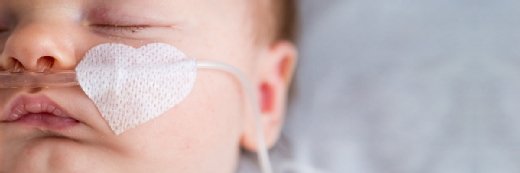
lavizzara - stock.adobe.com
How big data is solving future health challenges
An Australian paediatric research institute has started a big data initiative to facilitate early intervention in childhood conditions that may pose problems to people later in life
A big data project spearheaded by the Murdoch Children’s Research Institute (MCRI) in Melbourne to bring together multiple data sources could enable doctors to intervene earlier in childhood conditions.
Called Generation Victoria (GenV), the project is looking into several conditions, such as asthma, autism, allergies and obesity, to understand how those affected people as they became older.
But part of the challenge in projects such as GenV is the way research is typically done. According to Melissa Wake, GenV’s scientific director, researchers usually conduct their own research and collect their own data, which slows down the research process.
She likened it to taking a long train journey but having to build a new station and trains for each trip rather than leveraging an existing network. “We know that healthy children create healthy adults,” she said. “By 2035, we’re aiming to solve complex problems facing children and the adults they will become.”
GenV securely links data from a variety of national and Victorian data sources and will, with consent, use data from about 160,000 newborn children. This includes clinical information, data from wearables, and other sources from before birth through to old age. This data was never designed to be used together.
A jigsaw of unmatched pieces
Michael Stringer, GenV’s big data project manager at MCRI, said acquiring research data is the hard part.
“This is where a lot of effort goes. You can get data from participants through questionnaires and assessments. But with GenV we’re trying to get the data from all the ways they interact with existing services,” he said.
Data can come from hospital visits, immunisation records and records from local doctors, captured in a variety of formats including databases and images. Adding to the challenge is that there is no off-the-shelf data management tool for researchers.
“There’s no SAP for research processes,” Stringer said. “The best you can do is buy a collection of packages that do different parts of it and integrate it together as a coherent whole.”
The framework of GenV is designed so researchers can get a one-stop shop for research where they can leverage existing data securely.
A data model is vital
At the centre of GenV is the LifeCourse data repository, where data from a variety of sources can be accessed by researchers and other users. One of the keys here is having an effective data model.
Stringer said: “A well-curated data model is essential to having that database maintaining its value. It’s an effective way of transferring knowledge over the life of the database. Without it, the data is fragmented, and you end up solving the same problems multiple times”.
The model also ensures that when new data sources are used in future, they can be properly integrated. There is also a significant focus on metadata, which makes up about half of the data in the GenV system.
“Without that metadata – how it’s classified, what each particular variable means, what its quality level is – no one can actually use the information,” Stringer said.
Balancing quality and quantity
Unlike many other data warehousing projects, Stringer said the focus is not just on collecting and using data if it has a specific quality level. Instead, when data is added to LifeCourse, its quality level is noted so researchers can decide for themselves if the data should or should not be used in their research.
The GenV initiative relies on different technologies, but the two core pieces are the Informatica big data management platform and Zetaris.
Informatica is used where traditional extract, transform and load (ETL) processes are needed because of its strong focus on usability. Stringer said this criterion was heavily weighted in the product selection process. Usability, he said, is a strong analogue for productivity.
But with a dependence on external data sources and a need to integrate more data sources over the coming decades, Stringer said there needed to be a way to use new datasets wherever they resided.
That was why Zetaris was chosen. Rather than rely on ETL processes, Stringer said the Zetaris platform lets GenV integrate data from sources where ETL is not viable.
For example, many government data sources cannot be copied, but Zetaris allows the data to be integrated – through a data fabric – into queries run by researchers without extracting the data into a data warehouse.
Although the problems being solved through GenV by the MCRI are significant, the underlying challenges faced are the same as those of many organisations. Businesses today are dealing with large volumes of data from multiple sources, all structured in different ways.
Be it customer surveys, social media comments, website traffic or information from point-of-sale or finance systems, businesses need to be able to quickly and easily bring together different sorts of data in order to make good decisions.
The lessons from the GenV project are clear. Businesses must understand the problems they are trying to solve, invest time to create a strong data model, understand the sources and quality of data, and avoid creating a system that is limited to what they know today.
Read more about big data analytics in Australia
- Australian genome researchers have developed solutions to tackle big data challenges orders of magnitude more complex than anything previously possible.
- Analytics startup Kinetica is bullish about the Australian market as more organisations leverage GPU-based platforms to support real-time analytics.
- Organisations in Australia are among the most advanced in the Asia-Pacific region in harnessing data to achieve key business outcomes.
- Australian firms appear to have made the most progress in reducing the volume of dark data over the past year, study finds.