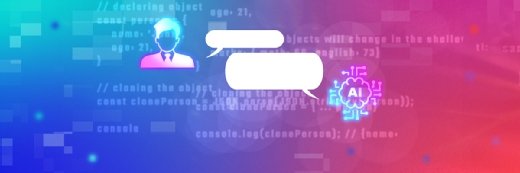
CESM I Studio - stock.adobe.com
Enterprise AI: Best-in-class or best-of-breed?
With generic chatbots underperforming in sectors like travel and telecoms, CIOs must decide if consolidating with one best-in-class provider outweighs the benefits of specialised, best-of-breed AI solutions
Precise personalisation at scale might sound like an oxymoron, but that’s exactly what marketing and consulting giants are investing in for their clients. Imagine an e-commerce brand instantly tailoring recommendations, promotional offers, and content uniquely for millions of customers, or a global social media platform whose artificial intelligence (AI)-powered playlists deliver extraordinarily individualised experiences to hundreds of millions of listeners simultaneously.
So, how do they achieve that kind of precision? Is it through a best-in-class strategy that consolidates AI capabilities under one vendor, or by assembling a ‘best-of-breed’ lineup of specialised tools tailored to each function?
This wave of strategic customisation is why analysts have predicted that the top 10 global advertising agencies will invest $50m to build “brand-specific” AI models, reflecting a shift towards tailored AI adoption. Already, 40% of organisations are developing enterprise-specific large language models (LLMs) and 71% of tech-savvy marketers are using marketing-specific AI tools.
Commercial AI solutions are rapidly becoming more specialised — spanning people management, legal, sales, customer experience (CX), and more. By 2027, more than 50% of the generative AI (GenAI) models that enterprises use will be customised for specific industries or business functions.

We’ve seen firsthand what happens when AI lacks domain specificity. In a recent mystery shopping exercise by our TDCX AI team, we found that in terms of effectiveness, AI chatbots in travel and hospitality, as well as telecommunications, scored below -60 and -70 respectively (see table above). These scores were based on information accessibility, query relevance, task completion, response quality, and issue resolution. These negative scores were largely due to an inability to retain context across interactions, handle nuanced requests, and understand industry-specific queries. Without domain-specific training, the AI system cannot effectively interpret industry terminologies or queries; while multistep resolutions need tech integrations to connect each phase of the customer interaction.
This underperformance raises an important point: A single vendor might cover multiple functions across the enterprise, which can be perceived as operational simplicity. However, they might not always be equipped to meet the specific needs of every touchpoint, whether due to technical limitations or domain expertise.
So, as enterprises deepen their reliance on AI to sharpen their competitive edge, my fellow CIOs face a new kind of strategic crossroads: Should we adopt a best-in-class approach, consolidating our enterprise’s AI capabilities with a single, comprehensive vendor? Or do we embrace a diverse, ‘best-of-breed’ portfolio of specialist vendors tailored to the precise needs of each department?
Historically, enterprise tech has oscillated between these two poles. But with today’s AI tools, the stakes are higher — precision in model performance is no longer just nice to have, but a critical differentiator. I’ve observed that while a single-vendor approach simplifies governance, integration, and procurement, it might underserve teams where specialised intelligence drives significant value, particularly in frontline CX roles, such as operations, where AI helps agents, for example, recommend next actions or detect sentiment based on industry-, market-, or product-specific training. On the other hand, embracing ‘best-of-breed’ AI vendors brings advantages in capability, accuracy, and agility, but it can also introduce complexity in integration, data management, and vendor relationships.
One AI and GenAI vendor to rule them all
The appeal of consolidating around a single vendor is compelling, particularly from an operational perspective. A unified vendor can streamline procurement processes, harmonise integration frameworks, simplify service-level agreements (SLAs), and onboarding. This allows the vendor to function as an integrated strategic partner, often providing a comprehensive suite of tools interconnected seamlessly with major cloud or enterprise productivity platforms.
For CIOs, this consolidated approach brings welcome relief: simplified governance, consistent compliance standards, and uniform system compatibility across the board. Frontline teams benefit from the coherent user experience, while finance departments enjoy the economies of scale — volume discounts and optimised resource allocation that drive down total ownership costs.
However, the best-in-class strategy also comes with limitations. Typically, a single vendor excels at delivering broad functionality, but might lack the deep, specialised expertise necessary for complex and critical business functions. In domains such as customer support analytics or talent acquisition, a generalised solution might fall short in delivering nuance and precision. This convenience-versus-capability dilemma creates a vulnerability where operational simplicity comes at the expense of the specialised excellence that drives competitive advantage.
A fellowship of AI and GenAI providers
In contrast, a ‘best-of-breed’ approach offers enterprises the opportunity to work with multiple vendors, each selected for their specialised expertise and deep industry knowledge. The advantage of this strategy lies in its ability to precisely match departmental needs with finely tuned AI solutions. HR teams can access recruitment analytics built on talent acquisition data, while marketing departments can benefit from GenAI models meticulously calibrated to reflect brand voice. CX teams, too, can deploy predictive tools specifically engineered to decode and enhance human interactions.
This specialisation ensures that each departmental function receives exactly the tools and data insights it requires. However, adopting a ‘best-of-breed’ strategy means navigating increased logistical demands, such as managing numerous vendor relationships, negotiating multiple contracts, and addressing integration challenges that arise from combining diverse technologies. Data governance, interoperability, and consistent user experience across disparate solutions become more challenging to maintain. For IT leaders, the critical task becomes orchestrating these specialised solutions into a cohesive, functional ecosystem without sacrificing agility or increasing operational risk.
The middle path: Orchestrating an AI ecosystem
In practice, enterprise AI strategy rarely falls neatly into an exclusively best-in-class or ‘best-of-breed’ category. The modern CIO is increasingly adopting a hybrid approach, integrating both methodologies into a cohesive, multilayered ecosystem. For instance, 54% of enterprises are already going hybrid, with some resources centralised and others distributed across business functions. This typically involves selecting a partner to handle foundational services and infrastructure, supported by a flexible, specialised outer layer of vendors closely aligned with functional requirements.
This hybrid ecosystem can be visualised less as a traditional vendor “stack” and more as an interconnected “vendor network,” unified by shared data pipelines, consistent governance policies, and standardised integration frameworks. This architecture allows enterprises to maintain operational consistency and strategic control while still providing teams the flexibility and specialised capabilities they need to excel. For CIOs, it represents the technological equivalent of having your cake and eating it too — harnessing both broad platform benefits and the surgical precision of specialist solutions.
Data as single source of truth holds the real power
Regardless of whether enterprises choose a best-in-class, ‘best-of-breed’, or hybrid approach, the foundation of any successful AI deployment lies in data — the single source of truth influencing the accuracy, relevance, and effectiveness of AI outcomes.
Ultimately, AI vendor strategy is becoming a proxy for enterprise agility. Enterprises don’t need to juggle numerous vendors unnecessarily, nor must they put all their trust in a single provider. A smarter strategy is to design the AI ecosystem much like you would a CX strategy: flexible and extensible, human-centred, and performance-focused.
Striking the right balance between vendor simplicity and specialised innovation is key. Data transformation and thoughtful orchestration ensures that the AI ecosystem becomes a strategic enabler rather than an operational burden, driving agility, innovation, and a sustained strategic advantage.
Byron Fernandez is executive vice-president and group CIO of TDCX