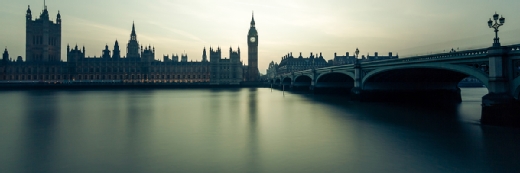
Daniel - stock.adobe.com
Data-driven government needs practical steps
We should build data platforms for government with the same techniques used in creating anything digital, argues Jim Stamp, head of data at Made Tech
Data-driven government is not new, or innovative – but it is essential to underpin policy and operational decision-making. Despite this having been central to government digital strategy for years, we are still struggling to see the outcomes, especially outside pockets of the pandemic response.
We know the problems – legacy technologies, a skills gap and cultural blockers – but what practical steps can we take today that will actually move the needle so that all public services are designed in a way that truly benefits citizens?
We talk a lot about user values in digital transformation, and working with data is no different. It is slightly astonishing how much money is put into building data platforms without applying the same techniques that we use when creating a digital system. For instance, if you were building a website, you would use user research to identify problems, test ideas and validate solutions, and only then deliver to those requirements.
Across the public sector, a lot of expensive data platforms are created with a “build it and they will come” mentality. This ignores what people really need, so the systems are not adopted and, as a result, those platforms are deemed failures.
Instead, we should build data platforms with the same techniques we use when creating anything digital. If you fix problems that people have, you make things easier for them, and a data platform will become sticky because there is a reason to use it.
Finding balance is critical for both the creation of a data platform but also the use of it. The data space moves fast, and it is worth remembering that what you are building will only last so long. This means you must balance this new-thing-versus-old-thing mentality. But, equally, you don’t want to just keep adding new tools to the toolkit. Iterate for as long as you need to really deliver value for the people you are designing for. Creating space for innovation is crucial – but don’t underestimate new tech fatigue.
Create a shared language
One significant blocker to the adoption of data-driven practices is a lack of a common language. It is too easy for one term to have numerous meanings within an organisation. Moving to a domain-driven, product view of data can help.
We have found domain-driven development to be a great starting point. It’s an idea that allows you to view your organisation as a set of bounded domains and identify the root of terms and their meaning. This allows you to create an organisational data model to clarify meanings and foster better conversations between teams.
Once you really understand your organisation in this way, you can start building a common vocabulary, where terms such as “person” and “property” have the same meaning (or at least an agreed one!) to all.
The reason that data platforms fail is rarely due to the technology – it’s often because of the culture behind its use. Even something as simple as ownership can cause issues. It is usually clear to teams that they are responsible for the data in the databases that they look after. What is less clear to them is that the data still belongs to them once it has been copied into a data platform.
Helping the teams to feel connected to the platform, because they use it to solve a problem, will give them a reason to care about their data once it’s in there. This extends to governance and legal issues, too – the data doesn’t stop being the team’s responsibility just because it has been copied.
There is a cultural aspect of making sure you train all your people to be data literate too.
Data literacy can take different shapes, but you are never going to become a data-mature organisation if you haven’t been through a cultural shift.
Architect your technology to be replaced
Creating technology to be replaced is hard to do in the digital and data space. There are foundational pieces that you should put in place that won’t change. But, equally, try to use open technology as much as you can.
There is a cost with open-source frameworks. They are free to use, but they can be expensive to maintain. But by using open technology, you can take your data and shift it from one system to another without having to rewrite everything.
The days of having a governance committee that reviews all things data feels opposite to what we have done within the digital sector.
Read more about data in government
- Data sharing: How can we make sure the UK is a world leader?
- NAO guide for senior government leaders flags barriers to better data use.
- UK government introduces data reforms legislation to Parliament.
We, as a community, should be agreeing on what principles we want to apply to our data. Agreeing on the definition of “sufficient testing” and finding ways to share/contract data schemas is far more efficient than a distant panel taking control. Shifting the responsibility to the teams creating the products is a huge step towards true data maturity.
You would never build an API [application programming interface] for your customers and then change the interface without warning them. With APIs, we use techniques like version numbers or upgrade paths to ensure continuity or service. Sadly, this isn’t always the case with data.
Often, we find data is collected from points within a system that are not really intended for consumption, so the data’s schema can be ill-considered or, even worse, change with no notice. By building data as a product, where it is intended and designed for use by others, we can prevent this issue.
This comes back to making sure the team that generates the data owns the data. They need to maintain and care about it, otherwise people won’t use it and it will cost the organisation time, money and effort.
Focus on the ethics of personal data
Having access to a person’s name or address often feels vital to completing a piece of analysis. In the vast majority of cases, it is not. It is only human to want to see names and postcodes that seem familiar to use, rather than a column of random numbers, but from a mathematical point of view, it very rarely makes any difference. In fact, we usually convert them to numbers to use the values.
Wherever possible, we should question when we see personal details, and even more so protected characteristics. As a default, we should not have access to them and we should pseudonymise the values.
As data platforms become more mature and people start using machine learning, ethics becomes more important. One of the only exceptions to the pseudonymisation rule is to make sure that any selected training data is representative of the population and has no bias in it. Even in this case, we should not be able to identify a person, but only know enough to assess the data for bias.
Data continues to be a hot topic, across both the private and public sectors. And although all the foundations mentioned come from a technology point of view, they are particularly applicable to the pockets of legacy-facing portals within our public services. If we want to realise the benefits of data-driven government, we need to get our foundations in place – and there is no better time to do that than now.
Jim Stamp is head of data at Made Tech