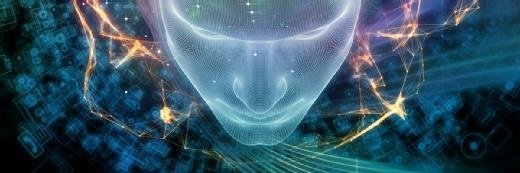
agsandrew - Fotolia
Aruba Atmosphere: Machine learning to transform networking
At Aruba Atmosphere EMEA in France, delegates learned how the network will incorporate machine learning features in the near future
Machine learning and artificial intelligence (AI) technology will have a huge impact on how networks are managed and run, and probably sooner than anybody thinks, according to speakers at mobile and wireless networking solutions supplier Aruba’s annual European customer and partner event, Atmosphere, which is currently underway at Disneyland Paris.
Citing his own figures from a November 2016 report, Thomas Meyer, group vice-president at IDC, said that by 2019, AI will be integrated into 40% of digital transformation and 100% of internet of things (IoT) initiatives, while in a separate keynote presentation, David Rowan, UK editor in chief at Wired, predicted AI would hit 100% of businesses eventually.
For Aruba machine learning will be instrumental in network management. In the past 15 years, network management has gone from a manual process to an automated one, and it is now going further; last year, the HPE-backed business bought networking firm Rasa to manage this evolution and start to introduce predictive network health assessments, benchmarking and recommendations via machine learning and correlations.
Partha Narasimhan, chief technology officer (CTO) at Aruba, told Computer Weekly the two businesses were drawn together because his customers consistently complained they were having problems with their Wi-Fi networks, only for Aruba to find it was actually a DNS server fault or a congested internet connection, for example.
Because Aruba was always the first stop for angry customers, Narasimhan started to ask if there was a way he could examine all the protocol exchanges going back and forwards, analyse this data, and infer the nature of a problem – or even predict one.
“Network management tools emphasise troubleshooting, the data that is collected is biased to the negative and the abnormal starts to look normal,” said Narasimhan. “We wanted to collect all the data possible so we have the good and the bad, and the bad stands out.”
“We started recording, but it became too big,” he said. “This is serious data science and not something we could just hack up. We needed data scientists to help us: Rasa wanted access to our data, and we wanted people to come and analyse it.”
Unreliable humans
It turned out that machine learning is actually ideal for network management and troubleshooting purposes because humans are very unreliable observers. Many will put up with a problem until it causes them substantial pain and then start complaining on Twitter, while others are terrible at identifying problems that don’t occur very frequently. They also have a tendency to get confused when presented with too much data.
“Machines have the opposite problem,” he said. “They’re no good if they don’t have a lot of data, and if you feed them data in a way that would overwhelm a human, they can find an issue the human wouldn’t.”
Besides troubleshooting and getting ahead of everyday faults, Aruba is also using Rasa to address network security issues – which are becoming more pronounced as IoT deployments gather pace in the enterprise – and identify possible problems, for example an IP security camera talking to something it shouldn’t be, which could indicate it has been recruited into a botnet.
“We are getting to a point now where the elements of running a network can be automated when expending human energy is not the best way of getting things done. We want to get to a point where we can be entirely data driven, but we have a long way to go,” said Narasinham.
Machine learning in real-world networks
At the European Organisation for Nuclear Research (Cern), machine learning will soon come into play to manage the vast amounts of data being produced by its various particle accelerators and, of course, the famed Large Hadron Collider (LHC).
Cern communications systems leader Tony Cass, who is in the process of deploying an Aruba Wi-Fi network across the organisation’s campus (although not in the LHC’s 28km long underground ring), said that the more data he had access to, the better he would be able to analyse it to provide the best service possible. Using machine learning was therefore an attractive idea.
“As the IT department, our role is to make the data we have easily available so that people can look at it in any way they want,” he said.
Cass referred to a recent scenario where a problem in one accelerator had knock-on effects in other accelerators down the line, and suggested machine learning would have been able to pick up on the missed warning signs that led up to the issue.
Read more about machine learning
- Unit4, which majors on business applications software for professional services firms and universities, adds machine learning to its product suite.
- The Royal Society believes the UK is well placed to make the most of machine learning, but it is early days, and technical and policy issues need addressing.
- Consultant David Loshin explains some widely used data analytics and machine learning techniques, and details how the associated automated algorithms work.
For network monitoring, he said, Cern had previously developed some applications with what was then plain old HP, to control a number of network monitoring functions which were being phased out as it moved onto Aruba’s hardware, and Cass said he anticipated the acquisition of Rasa would restore some of that functionality for Cern in time.
Back in the UK, the University of Cambridge has found itself in a similar scenario, needing to support the needs not just of students and researchers but the visiting public as well. Jon Holgate, head of networks at the institution’s University Information Services unit, said the use of machine learning and AI on the Cambridge network was “inevitable”.
“We’re not an internet service provider (ISP) but we do run a fairly large campus network. The volume of data and its complexity is already overwhelming, and we’re in desperate need of more analytical tools to be able to give us something slightly more nuanced,” said Holgate.
“We’re going to need help with this. We have one of the more complex types of network out there, with so many users and researchers wanting to do their own thing. We have to work in partnership with our suppliers and researchers.”