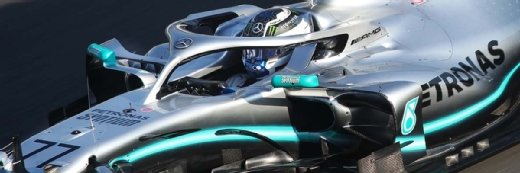
Daimler AG
How data drives wins at Mercedes-AMG Petronas Motorsport
We find out how Mercedes-AMG Petronas Motorsport uses data to drive its business and Formula One race strategy
The recent Russian Formula One Grand Prix demonstrated a real-time, reactive strategy that enabled Mercedes-AMG Petronas Motorsport to overtake a possible Ferrari one-two win.
Data is critical to every aspect of how Mercedes-AMG Petronas Motorsport runs, designs, builds and tests its race car and its race strategy.
Last year, the team celebrated its fifth consecutive double title after securing the FIA Formula One Constructors and the UK’s Lewis Hamilton took the Driver’s world championship.
As the 2019 Formula One season enters its final stages, there is pressure on all aspects of the business. Data is at the heart of the Mercedes-AMG Petronas Motorsport team’s decision-making, both from a race strategy perspective and in its wide business operations.
Matt Harris, CTO of Mercedes-AMG Petronas Motorsport, says: “Over the last five years, the importance of data coming from the car hasn’t got any different, but the time to make decisions has got much tighter.”
Harris says there is now a criticality in being able to visualise and turn data into insights and decisions that change the car’s configurations and what the race team needs to do during practice, qualifying and on race day. There is so much data – the telemetry data from the sensors on the car and the data from the race team, pre- and post-race simulations, dyno engine tests, complex fluid dynamics (CFD) and wind tunnel aerodynamics data.
“The holy grail would be to do a test in dyno, relate that to the simulator, correlate with wind tunnel and CFD data, run the setup on track then change one number and all the data points agree,” he says.
mug.jpg)
“We are forever chasing an optimal model of the physical world”
Matt Harris, Mercedes-AMG Petronas Motorsport
This is the concept of a Digital twin, a term used in heavy industry to describe a digital representation of a physical machine. But a true digital representation of a Formula One car is almost impossible, says Harris.
“It is almost impossible for us to get to, but what we want to get is to do P1 [the first practice session during a race weekend], learn from the conditions, understand where the software simulation was incorrect, and redo the simulation. We are forever chasing an optimal model of the physical world, minute to minute, hour to hour, day to day, from qualifying to racing.”
This iterative process continues after the race. It is updated post-race, and as and when improvements are made to the race car.
Read more about data-driven business
- Data is the new oil – but without tight control, how can business determine that the data is valid? Data catalogues are back in vogue.
- Crafting a data strategy is hard. Use cases help. At the MIT CIO Symposium, four IT leaders share how they are forging data-driven strategies that will pay off in the algorithmic economy.
Harris says Mercedes-AMG Petronas Motorsport is working with Tibco on a project to look at how to feed the performance data from the car’s telemetry systems into time series databases, which can be used to visualise different datasets to identify anomalies and correlations.
The project represents a big step forward for the team in the ability to analyse data, he says. “This will be a massive step-change for next year. It’s hard to look at car [telemetry] data. There are thousands of channels of data.”
Harris says there is so much data that engineers need to know which dataset they should be looking at and what they are looking for in that dataset. “You only know what to look for if you know it’s an important piece of information,” he says. “With Tibco Spotfire, we will get trends on channels. A quick win will be the ability to look for anomalous activities.”
Data is essential on and off the track. Given the cost caps in Formula One, the team needs to know it is spending money in the right areas. Harris says: “We have different ways to do scheduling, production orders and programmes to manufacture the car.”
Make the right decisions
Some of the data is in the company’s SAP enterprise resource planning (ERP) system, but not all data is in the ERP. Harris says the status in these different systems needs to be visualised in a standardised way. “Understanding business processes and business data allows us to do ‘what if’ scenarios, so we can make the right decisions up front,” he says.
Although there is a vast amount of valuable data generated by the car, Harris says: “We don’t have a rich description of the car’s data.”
Along with the car’s data, the team can correlate GPS data, timing data and weather data. But as for historical analysis, Harris says: “If I looked at data from a year ago with a car from the previous year, the weather would have been different and there may be different sensors compared to the current car.”
The compound used in the tyres will also have changed and the track may have been resurfaced, he adds. All of these differences make it far harder to correlate historical data with data from the current car.
With Hamilton and Valterri Bottas, the Mercedes-AMG Petronas Motorsport team has the best drivers in the world and their input becomes a fundamental part of the data pipeline. What the drivers say over the team radio and during the race weekend is correlated with the machine-generated data from numerous sources, including the car’s telemetry, the simulation data, CFD data, dyno data and wind tunnel data.
Harris says: “We have to take in what the driver has told us, find a correlation within the data and understand what has happened. Sometimes it may be a feeling. The drivers are more accurate than the sensors on the cars. They can give us more feedback than the data and so we need to turn round and trust the driver.”