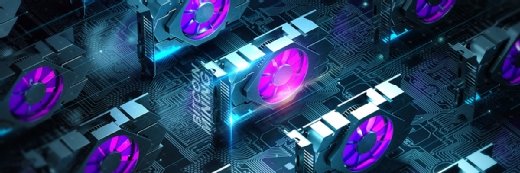
Alexander - stock.adobe.com
Chip sector gears up for AI revolution
While Nvidia has established its AI dominance, Intel has been working on AI chips running on supercomputers
The most recent quarterly earnings from Meta and Google demonstrate the trend among hyperscalers to extend the life of datacentre servers. Google announced it has saved almost $3bn in nine months by extending server life to six years, while Meta’s chief financial officer Susan Li revealed the company could see little performance gain from new server chips. Instead, she said: “We’re still seeing strong performance gains for a new GPU generation, so those will depreciate on a relatively shorter timeframe and we’ll just continue to evaluate how to most efficiently use the CPUs and GPUs across our fleet.”
Recognising the change in buying strategy among the hyperscalers and in anticipation of a shift to artificial intelligence (AI) workload optimisation among enterprise customers, semiconductor manufacturers are beginning to ramp up their AI portfolios.
Nvidia’s share price and strong financial results show how the GPU company has pivoted to becoming the leading supplier of AI-accelerated hardware. Its second quarter earnings saw strong demand for the Nvidia HGX platform based on its Hopper and Ampere GPU architectures, mainly due to the development of large language models and generative AI. This has led to growth of 195% in its datacentre compute business.
The chip company is working with HPE’s Cray supercomputer division on a family of AI-optimised supercomputers based on its Grace Hopper GH200 Superchips. HPE said the combination of AI software and hardware offers organisations the scale and performance required for big AI workloads, such as training large language models (LLMs) and deep learning recommendation models (DLRMs). According to HPE, using its Machine Learning Development Environment on this system, the open source 70 billion-parameter Llama 2 model was fine-tuned in less than three minutes. Overall system performance improved by 200% to 300%.
Rival chipmaker Intel reported a 10% decline in its datacentre and AI business in the third quarter of 2023. Keen to plug this gap, Intel has begun ramping up its AI efforts. At SC23, the international conference for high-performance computing (HPC), networking, storage and analysis, it unveiled work on a new supercomputer, Aurora, with Argonne National Laboratory and industry partners to create what it describes as “state-of-the-art foundational AI models for science”. Intel said the models will be trained on scientific texts, code and science datasets at scales of more than one trillion parameters from diverse scientific domains to support multiple scientific disciplines, including biology, cancer research, climate science, cosmology and materials science.
Using the Intel Max Series GPU architecture and the Aurora supercomputer system, Intel said the hardware is capable of handling one trillion-parameter models with just 64 nodes, which it claimed is far fewer than would be typically required.
According to Intel, the GPU Max Series 1550 outperforms Nvidia H100 PCIe card by an average of 36% (1.36x) on diverse HPC workloads.
Intel also showed benchmark tests conducted with Dell using the STAC-A2 independent benchmark suite for real-world market risk analysis workloads. Compared to eight Nvidia H100 PCIe GPUs, Intel said that four Intel Data Center GPU Max 1550s had 26% higher warm Greeks 10-100k-1260 performance and 4.3 times higher space efficiency.
Arm recently announced it was working with companies including AMD, Intel, Microsoft, Nvidia, and Qualcomm Technologies, on a range of initiatives focused on enabling advanced AI capabilities for more responsive and more secure user experiences. Arm said these partnerships would create the foundational frameworks, technologies, and specifications required for more than 15 million Arm developers to deliver AI experiences.
Beyond datacentre computing, Arm said it is collaborating with Meta on the deployment of neural networks at the edge. Arm and Meta are working on ExecuTorch, which brings the open source PyTorch deep learning framework to Arm-based mobile and embedded platforms at the edge. According to Arm, ExecuTorch simplifies the deployment of neural networks that are needed for advanced AI and ML workloads across mobile and edge devices. Arm’s long-term goal is to ensure AI and ML models can be easily developed and deployed with PyTorch and ExecuTorch.
In December, AMD is also set to unveil the work it is doing on AI.
Given the investments in AI hardware being made by the hyperscalers, the public cloud is gearing up to become the preferred deployment choice for machine learning and AI inference workloads. So long as IT governance policies are followed, corporate data can be deployed on AI hardware infrastructure in the public cloud safely, to enable companies to run machine learning and build data models for their AI-based applications.
LLMs generally rely on vast swathes of public data combined with domain-specific data, which is largely proprietary. This means that internal data cannot easily be integrated with public data to improve accuracy based on company-specific information. Used intenally, an HPC environment effectively offers enterprise users access to AI-acceleration hardware inside the corporate network. The fact that HPE and Intel are focusing on HPC for AI may pave the way to widening the application areas for supercomputers beyond research and development and scientific computing.
Read more about AI supercomputers
- HPE unveils cloud AI services powered by its Cray supercomputers working in tandem with a version of its GreenLake for Large Language Models.
- Nvidia GPUDirect Storage's driver hit 1.0 status to enable direct memory access between GPU and storage and boost the performance of data-intensive AI, HPC and analytics workload.
- Bristol and Cambridge to host 2024 AI supercomputers - Dell and HPE are working on supercomputers with the two universities as part of the UK government’s artificial intelligence funding drive.