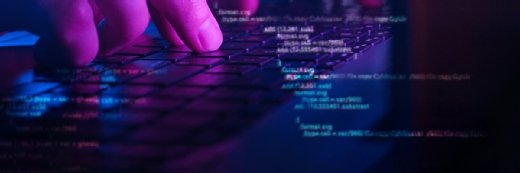
Getty Images/iStockphoto
Five tips to help you deploy a successful AI initiative
Former H&M head of AI, Errol Koolmeister, discusses an approach to delivering successful artificial intelligence projects
I have been working on artificial intelligence (AI) for large enterprises over the last 15 years, from setting up the Vodafone AI team, which is now powered by 500 people, to the past three years when I was head of AI foundation for the global fashion retailer H&M Group.
During my time at H&M, I was in charge of deploying the AI strategy. The technology is now used for forecasting, buying, spotting trends, improving the customer experience and helping the company to achieve a climate-positive value chain.
Although every business is different and will have different reasons for using AI, I have found that the recipes and blueprints to achieve a successful AI initiative can be used again and again. Often, I hear from enterprise clients that they do want to deploy AI in their operations, but often don’t know where to start.
They don’t know what they need and what are the outcomes they want to achieve, so they come to consultants like me. Here’s what I have learned over the years and what enterprises need to do when deploying an AI initiative to make it a success.
1. Stop focusing on algorithms
It can be easy to go from zero to 10 AI models by throwing money and people at the problem. But first, you need to step back and look at the strategy. Most companies don’t manage to do that because they don’t get the context right.
Before you do all this, you need to focus on having the right strategy and connecting it to your business strategy. How does AI fit into what you want to achieve as a company in the next six months to two years? You need to answer this question first by understanding how you can get the right people and talent in, focusing on the right ways of working or your operating model, technology and data, and then all the other things associated with it.
AI is as much about business transformation as it is algorithms, so start there first.
2. Bring stakeholders on board
Enterprises have lots of processes and data points associated with them that can be improved by utilising AI. But to improve every process, you need to have a clear path forward and roadmap, which starts with speaking to your stakeholders.
By getting them to agree what are the main processes that can utilise AI in the current context, value and quantify them, and combining that with an estimation of effort and how long it will take, means that you can decide which are the big use cases to use your specialised team for, and the smaller but still significant ones that your other teams could work on using a platform.
For instance, this could be deploying an AI model to improve customer service or investigating how AI could speed up product outcomes. By bringing together the business side and the development side, it will give you more of an idea of where value can be created and assign the appropriate teams to achieve it.
3. More people aren’t always the answer
Something I’m hearing time and time again is that the global skills shortage is hurting enterprises hard. It’s becoming more difficult and expensive to hire data scientists, whether you’re a tech company or not. But specialist staff aren’t necessary for every company, particularly when it comes to AI.
There has been a rise in recent years of AI-as-a-service platforms, such as Swedish-based platform Peltarion, which lower the barrier to entry and makes it easier for employees without a data science background to create and deploy AI models.
If you want to empower current staff to use AI, then it pays to arm them with the skills they need. Companies like iconic jeans brand Levi Strauss are launching internal upskilling programmes through in-house AI courses to allow employees to develop new skillsets.
Upskilling your current workforce to use their domain knowledge and apply it using an AI can bring valuable perspectives to the company and empower those who know what needs to be done to improve processes with the knowledge they need to implement them.
4. Test, test and test again
Companies can often be too focused on a certain use case, but that isn’t always the right strategy for implementing AI. Instead, what is the right strategy is to test, test and test again. The only thing you know with AI is you don’t know but you will learn on the way.
The use case for AI can be irrelevant, but what is relevant is the success of it, and to achieve this, you need to be constantly testing and adapting. After all, with AI, experimenting and having something “done” is always better than perfect.
Testing can have another benefit as well – in ironing and rooting out bias. One famous example was when e-commerce giant Amazon decided to attempt to automate its recruiting process back in 2014. After rigorous testing of the AI project, the company realised that the new AI recruiting system was not rating candidates fairly and showed bias against women.
Amazon was forced to ditch the algorithm for recruiting purposes and go back to the drawing board. Trying and failing is a key part of business and AI is no different – that’s why testing is so important.
5. Don’t be scared
Most people in enterprises are scared of AI – they understand they should be using it, but it can seem like a mountain they need to climb first. But once they realise they can combine their own skills with AI, they become more confident. Once they become more confident, then you can make the business case more realistic. It becomes more than just a technology; instead, it becomes a key asset for your business.
Errol Koolmeister is the former head of AI at H&M and AI consultant working with companies including Peltarion on how to make AI more accessible for enterprises
Read about AI in retail
- Prices for food, gas and more have risen during the past year. Revionics’ senior director of retail innovation discusses how retail AI tools can help companies navigate inflation.
- Google released AI products for online retailers as the tech giant increases its focus on industry verticals. The new products are designed for small and large retailers.