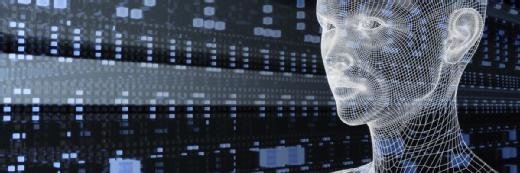
AI accountability held back by ‘audit-washing’ practices
Algorithmic auditing will be useless in holding artificial intelligence accountable until there are common standards, approaches and goals that scrutinise systems at each stage of development and deployment, says think-tank
Inadequate and ill-defined algorithmic auditing processes are being used to mask problematic or illegal practices with artificial intelligence (AI), according to a German Marshall Fund (GMF) report.
Published under the GMF think-tank’s Digital Innovation and Democracy Initiative, the report said that while algorithmic audits can help correct for the opacity of AI systems, poorly designed or executed audits are at best meaningless, and at worst can deflect attention from, or even excuse, the harms they are supposed to mitigate.
This is otherwise known as “audit-washing”, and the report said many of the tech industry’s current auditing practices provide false assurance because companies are either conducting their own self-assessments or, when there are outside checks, are still assessed according to their own goals rather than conformity to third-party standards.
“If well-designed and implemented, audits can abet transparency and explainability,” said the report. “They can make visible aspects of system construction and operation that would otherwise be hidden. Audits can also substitute for transparency and explainability. Instead of relying on those who develop and deploy algorithmic systems to explain or disclose, auditors investigate the systems themselves.
“Audits will not advance trustworthy AI or platform accountability unless they are trustworthy themselves.”
To ensure that algorithmic audits become a trustworthy and meaningful part of AI governance, GMF said a number of key questions should be considered.
These include who is doing the auditing. For example, while internal audits may be useful to catch problems before they affect people, GMF said this process is inherently untrustworthy because it could be used to provide unverifiable assertions that the AI has passed legal or ethical standards.
External audits, on the other hand, while unable to catch problems early due to necessarily looking backwards through the AI development process, will typically exhibit independence from the deploying organisation, signalling trustworthiness and compliance.
In July 2022, for example, Meta published its first-ever human rights report, which was conducted by the company itself. While it detailed the corporation’s most “salient risks” and how it creates “rights-respecting practices, decisions, approaches and products,” campaigners said at the time that Meta had neglected to properly examine the negative rights impacts of its own surveillance-based business model.
Other questions that organisations should consider when auditing include defining what exactly is being audited, and when. The GMF report said: “An algorithmic process runs from specification of the problem through data collection, modelling and validation to deployment and even post-deployment adjustments. For dynamic processes, like social media algorithms, this process is iterative and constantly renewing.
“Algorithmic auditing provisions using terms like ‘risk assessment’ or ‘audit’ are often vague about the object and timing of the inquiry, and whether they intend to look at the full lifecycle of an AI system or only parts of it.”
It added: “Another common pitfall in the technology space is for a firm to profess adherence to human rights standards without actually designing its systems to deliver on them.”
Read more about AI and algorithms
- The House of Commons Science and Technology Committee has launched an inquiry into the UK’s governance of artificial intelligence, which will examine how to ensure the technology is used in an ethical and responsible way.
- A coalition of UK digital regulators is calling for views on algorithmic processing and auditing to help streamline and shape the regulators’ collective work going forward, as well as identify further areas of common interest between the organisations.
- The massive proliferation of ethical frameworks for artificial intelligence has done little to change how the technology is developed and deployed, with experts questioning the tech industry’s commitment to making it a positive social force.
In the first-ever International Algorithmic Auditing Conference – a forum set up for auditing experts and practitioners to share knowledge and best practice for how organisations can manage their AI systems responsibly – the consensus was that organisations must conduct end-to-end audits that consider both the social and technical aspects of AI to fully understand the impacts of any given system.
Drawing similar conclusions to GMF, the auditing experts said a major part of the problem is that AI systems are often developed in a haphazard way, with firms not properly documenting the development process, making it much harder to conduct these “socio-technical” audits later on.
To be meaningful, GMF said there needs to be a set of common standards, which remain “contested and elusive” because of a lack of agreement about goals and basic definitions between auditors and the audited.
It said auditing provisions should also be clear about the issue being addressed by the audit. For example, whether they are conducted to fulfil a legal requirement or as an ethical goal, most audits will look to address issues around fairness, explainability, redress, privacy, or robustness and security.
“The standards the audit uses to assess norms like fairness, privacy and accuracy should be as consensus-driven as possible,” it said. “In the absence of consensus, which will be frequent, the standards being applied should be at minimum well-articulated. A situation in which auditors propose their own standards is not ideal.
“Common, or at least evident, standards will foster civil society’s development of certifications and seals for algorithmic systems, while nebulous and conflicting standards will make it easier to ‘audit-wash’ systems, giving the false impression of rigorous vetting.”
Giving evidence to Parliament’s Business, Energy and Industrial Strategy (BEIS) Committee on how AI affects the workplace, Anna Thomas, director of the Institute for the Future of Work, said: “Auditing tools themselves will rarely be explicit about the purpose of the audit, or key definitions including equality and fairness.” She added that many cultural assumptions around these concepts are imported from the US.
In a report published on 17 November 2022, entitled Rethinking data and rebalancing digital power, the Ada Lovelace Institute argued for greater public participation in the scrutiny of data and algorithms, which could help overcome some of these issues.
“Panels or juries of citizens could be coordinated by specialised civil society organisations to provide input on the audit and assessment of datasets and algorithms that have significant societal impacts and effects,” it said, adding that “participatory co-design or deliberative assemblies” could also be used to help bake public interest considerations into the design process.
The GMF report was authored by Ellen P Goodman, a former GMF senior fellow and current senior adviser for algorithmic justice at the National Telecommunications and Information Administration, and GMF programme manager and fellow Julia Tréhu.