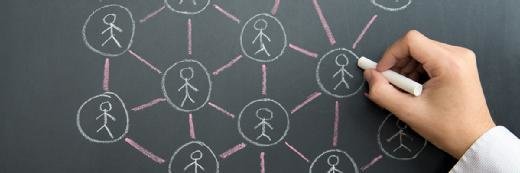
Kenishirotie - Fotolia
F-Secure’s AI reads mean tweets to fight abuse and trolls
Researchers working on F-Secure’s Project Blackfin have developed a model for clustering tweets to help pinpoint abuse and harassment
Researchers at F-Secure’s Artificial Intelligence Centre of Excellence and the University of Crete’s Forth-IS Institute have developed a novel method for categorising tweets that they hope will, in future, help platforms such as Twitter clamp down on bad behaviour and deal more effectively with abuse, harassment and other forms of malicious activity.
Working under the auspices of F-Secure’s Project Blackfin, researcher Andy Patel and PhD student Alexandros Kornilakis conducted experiments on replies to US president Donald Trump and other US politicians, including the field of Democratic candidates vying to take Trump on in the November 2020 election.
Patel said that the “torrential downpour” of content on social media gave bad actors cover to spread misinformation, hoaxes, lies, scams and fake news, and the inability of sites to stop this kind of behaviour was creating a marketplace for likes, views, subscribes, reviews, and fake accounts.
In their paper, A New, novel method for clustering tweets, Patel and Kornilakis set out a new method of clustering – the process of using machine learning to group phrases or passages into buckets based on their topic.
They developed a methodology that involved processing captured data, converting tweets into sentence vectors, combining said vectors into meta-embeddings, and then creating node-edge graphs using similarities between calculated meta-embeddings, from which the clusters were then derived.
Patel and Kornilakis’ tested their methodology around multiple events, including the 2019 UK General Election which set a new high-water mark for abusive behaviour online. The bulk of their research, however, centred on over a million replies to tweets sent by Trump, the Democratic Party candidates, and congresswoman Alexandra Ocasio-Cortez.
According to Patel, the online mentions of such politicians present an extreme version of what the average Twitter user may have to deal with. Inevitably, they receive lots of engagement, and it usually skews extremely positive or negative.
Patel and Kornilakis classified the mentions by identifiers such as subject-verb-object and overall sentiment and then used their methodology to build an average sentiment score. Based on these identifiers, posts were classified as positive, negative or toxic.
In the case of the Democrats, the most common negative tweets included terms such as “you are an idiot/moron/liar/traitor”, “you will never be president” and “Trump will win”. More positive themes included “we love you”, “you got this” and “you have my vote”.
The little-fancied Andy Yang received by far the most positive replies, followed by Bernie Sanders and Amy Klobuchar, while Alexandra Ocasio-Cortez and Elizabeth Warren received the most toxic posts.
However, when compared to Trump, none of the Democrats attracted as much toxicity, with the most common themes including “you are an idiot/liar/disgrace/criminal”, “you are not our president”, “you have no idea/you know nothing”, “you should shut up” and “you can’t stop lying”. Many also included references to Vladimir Putin. Trump’s positive mentions tended to include themes such as “God bless you” and “we love you”.
Patel hopes the methodology can be used to help reduce the misuse of Twitter by drawing attention to problematic content before it generates traction. This could prove particularly useful in countering life-threatening misinformation, he said.
“For instance, our methodology automatically identified and grouped tweets pushing a hoax that the Australian bush fires had been caused by arsonists,” said Patel.
“Additional research is necessary, but with some more development there could be a range of potential applications. This methodology could be used for automated filtering or removal of spam, disinformation and other toxic content. This could be done by assigning quality scores to accounts based on how often they post toxic content or harass users.”
The researchers have set up a website for interested individuals to explore the data, and the project’s code can be found on Github.
Project Blackfin was set up in 2019 at F-Secure’s Helsinki headquarters, and is described as a research programme dedicated to the development of decentralised AI for cyber security. The project researchers also hope to take artificial intelligence (AI) to the next level by challenging the common misconception that AIs should mimic human intelligence.
Read more about social media
- The Centre for Data Ethics and Innovation is recommending regulation of social media algorithms as part of a drive to make user targeting safe and ethical.
- Learn how social networking sites compound the insider threat risk, and explore how to mitigate the threat with policy, training and technology.
- Falcon.io, a social media marketing vendor, turned to AI vendor Lexalytics to add automatic social media sentiment analysis capabilities to its platform.