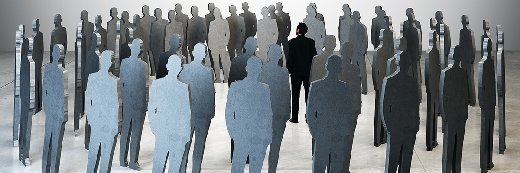
kantver - Fotolia
Machines delve deep into customer minds
Deep learning can help customer insight teams advance rapidly in their mission to understand their customers
Customer insight professionals charged with gaining a wider understanding of customers from the growing volumes of structured and unstructured data accruing in real time can benefit greatly from deep learning.
Many organisations are sitting on a wealth of untapped data in the form of call centre recordings and customer emails that contain valuable insights into the customer experience, while e-commerce sites have a lot of product image data that is ripe for classification and analysis.
These are examples of unstructured data sources that can be fed into deep learning algorithms to improve customer insights.
In most cases, there is no need to start from scratch – deep learning comes embedded in the voice, image and natural language processing (NLP) cloud services offered by suppliers.
There are many use cases to start with, and you don’t have to be an expert to leverage many of these capabilities.
Whether you are in retail, manufacturing, finance, healthcare, government, or any other vertical market, Forrester has identified three proven use cases and some options for implementing them based on deep learning.
Untapped data
The first is speech analytics. Speech analytics tools use deep learning to understand and interpret the spoken word. Typically, these technologies consist of three pieces: acoustic speech recognition, which “hears” speech; speech-to-text transcription; and text analytics, which understands the text.
Customer insight professionals who support call centre insights can employ speech analytics tools to identify customer pain points and areas of risk lurking in call centre recordings. Products such as Nice and CallMiner Eureka use Nuance Communication’s deep learning model for speech recognition to analyse and categorise speech data based on a variety of topics.
Read more about deep learning
AI can help companies shrink the gap between customer data and actionable insight by feeding intelligence into CRM, marketing automation and other operational tools.
When deploying deep learning models into production, experts say it's important to take care of the basics, like model design and testing, to ensure optimal business impact.
But the corpus of publicly available speech data available to train these solutions is not nearly as diverse or robust as that which text and image analytics tools are trained on. Expect to partner with your speech analytics supplier to retrain the solution with your own data so it learns to pick up the most important elements in your customer conversations.
The second application where deep learning can offer a real improvement is in traditional text analytics, which relies heavily on keyword analysis. In 2016, only 27% of customer insight professionals reported analysing their unstructured customer feedback data for insights. This is unfortunate, because this rich data source offers a view into customers’ perceptions, intents and feelings.
Additionally, customer insight teams may use the structured output of text analytics as input into another model, to more accurately predict churn, for example. Some customer insight staff are even using text analytics to help develop chatbots for customer service and conversational commerce.
Text analytics as a category is not new, but only a few suppliers are building in deep learning capabilities. Cloud services from Amazon, Baidu, Google, IBM and Microsoft provide deep learning application programming interfaces (APIs) for NLP and text understanding. A few others have developed deep learning alternatives for text analytics as well. Cortical.io leverages Numenta’s hierarchical temporal memory model for unsupervised text analysis, and Gamalon has developed an algorithm called Bayesian Program Synthesis (BPS) to power its natural language processing engine.
Deep learning also offers enhanced image recognition with very high precision, in many cases better than what humans can do. It is possible to use deep learning algorithms to classify and interpret new images, different from ones used during model training, and identify objects within the images, understand the image itself, write captions about the image, and match with what it knows about images and figure out similarities in real time.
For example, customer insight teams could analyse image data from social media to understand a customer’s lifestyle and enrich their segmentation models.
Emotion recognition, a sub-category of image analysis, may eventually allow customer insight professionals to detect and alleviate customer frustration in stores. Many social media monitoring companies, such as Crimson Hexagon and Talkwalker, have begun offering image analysis as part of their solutions. And Affectiva is the current leader in emotion recognition. Tech-savvy customer insight professionals who are sitting on a corpus of their own image data can use pretrained models exposed as APIs from Amazon Web Services (AWS), Google, IBM, Microsoft and Salesforce.
For more domain-specific applications, customer insight professionals will have to train their own models. Some deep learning frameworks, such as Caffe, are specifically designed for image analysis, but most are more general, such as TensorFlow, and can be trained and used for horizontal domains other than image and video recognition.
Easy, intermediate, expert: Three ways to get started
Enterprises don’t need to research or develop their own deep learning algorithms because these are available either for free in open source solutions or increasingly within commercial machine learning. Instead, the opportunity lies in identifying business problems that map well to deep learning use cases, having good data – and a lot of it – and applying the right strategy to build the model, train it, and test it.
The easiest way to get started in deep learning is to use models that have already been trained. You can either rely fully on pretrained models or improve them with your own data through a process called transfer learning. Transfer learning is one of the key benefits of deep learning because it enables developers to build on existing models instead of starting from scratch. This obviates the need for large amounts of training data.
Pretrained models are available for image, speech and text analytics. For example, customer insight professionals interested in image recognition can leverage APIs from Caffe, Clarifai, Google, IBM and Salesforce, among others, that have already trained their models on huge corpuses of image data.
The intermediate stage is about adopting a low-code approach. To democratise the use of deep learning, some suppliers have developed low-code platforms.
Bonsai, whose tagline is “AI for everyone”, offers a simplified platform to make deep learning and other machine learning algorithms accessible to data scientists with basic coding skills. Other companies have embedded deep learning in their more traditional machine learning offerings. For example, H2O.ai embeds Google’s open source TensorFlow in its product, and SAS offers its own deep learning algorithms in its platform.
The most involved approach is for companies to build their own model. Those with very specific data and use cases may prefer to train a deep neural network from scratch. In this case, companies will use their data scientists to “bring their own data” to a supplier’s deep learning platform, which is essentially a library of neural networks.
Unlike pretrained models, these algorithms have not seen any data, and they are therefore a tabula rasa with no preconceived notions of the world. Developers can download open source libraries, such as Caffe, MXNet and TensorFlow, or use libraries that have already been integrated into suppliers’ machine learning products.
Human-like intelligence
The end result of the deep learning revolution will be apps with a humanlike ability to sense the world around them. Whether you are trying to assess a customer’s emotional state, gauge intent during a phone conversation, or read through thousands of pages of tax code, deep learning will be the central nervous system.
This article is based on an extract from a Forrester report entitled Deep learning: The start of an AI revolution for customer insights professionals by Brandon Purcell, a senior analyst at Forrester.