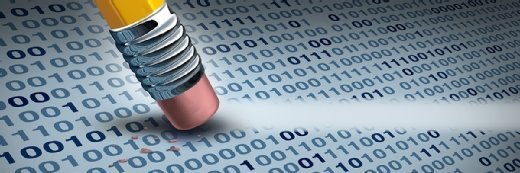
freshidea - stock.adobe.com
Dealing in data
Lessons learned from pandemic data reporting will help forge new analytics techniques to improve data quality
According to the World Economic Forum (WEF), the collection and misinterpretation of unreliable data can undermine trust in public health systems or the willingness to trust government generally.
The Covid-19 coronavirus pandemic has highlighted inadequacies in the collection, processing and interpretation of data. As the world’s population makes small steps on the journey to recovery, the lessons learned will help forge new data analytics techniques to improve data quality.
Inaccuracies in data collection
“We’ve seen lots of inaccuracies, inconsistencies and anomalies in the reporting of the data relating to Covid-19,” says Michael O’Connell, chief analytics officer at Tibco. “The pandemic has highlighted the need for sound data science, visual analytics and data management methods, and the infusion of these skills and literacy into broader groups of users – in companies and in the population at large.”
According to Stan Christiaens, co-founder and chief technology officer (CTO) at Collibra, which provides a cloud-based platform for building a data-driven culture, what the coronavirus pandemic has shown is that not all data is created equal and datasets are often incomplete.
“There is a lack of alignment among the players fighting the spread of the coronavirus about what is being measured and compared,” he says. “And all of that is contributing to uncertainty and inconsistency amid Covid-19, and that is compounding mistrust and fear.”
The challenge for researchers trying to combat the virus is that comparing the data they have available to them is often a bit like trying to compare apples to oranges, and there are discrepancies between countries.
“We’re all in this together,” he says. “Yet some countries are finger-pointing and telling others that their numbers related to coronavirus infection rates and fatalities are wrong.”
It all boils down to how people collect data and on what they base their measurements.
As Christiaens points out, there are many ways a country can account for the number of coronavirus fatalities. Officials may just count anyone who died with coronavirus-like symptoms. But unless a person was tested, it is unclear whether that individual succumbed to the virus directly. And even if a patient had the virus, that individual’s cause of death could have been due to coronavirus combined with something else.
This, for Christiaens, is a classic problem, but the coronavirus pandemic represents one of the first times the problem of recording deaths slightly differently has had a worldwide effect.
“Part of the solution involves those who are measuring cases to come together to identify the similarities and differences in their approaches,” he says. “That provides a fundamental layer of trust and alignment. If you don’t do this, it’s impossible to share numbers effectively. Everybody in accounting knows this. You have to keep what you’re comparing comparable.”
Christiaens believes the coronavirus pandemic has shown that not all data is created equal, and this applies not only to battling with a deadly virus, but also everyday business systems.
“In business, CRM [customer relationship management] systems often contain inaccurate data because they rely on salespeople typing in notes,” he says. “In the coronavirus fight, efforts that rely on Covid-19 self-reporting can create inaccurate data because people may not tell the truth or they might misinterpret signals.”
But machines also make mistakes. “You might also get inaccurate data coming from automated systems,” adds Christiaens.
“Say a country uses an automated system that connects with smartphones to check users’ temperatures. Maybe it’s warm where the person is and they are spending time in the sun, so that person’s temperature is elevated. Or maybe the person has symptoms that do not stem from the coronavirus. There are a hundred reasons why the measurements in automated solutions can display variability, leading to inaccurate data.”
Smoothing out data errors
Data science methodologies are key to dealing with case reporting and other data artefacts. To address the data reporting artefacts and inconsistencies, O’Connell says Tibco uses a “non-parametric regression estimator based on local regression with adaptive bandwidths”.
This technique – introduced by Jerome Friedman, professor emeritus of statistics at Stanford University – allows data scientists to fit a series of smooth curves across the data. It is called “super smoother”.
Read more about data quality
With the right data, individuals and organisations can make the most informed decisions to keep people safer through the coronavirus pandemic and restart the economy at the right time and place.
One of the biggest concerns that businesses have about their data is its quality, and quality of data is a governance issue.
“This is essential as, at the most basic level, people don’t report data well, such as the coronavirus infection rate on weekends, compared to weekdays. This is why we often see a spike on Mondays, or on days when an influx of test results arrive,” he says.
The super smoother technique fits a smooth curve to local regions of the data, which O’Connell says avoids chasing noise – a typical problem with many raw data presentations.
Data profiling
As well as using techniques to smooth out data discrepancies, data profiling tools can also be used to find incomplete data by identifying basic problems.
“They can spot that a dataset does not include the ages of patients, or that 70% of the ages are missing,” says Christiaens. “Perhaps these details are missing due to privacy laws. But if you’re going to build a model for Covid-19 that doesn’t include age information, particularly for the elderly, that model is going to be bullish compared to one that relies on datasets containing age details for the patients.”
His top tip for anyone looking at using such tools is to ensure they are programmed with relevant rules. “If you don’t, it could create problems,” he says. “For example, we all know there’s no such thing as a 200-year-old person or a minus-10-year-old person, but unless you set a rule for that, the data profiler will not know it.”
Reassess assumptions
Beyond the immediate challenges of accurately recording and modelling the infection rate and learning how other countries respond to the easing of lockdown measures, there are set to be numerous data science challenges as economies attempt to return to normal working patterns.
In a recent blog, Michael Berthold, CEO and co-founder of Knime, an open source data analytics company, wrote about how some existing data models were wholly inadequate at predicting business outcomes during the lockdown.
“Many of the models used for segmentation or forecasting started to fail when traffic and shopping patterns changed, supply chains were interrupted, borders were locked down, and the way people behaved changed fundamentally,” he wrote.
“Sometimes, the data science systems adapted reasonably quickly when the new data started to represent the new reality. In other cases, the new reality is so fundamentally different that the new data is not sufficient to train a new system, or worse, the base assumptions built into the system just don't hold anymore, so the entire process from data science creation to productionising must be revisited,” said Berthold.
A complete change of the underlying system requires both an update of the data science process itself and a revision of the assumptions that went into its design. “This requires a full new data science creation and productionisation cycle: understanding and incorporating business knowledge, exploring data sources and possibly replacing data that doesn't exist anymore,” he said.
In some cases, the base data remains valid, but some data required by the model is no longer available. If the missing data really represents a significant portion of the information that went into model construction, Berthold recommends that the data science team re-run the model selection and optimisation process. But in some cases, where only the missing data is partial, he says it may only be necessary to retrain the data model.
Data governance
Rachel Roumeliotis, vice-president of data and artificial intelligence (AI) at O’Reilly, points out that some common data quality issues point to larger, institutional problems.
“Disorganised data stores and lack of metadata is fundamentally a governance issue,” she says, adding that data governance is not a problem that is easy to solve and one that is likely to grow.
“Poor data quality controls at data entry is fundamentally where this problem originates – as any good data scientist knows, entry issues are persistent and widespread. Adding to this, practitioners may have little or no control over providers of third-party data, so missing data will always be an issue,” she adds.
According to Roumeliotis, data governance, like data quality, is fundamentally a socio-technical problem, and as much as machine learning and AI can help, the right people and processes need to be in place to truly make it happen.
“People and processes are almost always implicated in both the creation and the perpetuation of data quality issues,” she says, “so we need to start there.”