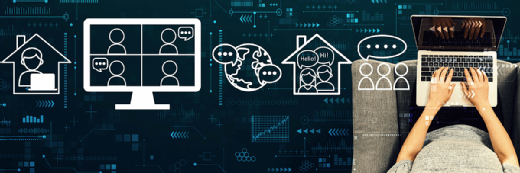
Four emerging data integration trends to assess
To accelerate their performance in data integration, companies are evaluating and adopting a range of contributing technologies
Data integration is increasingly critical to companies’ ability to win, serve and retain their customers. Enterprises face increasing data integration challenges, primarily due to the growing volume of data, compliance pressure, need for real-time information, increased data complexity, and data distribution across hybrid and multiple clouds. Business users want quick access to reliable, real-time information to help them make better business decisions.
A modern data integration strategy is critical to support the new generation of data and analytics requirements, including support for real-time customer 360, data intelligence, and modern edge applications. Enterprise architecture, technical architecture and delivery leaders should look at leveraging new approaches in data integration – such as data virtualisation, data mesh, artificial intelligence-enabled data integration, and data fabric – to make data and analytics even more effective.
Modern data integration technologies focus on advanced automation, connected data intelligence and persona-based interactive tooling, helping organisations to accelerate various use cases and other data integration requirements.
Distributed hybrid and multicloud data is creating new integration challenges. Data lives everywhere, so centralising it into data lakes or data hubs to support business insights is no longer practical, especially with the explosion of data at the edge. Forrester expects the adoption of data integration systems to proliferate in the coming years as organisations look for supporting insights across multicloud, hybrid cloud and edge environments.
Artificial intelligence (AI) is driving the next level of data integration solutions. New and innovative AI features are helping enterprises to automate data integration functions, including data ingestion, classification, processing, security and transformation. Although AI capabilities within data integration are still emerging, areas that technology architecture and delivery leaders can leverage today include the ability to discover connected data, classify and categorise sensitive data, identify duplicates and orchestrate silos.
Real-time data integration has become critical to support modern requirements. As the pace of business accelerates, real-time insights become critical, requiring enterprises to focus on platforms that can deliver analytics quickly. Enterprises often cite real-time and near-real-time data support as a top data integration requirement, primarily to support modern customer experience initiatives.
Customer demand is shifting to use-case-driven data integration solutions. This new and emerging category delivers optimised and comprehensive end-to-end data integration by automating the process of ingestion, integration, security and transformation for new and emerging business use cases, such as customer 360 and internet of things (IoT) analytics.
In mapping the future of the technologies in the data integration ecosystem, Forrester identified data as a service, data mesh, knowledge graph and query accelerator as four technologies that fall into the “experiment” category. They are regarded as having low maturity and low business value. Most enterprises should limit their exposure to these technologies to bounded experiments, waiting for the expected business value of these newer categories to improve before investing.
Data as a service
Data as a service (DaaS) – also known as data as a product (DaaP) – delivers a common data access layer through application programming interfaces (APIs), SQL, ODBC/JDBC and other protocols, leveraging data platforms such as data virtualisation, data mesh, integration platform as a service (iPaaS) and others. These are part of the new generation of advanced data integration technology that focuses on a common data access layer to accelerate various use cases.
DaaS/DaaP delivers a data access layer to support querying, reporting, data access, and integrated and custom-built applications. It offers several business benefits, including supporting a common view of business and customer data using industry-standard protocols.
Forrester expects DaaS/DaaP to experience continued growth in the coming years as demand for trusted and real-time data grows across applications. We are likely to see further innovation in real-time updates, integration and self-service capabilities.
Data mesh
A data mesh offers the ability to optimise mixed workloads by matching processing engines and data flows with the right use cases. It interfaces to the event-driven architecture, enabling support for edge use cases.
A data mesh offers an architecture that enables a communications plane between applications, machines and people. It matches the data, queries and models to the solution to keep each party – human and machine – in sync and speaking the same language.
It enables developers, data engineers and architects to become more productive and accelerate various business use cases.
Data mesh technology is still in its infancy. A data mesh leverages service mesh for data, a publish/subscribe (pub/sub) model for the edge, and onboard and local storage and commute to support a cloud-native architecture. We are likely to see data mesh evolve into a platform in the long term.
Knowledge graph
A knowledge graph makes use of graph engines to support complex data connections and integration. It helps build recommendation engines, cleanse data, perform predictive analytics and connect data quickly. Developers, data engineers and data architects can rapidly work through messy, unrelated data to accelerate application development and new business insights.
It leverages a graph data model to store, process and integrate connected data, building a knowledge base to answer complex questions and modern insights.
A knowledge graph accelerates analytics and insights that need connected data for applications, insights or analytics. It also improves the productivity of developers, data engineers, architects and data analysts.
As a data integration technology, knowledge graphs are still evolving with support for automation, built-in AI/machine learning and self-service capabilities. A knowledge graph leverages graph model, data catalogue and domain-specific ontologies to deliver a knowledge base.
Query accelerator
The query accelerator market has gained some traction to help developers and data engineers optimise queries quickly and move compute closer to data, thus minimising data movement. This technology is helpful when you have data stored in data lakes, object stores or complex data warehouses where tuning queries are not often straightforward.
Unlike data virtualisation systems, query accelerators speed up queries through an improved query optimiser, moving compute closer to data and fetching only selected data from data sources such as distributed databases, data warehouses, data lakes, object stores and files.
A query accelerator helps businesses to accelerate analytics and data searches through a simplified query that can be run by business analysts, business users and IT organisations.
Forrester expects they will evolve further in the coming years, with improved AI/machine learning and data intelligence being built into query accelerator products, combined with higher query performance and scale with less compute resources and more automated integration of distributed data.
This is an excerpt from “The Forrester tech tide: enterprise data integration, Q4 2021”. Noel Yuhanna is a principal analyst and vice-president at Forrester.
Read more about data integration
- Business process improvement is a never-ending quest for betterment that can be lit up by a new generation of process mining software. We take a deep dive.
- Continuous real-time analytics, operating at sub-second speed, is finding real-world applicability across industry sectors, such as retail, transport and banking. Their value crux: can businesses act on the signals provided?