an explanation. So, over the last year or a year and a half, we created several models that employ these different modes of human explanation.”
For example, IBM has created a model called ProtoDash that explains the results of AI using prototypes – examples of the kinds of scenario that drive predictions. Meanwhile, a model called Boolean decision rules generates sets of rules that humans find they can interpret, a method that won the inaugural FICO Explainable Machine Learning Challenge.
Lastly, there is an approach that relies on the concept of “contrasting explanation” which tries to pick out things that are missing.
“Doctors, for example, tend to diagnose patients as much on symptoms that are not present as ones that are,” says Mojsilovic. “If something is missing, it is an important differentiator. Had it been there, the decision would have been vastly different.”
The governance imperative
But the challenge of creating AI decision-making that companies can explain is not only about the tools and technology, says Sofia Ihsan, trusted AI lead at global consultancy EY.
“When I’m doing some of my work, I’m often in a room for PhDs – some really clever data scientists,” she says. “If you look at what motivates them, it’s about accuracy – explainability isn’t something they are thinking about. At least, it’s not their primary consideration. When they do think about it, they might think it is a limiting factor and they don’t want to be limited.”
So, creating an overall governance structure that includes explainability in the AI process from the outset is a struggle for many organisations, says Ihsan. “When you think about training data, that’s right at the beginning of the lifecycle of development.”
Explainability needs to start at the beginning, she says. “It’s not about coming in after the event and trying to put controls and assurance in place. It is about identifying and managing risk throughout the lifecycle of development and monitoring them while models are in use to make sure that they are working in the way you expect them to work.”
Such is the growing public interest in the fairness of AI decision-making, building in explainability from the start will come under the umbrella of corporate social responsibility, says Ihsan.
“What is the impact on society, on mental or physical wellbeing, and the environment?” she says. “The public is generally getting more savvy. This is going to come in from a brand perspective. People not only want to know that they’re being treated fairly as individuals, but also more broadly, that things are fair and unbiased.”
Read more about explainabilty in AI
- What is explainable AI (XAI)?
- The answer to the ultimate question of life, the universe and everything is 42, according to Deep Thought in The Hitchhiker’s Guide to the Galaxy – but experts need to explain AI decisions.
- Bosses want to see explainable AI.
But for AI to be accepted on ethical grounds, it will require more than simply explaining the reason behind machine learning decisions, says Rachel Thomas, director of the University of San Francisco’s Center for Applied Data Ethics.
“When AI makes decisions that really impact people’s lives, then not having an explanation is incredibly frustrating,” she says. “But an explanation alone is not sufficient. There needs to be some sort of system for recourse as well, such as the ability to appeal decisions.”
The difficulty of building explainable AI from the start, and offering a justification for decision-making when challenged, is tempting some organisations to skip some of these processes, says Thomas.
“It’s called ‘fair-washing’, where people take an unfair system and post-hoc give a fairer justification for the decisions they have made,” she says. “If somebody misses out on a loan because of their gender, and then you could go back later and say, ‘oh no, this is because of their credit score’. You can always find an explanation that is less suspect. It is another reason why explainability, in itself, won’t be sufficient [to create ethical AI].”
Some organisation have promised AI that helps with hiring decisions or predicting crime, but Thomas warns businesses against the blanket adoption of AI in all use cases.
“Organisations need to think about their particular use case and not see AI as a kind of magical entity that is making everything better,” she says. “Yes, we’ve made concrete advances in certain areas, but there are other areas where we have not at all. The whole idea of the premise of trying to predict what a person is going to do in the future is very dubious.”
As the popularity of AI spreads, so does public concern about its impact. Only AIs that can explain their decisions in a way people can understand and accept will create long-term value for the organisations that create them.