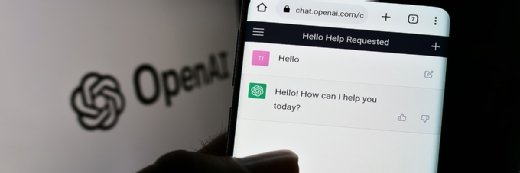
Timon - stock.adobe.com
Gartner: Exploring the short- and mid-term implications of ChatGPT
ChatGPT and competitors such as Google’s Bard mark a significant shift in the market for data and analytics
Large language models (LLM) have the potential to transform the approach to data analysis and decision-making. As such, it's crucial to understand the short-term and mid-term implications of this new development.
As a cutting-edge application that leverages a large language model, ChatGPT is revolutionising the thinking about data and analytics. Its advanced capabilities in natural language processing and machine learning (ML) have already made it a valuable tool for various industries, from finance and healthcare to education and entertainment.
ChatGPT’s evolution is far from over. In the coming year, it’s expected that there will be further rapid progress in the development of ChatGPT and similar solutions, as well as the emergence of complementary technologies.
Limitations and risks
As an application built on the GPT large language model, ChatGPT is well-suited for language-related tasks. However, its ability to perform mathematical operations is more limited. It is important to note that ChatGPT has limitations and is not suitable for many analytics tasks.
Many use cases for ChatGPT in data and analytics will be to aid data engineers, data analysts and data scientists with tasks that involve programming systems and code generation. For example, a data engineer could ask a language model to generate data ingestion and transformation scripts, configuration templates, and SQL queries. A data analyst could use it to assist in generating DAX code for PowerBI, while a data scientist could use it to assist in reviewing Python code for machine-learning-related functions.
The above usage, however, risks the exposure of restricted or sensitive information being passed to the company that builds the model. As a result, data and analytics practitioners should ensure that any confidential or proprietary material is properly handled by the LLM service provider. For ChatGPT that would be OpenAI or Microsoft Azure OpenAI Services. Another risk is that generated code or other output may be unreliable, therefore the use of these tools to augment the developer’s processes is the recommended approach.
Data and analytics practitioners should take the lead in informing the risk and compliance policies related to using generative AI tools and act as subject matter experts when educating business stakeholders. Practitioners should apply the generated AI code in phases and highlight and monitor it against standard code quality controls or be subjected to the same review and testing as human-written code.
Policies to guide responsible use of proprietary content
To ensure safe and appropriate use of ChatGPT look to establish policies for its use guided by the data and analytics delivery team. This includes a close review of generated code by the developers and marking generated code as such and subjecting it to the same testing procedures as normal code. By doing so, organisations can ensure that ChatGPT is used responsibly and that any output is reliable.
In the coming years, a more proactive approach will be required to use generative AI tools like ChatGPT. This involves participating in and leading initiatives to understand the impact of generative AI on business and society as a whole. Through data literacy programmes, every employee should be educated on how generative AI systems work, their limitations, and the appropriate scope of use for their role. They should also be encouraged to think about use cases and ways this can be used to assist them by augmenting some of their tasks and by automating others.
To ensure the safe and effective use of ChatGPT and competitors, it is also essential to establish specific policies around their use. This includes seeking guidance from legal leaders to ensure compliance with regulations and laws.
Policies should ensure that there are always human-in-the-loop processes to check for errors and ensure that generated code or output is accurate and reliable. By taking these steps, organisations can maximise the potential benefits of generative AI tools while minimising the risks associated with their use.
Bern Elliot is a distinguished VP analyst at Gartner. The Gartner Data & Analytics Summit is taking place 22-24 May 2023 in London.
Read mre about Large Language Models
In a guest blogpost, Neo4j’s Alyson Welch explains why Large Language Model AI systems can’t move beyond non-trivial applications until they are properly curated.
Large language models carry significant risk for enterprises. For JPMorgan Chase, mitigating the risk means creating a learning environment and monitoring the use of the models.