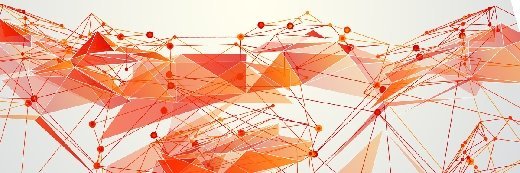
Fotolia
Explainable AI: How and why did the AI say ‘true’?
The answer to the ultimate question of life, the universe and everything is 42, according to Deep Thought in ‘The Hitchhiker’s Guide to the Galaxy’ – but experts need to explain AI decisions
Artificial intelligence (AI) is expanding beyond academics and the web giants, who have immense access to data and computing power and deep pockets to fund research projects. There is a lot of hype, but companies are being urged to embrace AI.
Two studies published recently have emphasised the importance of businesses adopting AI to remain competitive.
The Let’s get real about AI study by management consulting company OC&C reported that spending on AI has been huge: $219bn was spent by businesses on AI globally in 2018, equivalent to about 7% of the total enterprise IT spend. AI spend in the US was $91bn in 2018, and $12bn in the UK.
But OC&C warned that one of the key challenges in using AI is building trust in the answer. AI systems typically learn “the rules” from exposure to outcomes rather than building up from simple rules, such as “if x, then y”. This means that the AI system may not be able to explain why a particular result was achieved. In turn, this can cause serious problems with trust in AI infrastructure and rejection of human operators, and/or fundamental problems with conforming to regulations, OC&C warned.
Similarly, Gartner’s Trends 2019 report, published in January 2019, forecast that through to 2023, computational resources used in AI will increase five times from the levels in 2018, making AI the top category of workloads driving infrastructure decisions.
Gartner’s research predicted that people increasingly rely on the outcome of AI solutions. By 2022, 30% of consumers in mature markets will rely on AI to decide what they eat, what they wear or where they live, said the analyst firm.
However, Gartner noted that issues related to lack of governance and unintended consequences will inhibit the success of AI investments and will slow down adoption. The levels of explainability and transparency of the deployment and function are crucial to the accuracy and trustworthiness or effectiveness of AI in IT work.
Explainable AI has business benefits
Last year, the unfortunate story of a woman in Arizona who was mown down by an autonomous Uber car was widely reported. At the time, anonymous sources claimed the car’s software registered an object in front of the vehicle, but treated it in the same way it would a plastic bag or tumbleweed carried on the wind.
In the recent IEEE paper, Peeking inside the black box, the authors, computer scientists Amina Adadi and Mohammed Berrada, wrote that only an explainable system can clarify the ambiguous circumstances of such situation and eventually prevent it from happening. Adadi and Berrada concluded that explainability in AI could become a competitive differentiator in business.
In a recent popup event hosted by Domino Data Lab, Tola Alade, a data scientist at Lloyds Banking Group, said: “Loads of organisations are using AI to make decisions. But it is becoming important for organisations to explain the decisions of AI.”
In some regulated industries, companies have to provide an explanation of their decisions. So if an AI is involved in the decision-making process, the data the AI used to obtain its decision, and the weightings it associated to each element of data, needs to be measurable.
Supervised learning
Decisions are learnt in one of two ways – supervised and unsupervised. As its name suggests, unsupervised machine learning involves letting the machine do all the work on its own. The AI is effectively a black box and, as such, it is far harder to debug and harder to understand how its decisions are being made, compared to when a known dataset is used for training.
In supervised machine learning, pre-labelled input and output data is used to train an algorithm so that the AI can predict outputs from new inputs not previously seen. Because the pre-labelled data is known, the AI algorithm is said to be a white box.
XAI (explainable AI) aims to explain machine learning model predictions. Tools are starting to appear that create a prediction model to “understand” how white-box and black-box AIs make their decisions. These tools effectively use some clever maths based on the variation between a predicted result from an AI algorithm and an actual measured result.
Read more about explainable AI
- There is a mantra in technology that if a program is fed bad data, it will produce bad results. The same is true of AI. The quality of a machine learning application is directly related to the learning data used to train the AI model.
- Explainability has been touted as a solution to the problem of biased AI models, but experts say that approach only gets you part of the way to bias-free applications.
But in some application areas, even if an AI is trained on known data, it may not give the most optimal results. In a recent interview with Computer Weekly, Sébastien Boria, mechatronics and robotics technology leader at Airbus, said an AI system that takes a sample of all the people who work on a particular manual process to understand how they work, may well come up with a machine learning model based on the average behaviour of the people sampled. “Just because 50% of the population does something does not make it the right solution,” said Boria.
So along with XAI, in some circumstances, businesses may also need to assess the impact on an AI algorithm of source machine learning data that represents either an exceptionally good or exceptionally bad result. The bias associated with these two extremes must be carefully considered as part of an XAI model.
Going beyond the final answer
Explainability in XAI is more than just how the machine arrived at its answer. People also need to understand the “how” and the “why”, according to the authors of the IEEE Peeking inside the black box paper.
“It is not enough just to explain the model – the user has to understand it,” the authors said. However, even with an accurate explanation, developing such an understanding could require supplementary answers to questions that users are likely to have, such as what does “42” mean in The hitchhikers guide to the galaxy?