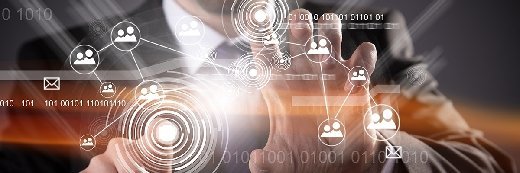
Kesu - Fotolia
Accurate data in, better insights out
The coronavirus pandemic has propelled data into the headlines, but it has also shown the challenges of dealing with incomplete datasets
In Covid-19 coronavirus daily news briefings, the epidemiology “R” reproduction value is regularly plucked out as a metric policy-makers use to show the general public the infection rate of the virus. The mathematical model behind the R value has driven policy decisions during the crisis, such as when to impose the lockdown, and when and how to loosen restrictions.
The importance of accurate data during crisis management was highlighted in a global crisis survey by PwC in 2019, which found that three-quarters of those in a better place following a crisis strongly recognised the importance of establishing facts accurately during a crisis.
According to PwC, it is essential that the crisis plan outlines how information will flow and that everyone has confidence in its veracity. “Strong data also reinforces a central element of crisis planning – exploring different scenarios and how they could affect the business in the short, medium and long term,” PwC partners Melanie Butler and Suwei Jiang wrote in February.
Behind the R value for coronavirus is the raw data the government uses to predict the impact of policy decisions. But data models are only as good as the raw data on which they build their assumptions and the quality of the data that is fed into these models. Data models that use machine learning to improve their predictive power can exacerbate problems caused when the assumptions made in data models are not quite right.
Read more about data quality
Statistics on data quality are the most common metrics used to track data governance programs, but other types of data-related measurements also can be applied.
Information management consultants talk about the importance of aligning business and IT drivers for a holistic architectural approach to ensuring strong data quality.
For instance, the Fragile Families Challenge – a mass study led by researchers at Princeton University in a collaboration with researchers across a number of institutions, including Virginia Tech – recently reported that the machine learning techniques scientists use to predict outcomes from large datasets may fall short when it comes to projecting the outcomes of people’s lives.
Brian Goode, a research scientist from Virginia Tech’s Fralin Life Sciences Institute, was one of the data and social scientists involved in the Fragile Families Challenge.
“It’s one effort to try to capture the complexities and intricacies that compose the fabric of a human life in data and models. But it is compulsory to take the next step and contextualise models in terms of how they are going to be applied in order to better reason about expected uncertainties and limitations of a prediction,” he says.
“That's a very difficult problem to grapple with, and I think the Fragile Families Challenge shows that we need more research support in this area, particularly as machine learning has a greater impact on our everyday lives.”
But even if the dataset is not complete, it can still be used to enable policy-makers to build a strategy. Harvinder Atwal, author of Practical DataOps and chief data officer (CDO) at Moneysupermarket Group, says models of forecasting Covid-19 can show the impact of policy changes.
For instance, he says the infection rate can be tracked to tell governments if their approach is working or not.
However, one of the challenges Atwal points to is the limited dataset. “You can create rough forecasting models, but the margin for error is quite high. Even so, looking at insights to drive policy decisions is fine,” he says.
For instance, while it has become apparent that the temporary Nightingale hospital at Excel was not required, the models used by the Department of Health and the government pointed to the coronavirus overloading the NHS and, as such, the need for additional intensive care beds. Even if the margin for error is quite high, the data model enables policy-makers to err on the side of caution, and prepare for a worst-case scenario.
Sharing data for better insights
Collaboration helps to improve the accuracy of data insights. “If you have lots of models, you can use the wisdom of crowds to come up with better models,” says Atwal. “Better insights arise when there are lots of opinions. This is particularly relevant with coronavirus predictions as the impact of the virus is non-linear, which means the economic and social impact become exponential.”
Data company Starschema has developed an open platform for sharing coronavirus data, based on a cloud-based data warehouse. Built on the Tableau platform and Snowflake, it includes datasets enriched with relevant information such as population densities and geolocation data.
Tamas Foldi, chief technology officer (CTO) at Starschema, says it aims to ensure everyone can get the cleanest possible source of data, the idea being to provide the data in a way that enables everyone to contribute to and comment about the data and use GitHub to request features, such as adding another dataset.
“After the pandemic, we will have enough data on how people reacted to policy changes,” he says. “It will be a really good dataset to study how people, government and the virus correlate.”
Getting quality data at the start
Data also needs to be of the highest quality, otherwise the data model may lead to invalid insights.
Andy Cotgreave, technical evangelism director at Tableau, recommends that organisations put processes in place to ensure data quality as it is ingested from source systems.
“Ensure data is checked for quality as close to the source as possible,” he says. “The more accurate it is upstream, the less correction will be needed at the time of analysis – at which point the corrections are time-consuming and fragile. You should ensure data quality is consistent all the way through to consumption.”
This means carrying out ongoing reviews of existing upstream data quality checks.
“By establishing a process to report data quality issues to the IT team or data steward, the data quality will become an integral part of building trust and confidence in the data. Ensure users are the ones who advise on data quality,” says Cotgreave.
“When you clean data, you often have to find inaccurate data values that represent real-world entities like country or airport names. This can be a tedious and error-prone process as you validate data values manually or bring in expected values from other data sources,” he adds. “There are now tools that validate the data values and automatically identify invalid values for you to clean your data.”
Gartner’s Magic quadrant for data integration tools, published in August 2019, discusses how data integration tools will require information governance capabilities to work alongside data quality, profiling and mining tools.
In particular, the analyst firm says IT buyers need to assess how data integrations tools work with related capabilities to improve data quality over time. These related capabilities include data profiling tools for profiling and monitoring the conditions of data quality, data mining tools for relationship discovery, data quality tools that support data quality improvements and in-line scoring and evaluation of data moving through the processes.
Gartner also sees the need for greater levels of metadata analysis.
“Organisations now need their data integration tools to provide continuous access, analysis and feedback on metadata parameters such as frequency of access, data lineage, performance optimisation, context and data quality (based on feedback from supporting data quality/data governance/information stewardship solutions). As far as architects and solution designers are concerned, this feedback is long overdue,” Gartner analysts Ehtisham Zaidi, Eric Thoo and Nick Heudecker wrote in the report.
Build quality into a data pipeline
A new area of data science that Moneysupermarket’s Atwal is focusing on is DataOps. “With DataOps you can update any model you build, and have a process to bring in new data, test it and monitor it automatically,” he says.
This has the potential to refine data models on a continuous basis, in a similar way to how the agile methodology improves software being developed based on feedback.
Atwal describes DataOps as a set of practices and principles to create outcomes from data, by having a production pipeline that moves through various stages from raw data to a data product. The idea behind DataOps is to ensure the process of data through the pipeline is both streamlined and results in a very high-quality data output.
One of the adages of computer science is “garbage in, garbage out”. In effect, if the data fed into a data model is poor, the insights it produces will be inaccurate. Assumptions based on incomplete data clearly do not tell the whole story.
As the Fragile Families Challenge found, trying to use machine learning to build models of population behaviour is prone to errors, due to the complexities of human life not being fully captured within data models.
However, as the data scientists working on coronavirus datasets have demonstrated, even partial, incomplete datasets can make a huge difference and save lives during a health crisis.
Broadening collaboration across different groups of researchers and data scientists helps to improve the accuracy of the insights produced from data models, and a feedback loop, as in DataOps, ensures that this feedback is used to improve them continuously.