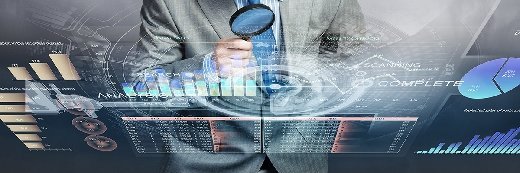
Sergey Nivens - Fotolia
Too many data sources and too little consistency
One of the biggest concerns that businesses have about their data is its quality, and quality of data is a governance issue
While we are consistently creating data and analysing it to use in our businesses, we often forget to reflect on the value of the data we are collecting. Don’t get me wrong – businesses are aware of the data quality issues throughout their organisations, but many face the dilemma of how to tackle it, unaware of simple steps that can be taken to ensure data quality.
In a recent survey, over 60% of organisations indicated that too many data sources and inconsistent data was their top data quality worry. So, more needs to be done to ensure that organisations are not overwhelmed with the data they have and, at the same time, are aware of how to handle it.
Despite the sheer amount of data being a top concern, it will be hard for any organisation to reduce the number of data sources it has. If anything, this is only likely to increase over time. But this is not a new problem. It was first tackled when we were still maintaining data in spreadsheets, with data management practitioners coining the term “spreadmart hell” as they tried to maintain data governance over multiple spreadsheets.
Instead of looking at the number of data sources as a problem, we should look at it as a feature and be thankful that technology has progressed to match organisations’ needs. Front-end tools generate metadata and capture provenance and lineage, and data cataloguing software then manages this – so technology has our back. We do, however, have to continue to push a cultural change around data, encouraging people throughout the organisation to ensure data quality, governance and general data literacy.
Some other common data quality issues point to larger, institutional problems. Disorganised data stores and lack of metadata are fundamentally a governance issue and, with only 20% of respondents saying their organisations publish information on data provenance and lineage, very few organisations have adequate governance.
Data governance is not easy to solve and is likely to grow. Poor data-quality controls at data entry are fundamentally where this problem originates. As any good data scientist knows, entry issues are persistent and widespread. Adding to this, practitioners may have little or no control over providers of third-party data, so missing data will always be an issue.
Data governance, like data quality, is fundamentally a socio-technical problem, and as much as machine learning and artificial intelligence (AI) can help, the right people and processes need to be in place to truly make it happen. People and processes are almost always implicated in both the creation and the perpetuation of data-quality issues, so we need to start there.
Organisations must take formal steps to condition and improve their data, such as creating dedicated data-quality teams. This will be an ongoing process, not a one-and-done panacea.
Rachel Roumeliotis is vice-president of data and AI at O’Reilly.
Read more about data governance
- Strong data governance and master data management strategies typically go hand in hand. Read on to see how key factors of data governance can support your master data management.
- Governing by the use of data analytics may be fashionable, but what are its limits as well as its opportunities? Lessons from Estonia, Essex, and healthcare.