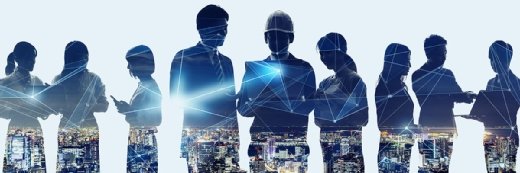
metamorworks - stock.adobe.com
Fuzzy logic: The challenge of building data science teams
In the face of a dearth of data science talent, and a crippling lack of diversity in the pool as it is, what are companies doing to build, manage and keep data science teams together? We find out
“There is no doubt that data science is one of the most challenging areas to recruit and retain teams,” says Richard James, senior data recruiter at tech recruitment and outsourcing consultancy Harvey Nash. It’s the consequence of an almost perfect storm of problems impacting recruiters, where demand is outstripping supply and the supply line is littered with inconsistencies and inadequacies in fundamental skills development.
This is having a significant impact on business. According to James, Harvey Nash’s recent Digital leadership report found that two-thirds of digital leaders in the UK are unable to keep pace with change because of a dearth of the talent they need. While cyber security tops the charts for the most in-demand skills, big data and analytics come in a close second.
With data increasingly touching every aspect of business, this is understandably a worry. If data is supposed to offer competitive advantage, how can UK businesses compete, especially in a recruitment market that has been hit by the double whammy of Covid-19 and Brexit?
The UK government published a policy paper in May 2021, entitled Quantifying the UK data skills gap, to try to assess the scale of the problem and the main causes. It found that nearly half of businesses interviewed were currently recruiting for data roles, but a similar number – just under 46% – had struggled to fill data roles over the past two years.
It’s a problem that is not really being addressed and while government interest is welcome, you feel the horse bolted quite a while ago on this one.
“There is often a disparity between graduate experience and skills and the real-life business understanding that is needed to be effective in the role,” adds James. “With a shortage of skills in this area, competition for candidates is huge.”
Of course, it is natural to think that a steady stream of graduates attracted by good salaries may come to the rescue, but for Ved Sen, business innovation leader at Tata Consultancy Services (TCS), the problem runs even deeper.
“There is a shortage of STEM [science, technology, engineering and maths] skills and individuals with high-level maths and science qualifications, and also a lack of knowledge of programming languages like Python,” he says. “The statistics and probability element of maths also need to be emphasised more, as there is so much data being collected today, but we need to help students understand how it can be applied in a real-world business context.”
Sen also highlights a lack of diversity in data science and a need to “make data cool” and “find ways of encouraging girls into taking STEM subjects at 14, 16 and 18 years of age”. It’s a big challenge. According to ONS data, women make up only 18% of digital technology roles, and the UK government’s Wise Campaign found that only 8% of women progress to a Level 4+ STEM qualification, and only 24% of women progress to the STEM workforce.
Increasing diversity in data science teams makes a lot of sense, not just because it casts the recruitment net wider, but also because it brings additional approaches, experiences and soft skills to the business. For any organisation that has decent in-house skills development, this makes perfect sense. Why look for a data geek who ticks all the boxes and is difficult to find when you can find people that tick some of the boxes and train them up?
Data literacy and in-house learning
Of course, it’s easier said than done. According to research conducted by automated analytics firm Alteryx, only 17% of UK data workers say they receive “the right kind” of data training at work. Interestingly, 33% of data scientists still spend an average of at least nine hours a week on basic data cleansing, blending and shaping, while 64% of employees say data training should be expanded to all data workers.
“The core challenge facing business today is twofold,” says Richard Timperlake, senior vice-president, EMEA, at Alteryx. “Not only are businesses in the UK facing a general shortage of data scientists, but are also seeing the work of their existing teams sapped by day-to-day data work that should not be their responsibility. It’s just not an efficient use of time for a master mechanic to perform an oil change.”
Timperlake’s point is that a lot of businesses are getting it wrong on in-house training and managing existing data skills resources. Demand for workers with specialist data skills in the UK has more than tripled over five years, according to the Royal Society, but businesses cannot rely exclusively on small teams of data specialists to do all the work. Instead, they need to boost the skills and training of every employee across the business, so that more people can use data to deliver business value.
