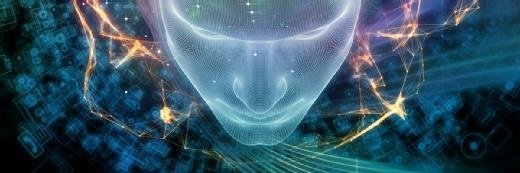
agsandrew - Fotolia
Automated decision making shows worrying signs of limitation
AI and data-driven decision making projects need to be handled with care – worrying limitations revealed in the law and healthcare
Data released by West Midlands Fire Service appears to show the city of Birmingham has too many fire stations, with 15 compared with neighbouring Solihull’s two. The service’s online map of attendance times shows many parts of Solihull, a suburban and rural area, have to wait much longer for firefighters to arrive. Even on the basis of relative population sizes, Solihull looks under-served.
But other data mapped by the service reveals why urban Birmingham has numerous stations: “If you look at where the incidents are, you’ll be able to see very clearly there’s a strong justification,” says Jason Davies, a data analyst for the service’s strategic hub. There are proportionally more fires in areas of Birmingham, including Aston, Handsworth, Ladywood and Highgate.
The service also uses data analysis to target fire safety advice, and has found correlations between high risks of accidental home fires and single-person households, social renting, unemployment, smoking and black and Afro-Caribbean ethnicity. Davies says some factors may directly contribute: unemployed people are more likely to be at home, making it more likely someone will accidentally start a fire.
However, “you have to be careful before you jump to conclusions,” says Davies. “The correlations alone are enough to inform our service delivery model. We don’t need to understand the exact causes.”
It’s not hard to make a case for using data to focus fire service resources on the households most at risk, particularly when that data is openly available. But many organisations use data to make decisions in a far less transparent way, and the increasing use of machine learning or artificial intelligence (AI), where computers use such data to adjust decision-making algorithms, has worried Sir Tim Berners-Lee, the inventor of the world wide web.
“When AI starts to make decisions such as who gets a mortgage, that’s a big one,” Berners-Lee told an event in April 2017, going on to imagine AI-based systems creating their own companies.
“So you have survival of the fittest going on between these AI companies until you reach the point where you wonder if it becomes possible to understand how to ensure they are being fair, and how do you describe to a computer what that means anyway?”
AI gives stiff sentence
Some people’s lives have already been severely affected by secret algorithms. In the US, in 2013, Eric Loomis was sentenced to six years in prison by a Wisconsin judge. Loomis pleaded guilty to eluding a police officer, but the issue was the length of his sentence, which the judge partly decided based on a “Compas score”.
Compas [Correctional Offender Management Profiling for Alternative Sanctions] scores assess the risk that someone will commit a further crime, using an algorithm developed by US criminal justice IT company Northpointe.
Northpointe did not release its workings when Loomis challenged the length of his sentence. The US Supreme Court recently declined to review the Wisconsin supreme court’s ruling in favour of Compas’ use in Loomis’ case, although the Electronic Privacy Information Centre is involved in several other legal challenges it calls a lack of algorithmic transparency. Many US states use similar algorithms, and Durham Constabulary in the UK is preparing to use a similar system to decide whether or not to release people arrested from custody.
Christopher Markou, a doctoral candidate at University of Cambridge’s law faculty, says the results of such algorithms can be beneficial in supporting human decisions – the problem is when they replace them. “I’m old fashioned in that I believe the justice system is a human system,” he says. “By trying to get systems like Compas to replicate portions of the justice system, there is an implicit concession that we are not good enough at this.”
There are particular problems when algorithms are secret: “That’s a pretty fundamental challenge to how we’ve thought the justice system has worked, which is equality of arms – you have to disclose your case proactively to the defence counsel so a robust defence can be mounted,” he says.
There is a further risk that algorithmic opinions are seen as untainted by human bias and therefore risky to challenge: “It’s easier to blame something else, other than yourself,” says Markou.
Read more about the limitations of AI
- Watson Analytics is a strong player in a formidable field that includes Google and Adobe. It does perform as advertised – but IBM's AI-driven analytics technology leaves a lot to be desired.
- Modern AI tools fall short of true artificial intelligence, and this could have implications for how the technology is used by enterprises in the near future.
The problem is that algorithmic decisions are very often based on an archive of human ones, along with all the biases that archive contains. US public interest publisher ProPublica last year analysed Compas risk assessments for 7,000 people arrested in Florida and found that as well as being “remarkably unreliable in forecasting violent crime” the scores wrongly rated black defendants as likely to become criminals almost twice as often as white ones. Northpointe disputed the analysis.
