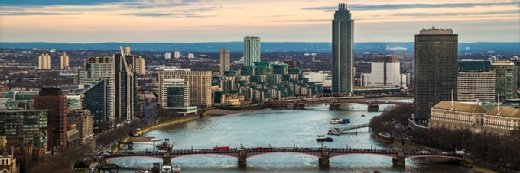
zgphotography - stock.adobe.com
Big Data London: Mitigate AI bias rather than try to remove it, say experts
Bias cannot be stripped from artificial intelligence systems, but organisations can work to mitigate it, Big Data London 2021 attendees heard this week
Bias cannot be removed from artificial intelligence (AI) systems but organisations can work to mitigate it, according to speakers discussing the ethics of AI at Big Data LDN this week.
“You’ll never solve bias,” said Simon Asplen-Taylor, a consultant who has worked on data for major organisations over three decades. “Unless you understand every kind of bias, you’ll never be able to fix it.”
He suggested organisations should instead seek to understand how data is gathered and consider context when looking at the results of an AI model.
Charlie Beveridge, who advises startups on using AI, said bias may be inescapable in existing data but it could be reduced in future by gathering more contextual information. However, she said tools that aim to do this usually focus on legal protected characteristics such as ethnicity, sexuality or gender, rather than a broader consideration of an individual’s particular circumstances.
“How could we build something that mitigates the disadvantages and advantages that people are experiencing, as opposed to arbitrarily assuming that everyone in the same group has exactly the same experience?” she asked.
Chris Fregly, principal engineer on AI and machine learning for Amazon Web Services, added that even if organisations believe their own data is free of bias, they are likely to introduce it if they use pre-trained AI models. “The best we can do is at least detect it and try to work around it,” he said.
Panellists were more optimistic about lessening the environmental impact of artificial intelligence work. Sophia Goldberg, senior data scientist at broadcaster Sky – which plans to reach net-zero carbon by 2030 – said improving AI’s efficiency so that it can generate similar performance from much less computation is a growing area of interest for researchers.
“I’m hopeful that will continue as a trend and as an active area of research,” she told the event. “If that continues, we’ll be in a good place.”
AWS’s Fregly said the statistical method of distillation could be 97% as accurate as standard AI models while using millions rather than billions of parameters. Beveridge added more broadly that organisations would need to consider efficiency in future. “We have to change our mindset with the way we do AI,” she said.
Asplen-Taylor said organisations should approach AI differently depending on their size. He has worked with insurance startups that design their businesses around how they use AI, while mid-sized organisations that do not have the capacity to set up a dedicated team should consider partnering. Large organisations should consider using AI initially in a lower-risk area that provides benefits if it works rather than causing damage if it doesn’t, such as spotting faults.
Goldberg said it could be difficult to innovate in large companies, although Sky tackles this by focusing her department specifically on innovation. She added that it was important to consider the problem that needs to be solved rather than particular technologies.
“When you’ve got an ML [machine learning] problem, the first question to ask is [whether] you need to use ML,” she said. “Machine learning is just a tool and there are lots of other really cool tools out there that can help solve business problems.”
Read more about AI and bias
- What is machine learning bias (AI bias)?
- Why algorithmic auditing can't fully cope with AI bias.
- Combating racial bias in AI in hiring.