“Unsurprisingly, the data scientists we surveyed strongly support upskilling other members of staff to take some of the strain,” says Timperlake. “While many say that training is currently only available to data scientists, 64% of the data scientists surveyed say training should be expanded to all data workers.”
That earlier point about “the right kind of training” is crucial, of course. There is no one-size-fits-all course here. Each business will have its own challenges and requirements. David Collins, managing director at capital markets consulting firm First Derivative, suggests that data literacy is relative but it is also important for businesses to engage with education, so that education understands what businesses need.
Read more about the design of data science teams
- How to structure and manage a data science team.
- What is data science? The ultimate guide.
- Data science teams use business ties to boost data knowledge.
“Data literacy alone does not make an individual a good data scientist in the business world – it is also key to have good knowledge on client sectors,” says Collins. “This is the belief driving our partnerships with Ulster University Business School and the University of Limerick. We are the co-creators of an MSc programme with the Business School that fits the financial and associated professional services skills needs of First Derivative.”
But what if this isn’t possible or employers lack resources and capability to train in-house? According to Harvey Nash’s James, it’s not unusual. Many organisations lack either the time or knowhow to deliver the skills that data teams need to thrive. Part of the problem is the ever-changing data landscape and demands placed on data teams to justify their existence to management. In the ongoing fight to attract and retain data scientists, this is key.
“For data scientists, we have found that learning and development is a high priority when choosing the right business to work for,” says James. “However, an often-overlooked area is the need to develop an understanding of business itself. This can make the difference between a ‘good’ or ‘great’ data scientist.”
To that end, James says he has seen an increase in self-development – more data scientists teaching themselves new tools to stay on top of latest technologies, but also markets and how the business works and competes in those markets. This, he says, is key, not just for the effectiveness of a data team, but also for the value of a data scientist to the overall business function. It brings job clarity and relevance, and with it, job satisfaction.
Motivation and morale
This comes into motivation. Keeping a team motivated is key to retention, but also attraction. As James says, it’s a seller’s market.
“If they don’t feel they are part of the journey and are not fairly rewarded for their efforts, they will leave,” he says. “It’s also well known that data science projects can be experimental and often fail or don’t go into production. This can lead to frustration and unrest in a team, causing a high turnover of staff.”
Barry Carter, CTO at contact centre tech firm MaxContact, agrees, pointing out that understanding their business’ priorities and how these relate to their own workloads is key for data scientists. Teams should be able to see the business impact of what they are doing in order to help keep motivation high.
“Ensuring that the scope of work is understood, and processes documented and automated where possible, is vital,” says Carter. “For repetitive tasks, document your approach and work within a set framework which allows you to ensure speed and quality. People-focused processes can really help in tackling burnout. Rotating individuals between different work types, for example, major projects and fixing bugs, allows them a break.
“Building in reflection periods to work cycles or sprints is invaluable in giving people the flexibility to address process issues. This prevents repetitive problems demoralising the team and harming the business. Teams should also have control over their projects, with the scope to investigate and realign internal expectations based on their findings.”
It is also key that managers identify any bottlenecks in complicated protocols and share knowledge to remove them. Fixing major issues together and documenting the fixes goes a long way to building morale and confidence.
Emphasis on teams
This emphasis on teams is increasingly crucial. As Felipe Henao Brand, senior product manager at data software company Talend suggests, the trend of centralising data teams has put even greater emphasis on business understanding. Data teams need to know the business, and need to communicate and be relevant.
“With this centralised approach, the management of data and its lifecycle and value becomes a collective project with the chief data officer as the leader,” says Henao Brand. “From an influential and transverse role with little power, this person becomes a decision-maker.
“Chief data officers will surround themselves with data scientists, data engineers and more and more business analysts, if necessary. The latter will play a key role because they are the missing link between data theory and its practical business application, as the business analyst will understand the business expectations linked to data. They will provide the missing key to unlocking the value of data.”
Keeping these teams together will be a constant challenge, and with little sign that the shortage of data scientists is being addressed, that challenge will continue for years to come. A good salary and in-house skills development will go a long way to attracting talent, but keeping teams engaged and relevant is a different matter altogether.