The legal world has a technophobic history: courts initially resisted the use of photocopying and a 1950s paper by a US law professor worried about ham radio hooking up prostitutes to truck drivers. However, there is something different about machine learning: “It can reprogramme itself, at least in deep learning applications,” he says. This means such a system could produce different results from the same data for no apparent reason.
Those managing projects that make decisions with data need to think about how to protect it, says Joanna Bryson, a cognitive scientist at the universities of Bath and Princeton. “People talk about data as the new oil,” she says. “It does have value, but oil is something you have to be careful with. It’s explosive – it’s something you don’t want to store everywhere and not think about.”
Bias in data-driven decisions
Bryson has researched bias in data-driven decisions and sees three main ways to tackle it. The first is to recognise that biases exist: “The reason machine learning is working so well is it is leveraging human culture. It’s getting the bad with the good,” she says. This may particularly affect data on decisions made over several decades, but only using recent data can increase random bias.
The second is to test data for biases in obvious areas such as ethnicity, location, age and gender. This may come more naturally to a diverse IT workforce as individuals are more likely to consider the impact on themselves, but Bryson says this is not guaranteed: “As a woman who used to be a programmer, you’re often absorbed into the dominant group you’re in.”
Third, Bryson says those using data and algorithms should get used to auditing and surveillance. “There may very well become bodies like the FDA for AI and tech more generally,” she says, referring to the US’s powerful Federal Drug Administration. The EU’s General Data Protection Regulation, which comes into force in May 2018 and which the UK looks set to retain after Brexit, includes a specific right to challenge automated decisions. “In advance of that, for your own benefit, you can make sure you have internal processes,” she says.
Such auditing can help board members and other managers to check what is going on. “A lot of programmers are sloppy,” says Bryson. “They’re used to not having to do a lot of tests, although big organisations have got better at expecting to do tests. But you do get people that are almost deliberately obfuscating – ‘if we’re using machine learning we don’t have to do these tests because no-one can check machine learning’. Well, that’s not true.”
Pneumonia less likely to carry off asthmatics?
An increasing number of experts are looking at how to make data-driven decisions, including those that involve machine learning, fairer. In the 1990s, Rich Caruana, then a graduate student at Carnegie Mellon University, worked on training a neural net machine learning system to predict the probability of death for pneumonia patients.
A parallel rule-based model came up with the surprising rule that patients with asthma were less likely to die from pneumonia as those without asthma. “You don’t need much background in healthcare to question whether that would make sense,” says Caruana, now a senior researcher at Microsoft Research.
The data was biased, but for good reasons: patients with asthma were more likely to see a doctor quickly for new breathing problems, doctors were more likely to take these seriously and hospitals were more likely to treat them urgently. The actions of patients and professionals, based on the medical reality that pneumonia is more dangerous for those with asthma, made the data suggest the reverse was true.
As a result, Caruana and his colleagues did not use the neural net, a black-box model which does not disclose why it makes the predictions it does. More recent research found this data similarly suggested that chest pain and heart disease patients were less vulnerable to pneumonia.
Identifying and adjusting for biases
Caruana has helped develop a generalised additive model known as GA2M that is as accurate as a neural net but allows users to see how predictions are made, allowing them to spot anomalies. “With this new kind of model, you absolutely include all the variables you are most terrified about,” he says, so biases can be identified and adjusted for.
This is a better option than removing variables, as bias is likely to affect correlated data as well. He will be discussing his work at the Fairness, Accountability and Transparency in Machine Learning event in Halifax in Canada on 14 August.
“Every complex dataset has these landmines buried in it,” says Caruana. “The most important thing, it turns out, is just knowing you have a problem.” In some applications, the problems will not matter: the pneumonia probability of death data would be fine for insurers looking to calculate survival rates. “The data is not right or wrong, the model hasn’t learnt something that’s right or wrong,” says Caruana – but applications of it can be.
Data can be used to make decisions well if processes are transparent, data is tested for problems and users recognise perfect, unbiased data is usually not available. In theory, a clinical trial could determine the true risk to asthma patients from pneumonia by sending half of them home to see if more of them died than those treated in hospital – but for obvious reasons, this would be unethical.
“It’s illegal to have the data you want, and it should be illegal,” says Caruana. That is why it makes sense to learn how to use the data you have.