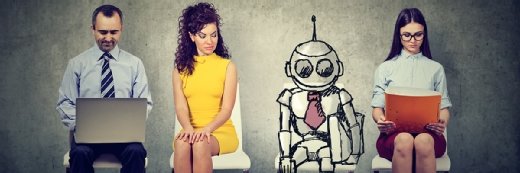
pathdoc - stock.adobe.com
How to avoid AI myopia
Artificial intelligence holds much promise and is generating a lot of excitement, but it can have unintended consequences. Technologists need to avoid repeating the mistakes of the past
The world of artificial intelligence (AI) is full of very smart people. Its pioneers are justly treated like rock stars, as breakthroughs continue to be made in fields as diverse as games and diagnostics.
Presidents, prime ministers and CEOs do all they can to share some of the stardust, and research is pulling in vast sums of money from companies like Google, Facebook and Alibaba, as well as from governments.
It’s likely that AI in its many forms will shape the great majority of machines and processes that surround us over the next few years. But it’s still an open question whether this field will be not just smart, but also wise. Many fields have turned out to be clever but foolish. Hopefully AI won’t be one of them.
Ethics and bias
So far, AI’s leaders have shown a healthy determination to reduce the risks that their technologies do harm. The many moves around ethics and bias signal widespread understanding that powerful technologies bring with them big responsibilities – even if the endless theoretical discussions about trolley problems have often distracted from the more pressing and subtle ethical challenges of AI in the real world.
But the leaders of AI have yet to make a shift in thinking that could be just as vital if their technologies are really going to do good. This is the shift to thinking of intelligence in terms of outcomes rather than inputs.
Technologists inevitably think about how their tool, gadget or algorithm can be used in the world. They start with a solution and then look for problems. This is a necessary part of any innovation process, but in most fields, it’s even more productive to think the other way around – to start with a need or outcome and then look for answers or tools that can help.
There is a long history of digital technologists getting this wrong. From smart cities and smart homes to digital government, too many focused on inputs rather than outcomes, hyping fancy applications or hardware that didn’t really meet any needs that matter. Invariably this led to disappointment, wasted money and backlashes. Too many involved in AI are now repeating exactly the same mistakes.
Smart and stupid
The world badly needs smarter ways of achieving outcomes, whether for running businesses and governments, education and health systems or media. But it’s a paradox, perhaps the paradox, of our times, that proliferating smart technologies have so often coincided with stupider systems.
To understand this, and how it can be avoided, requires better theory as well as better practice. Many involved in data and AI of course work on better outcomes such as better delivery schedules, click-through results or diagnostics. Outcome metrics drive much of the hard work underway in startups and vast multinationals, and hyperparameter optimisation methods formalise this.
But they tend to miss some crucial steps. Engineering theory has always recognised that you can optimise one element of a system in ways that leave the whole system less optimised. This should be obvious around AI – not least because of examples like Facebook that optimised click-throughs in ways that left a neighbouring system, democracy, badly damaged.
Fields like educational technology (edtech) are full of examples of tools that appeared to deliver results, but when looked at in context had little or no effect. As Bill Gates put it last month, at least edtech has probably not done much harm.
Intelligent outcomes
If you really want more intelligent outcomes, and systems that consistently achieve more intelligent outcomes, three conclusions quickly follow – all of which challenge current AI orthodoxy.
First, intelligence in real systems depends, just like human brains, on combining many elements: observation, data, memory, creativity, motor coordination, judgement and wisdom.
AI can contribute a great deal to some of these elements, like predicting where there are large datasets, or the organisation of memory, or the management of warehouses and recommendation engines.
But it offers very little to others, especially those involving nuanced judgements in conditions of uncertainty. So if you want an intelligent education or health system, for example, you have to be interested in hybrids – combinations of machine and human intelligence.
A second conclusion is that combination requires quite complex design, for example including mechanisms to encourage people to share the right ideas and information; shared taxonomies; incentives; culture; and defences against the risks of bias and systematic error.
Read more about AI and ethics
- The winter of AI discontent – thoughts on trends in tech ethics
- IBM pushes boundaries of AI, but insists companies take an ethical approach
- Ethical AI requires collaboration and framework development
We need, and will need even more in the future, both human supervision of machines and machine supervision of humans. Computer and data science offer vital insights into how these processes need to be designed – but without psychology, organisation, economics, decision science and sociology, there are bound to be huge errors. The biggest risk facing the field right now is too much hubris and too little humility.
A third conclusion is that intelligence in the real world involves continuous learning, not just adapting algorithms to new data but also recognising when you need a new category to think about a problem in the right way. AI offers little help in doing this. Yet this is fundamental to how most important systems learn and improve.
These are all aspects of what can be called “intelligence design” – shaping the combination of data, computing and human intelligence to achieve outcomes we care about.
There is a lot to welcome in the big investment in AI around the world. But we need to match this with much more attention to complementary fields like collective intelligence – orchestrating human intelligence at scale. Smart companies realise this – which is why firms as diverse as Lego and Siemens make extensive use of collective intelligence. But it’s rarely mentioned in the hype around AI.
Most of all, we need to focus more on outcomes than inputs. Fixating on the latest algorithm or gadget rather than outcomes risks repeating the mistakes of the past. Addressing this very simple truth now would do a lot to help the AI world avoid an all-too-likely future of disappointment.