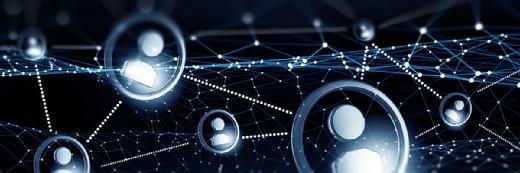
Sergey Nivens - Fotolia
With auditability, deep learning could revolutionise insurance industry
The likes of Google may be pushing deep learning in artificial intelligence, but it may not be the best approach for regulated industries
Deep learning has the potential to revolutionise the insurance sector – but the challenge is how to make the artificial intelligence (AI) models auditable.
Speaking at the Deep Learning in Finance summit, Michael Natusch, group head of AI at Prudential, discussed how the insurer was starting to use deep learning.
“At a high level, you need data and some kind of model to make sense of what is in that data,” he said. “You also need a user interface so that experts are not needed whenever the business wants to ask a new question.”
Natusch said the user interface needs to triggers some kind of action, so that there is a feedback loop to refine the algorithm. “The key is to learn from the actions we want to drive,” he said.
According to Natusch, the choice of algorithm boils down to the value or risk of making a wrong decision based on the AI. “There is a volume element and a cost of getting the wrong decision,” he said.
As an example, he pointed to Google, which is using deep learning to amass understanding, such as identifying an image, based on collecting meta data from millions of people.
Given the low level of risk involved, said Natusch, “correlation is sufficient to drive action”. For applications such as Google’s photo identification, neural network-based algorithms are sufficient, he said.
But in a risk-averse use case, such as decision-making in healthcare, people need to understand why a given decision was made, he said. This requires a causation-based approach, more suited to probabilistic graphical models, he added.
Speaking of his experiences at Prudential, Natusch said: “We need two models – one to understand historical data, and something for handwriting recognition.”
Discussing how handwriting recognition could streamline claims processing at the insurer, Natusch said that once a paper claims form is scanned, it ends up as a grayscale image. This is effectively a set of numbers that can be analysed using a neural network.
Read more about deep learning
- Deep learning can help customer insight teams advance rapidly in their mission to understand their customers.
- Deep learning professionals require a specific set of skills and tools. Follow this simple example of a data science project to learn more about the technology and related careers.
Once the handwriting recognition process is run, said Natusch, “you then need to use machine learning to understand what the form actually means”.
Deep learning gives the insurer the opportunity to enable a fully automated claims process and get additional customer insight from the claims forms. “This is what we are heading,” he said.
For Natusch, deep learning should not be treated as a big IT programme. “If you treat it this way, it won’t go anywhere,” he said.
Instead, Natusch argued that it is better to develop alpha prototypes in small teams, which can then be tested with colleagues and put into wider beta programmes.
“You need to prove this works by building small prototypes and then ramp up the maturity model,” he said. “Experimentation is cheap.”
Specifically, Natusch recommended starting out by processing historical data, then evolving to standalone machine learning. The next stage of maturity would involve developing predictive models with APIs, which leads to greater automated decision-making, he said, and the pinnacle of deep learning is contiguous learning loops for fully augmented decision-making. “We see a step change in the way we do business,” he said.
Auditing recorded customer telephone calls is another big opportunity for machine learning, said Natusch. “We have to listen to half a million hours of phone calls. Those phone calls range from amusing to absolutely bewildering. There is an opportunity to listen to those calls automatically.”