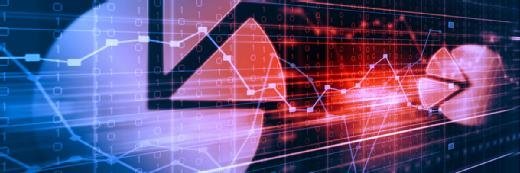
Sergey Nivens - Fotolia
Combine data mining and simulation to maximise process improvement
Data mining and computer simulation can be used together to better model and improve industrial processes, city development and other complex systems
We are living in an increasingly interconnected world – not just in the number of digital devices, but also in how actions can have dozens, if not hundreds or more consequences that cascade through a series of permutations, resulting in unforeseen consequences. But using a computer model of these can predict probable consequences.
Data mining has become something of a buzzword in recent years. It is also a misnomer and the term has been increasingly misused. Alan Mason, CEO of data mining firm AJM Consulting, prefers to use the term “process diagnostics”, as he believes it is more accurate for what the technique entails.
Rather than extracting data, as the term implies, data mining is the process of analysing large datasets and understanding their patterns. An understanding of past trends can allow insight when making decisions about current situations.
Data mining is often applied in process industries – chemical, pharmaceutical, nuclear, etc – where a small change in the beginning of a process can, through a series of interconnected events, have major consequences that were initially unforeseen.
An example application is AJM Consulting’s work with the Sellafield reprocessing and decommissioning site. AJM used process diagnostics to study the real-time data and operational history of the cooling process for the Waste Vitrification Plant, where the highly radioactive, toxic and corrosive waste is entrapped in corrosion-resistant borosilicate glass for long-term storage. Through this study, AJM identified specific events on the plant that accelerated the rate of corrosion of the cooling coils.
Taking this one step further, AJM could confidently predict when each cooling coil would fail. The Waste Vitrification Plant could withstand a certain number of cooling coils failing, but to have all of them fail would be catastrophic. Thus, AJM could mitigate the risk of unacceptable cooling coil failures, as well as save money for their customer by minimising corrosive processes and avoiding plant shut-downs.
Read more about data mining
- Data mining is sorting through data to identify patterns and establish relationships.
- Get these examples of how data mining is used in vertical industries – such as retail, manufacturing, healthcare, financial and telecommunications.
- Book author David Nettleton offers advice on avoiding data mining pitfalls and pinpointing valuable business data to mine and analyse.
Data mining and simulation
“It was sort of a simulation, but using process diagnostics at its heart,” explains Mason. “It has been found to give a better predictive warning system than had previously existed.”
For this, AJM Consulting used its own commercially available data-mining software called MS2. This program was developed through a European grant by AJM, in conjunction with Newcastle University, and has been applied to various process-type industries for over fifteen years.
Simulation, on the other hand, allows for the analysis of statistically probable events. Through studying the data and extrapolating the relationship between each variable, companies can model not only what is happening now, but also possible future scenarios.
At first glance, data mining and simulation might appear to offer the same results using similar techniques – but they are subtly different. Mining data determines the historic patterns of what has happened before, and extrapolates the most likely result of what will happen in the future. Conversely, simulations determine the most likely outcomes based on the relationship between the different variables extrapolated from the data.
Data use in civil engineering
It is true that both data mining and simulation require datasets to create an accurate model. The greater the amount and accuracy of the data, the better the model. However, the data scientist Leonardo Reyes of Profusion argues that, for simulation: “If you understand how the model really works – if you have been able to recognise the relationships for the model – then all you need is just that information.”
To attain that understanding, ideally data mining needs to be performed to identify the relationships between the different variables. In this regard, Reyes argues:“Data mining is almost like the previous step to really understanding how this data is working.”
Simulations can predict what will probably happen. It is for this reason they are commonly found in engineering, where proposed designs are modelled to ensure they provide an appropriate solution to meet the required safety and/or reliability targets. Simulations can also help to prevent unforeseen consequences.
Modelling urban design
One example is the design software Micro Drainage, which is used in civil engineering to simulate rainfall events in existing and proposed underground drainage networks. Micro Drainage models the fluid dynamics of water, using an established set of rules to ensure the design meets national standards. The program allows users to model extreme rainfall events to determine whether drainage systems can cope with excess rainfall.
On a much larger scale, cities can be modelled for studying urban design proposals.
Cities have rates of immigration and emigration, as well as people being born and dying. Through understanding the functions of the city and the needs of the city – such as energy and food consumption and housing requirements – all of these diverse elements can be linked together to provide optimal services for the population as it is now, as well as to plan for the population in the future, using different scenarios.
“If you are able to understand how your city works and what resources it needs and how it comes together, you can use the model to make decisions for people in the future,” explains Reyes. The ability to determine the possible consequences of conceptual scenarios is the key strength of the simulation.
Through simulating the existing infrastructure layout and comparing results with what is happening in reality, simulations can examine possible outcomes when new designs are proposed. In one such instance, city planners can map the probable changes in traffic flow caused by proposed city developments, allowing them the opportunity to mitigate possible congestion before it becomes a problem.
Data mining and simulation use
Data mining and simulation both have their own advantages and situations for which they are most appropriate.
Data mining can be used on real-word, historical data. “For some problems this is enough,” explains Herman Narula, CEO of Improbable.
“However, for complex systems, or situations without precedent where you have no data, it may be difficult to make meaningful predictions by extrapolating past trends.” In these circumstances, simulation is the answer. However, simulations are only as good as the understanding of the relationships between the variables within the system, and often this can only be accrued once data mining has been performed, the patterns identified and model verified by simulating current events and comparing results with what is happening in reality.
As well as having sufficient data, data scientists also need to ensure the data is accurate. “You really need great input to have really good output,” says Reyes.
Accurate data mining
It is also important to validate the findings with what happens in reality to ensure that the model is a true representation. “The strength of data mining – the fact that you can ‘blindly’ look for patterns in data – is also its major weakness,” says Enrico Scalas, head of department and professor of statistics and probability at the University of Sussex. “Correlations could be there for a common cause, or because one of the variables is the cause of the other – but they could be completely spurious.”
Depending on the duration of the model, pattern-finding may need to be conducted multiple times. “Validation and model improvement should be seen as an ongoing process, for any long-running simulation initiative,” explains Narula. “It is the responsibility of the simulation expert to ensure that any uncertainty is treated in a rational way.”
Data mining can be performed on a conventional desktop computer. Obviously, the greater the datasets, the more computing power will be required – especially when parallel processing is used. There are tools available that can help with parallelisation and distribution, which allows for data mining to be undertaken faster.
Simulation distributed across machines
For simulations, however, the visualisation requirements can require more computing power. In the past it has been impossible to distribute simulations, but Improbable have been developing a system for instances when elements can be defined as an entity in space. “SpatialOS automatically distributes the code across hundreds, or even thousands, of machines, enabling developers to build simulated worlds of a size and level of detail previously impossible,” says Narula.
Data mining and simulation have often been considered as in competition with each other, but the reality is that they are complementary. Each informs the other and produces more accurate results. Appropriate data mining gains an understanding of the relationships in a system. Simulations can then be modelled to plot hypothetical scenarios, the results of which can enable further analysis. However, care should be taken to ensure that modelling iterations do not amplify any errors.
With computing power increasing and Data retention becoming ever more ubiquitous, we become able to model far larger and more complex systems, allowing the possible applications for data mining and simulation to expand. These systems will then be able to further detect previously unforeseen consequences, allowing for the mitigation of detrimental consequences before they materialise.